A Reinforcement Learning Algorithm for Data Collection in UAV-aided IoT Networks with Uncertain Time Windows.
ICC Workshops(2021)
摘要
Unmanned aerial vehicles (UAVs) have been considered as an efficient solution to collect data from ground sensor nodes in Internet-of-Things (IoT) networks due to their several advantages such as flexibility, quick deployment and maneuverability. Studies on this subject have been mainly focused on problems where limited UAV battery is introduced as a tight constraint that shortens the mission time in the models, which significantly undervalues the UAV potential. Moreover, the sensors in the network are typically assumed to have deterministic working times during which the data is uploaded. In this study, we revisit the UAV trajectory planning problem with a different approach and revise the battery constraint by allowing UAVs to swap their batteries at fixed stations and continue their data collection task, hence, the planning horizon can be extended. In particular, we develop a discrete time Markov process (DTMP) in which the UAV trajectory and battery swapping times are jointly determined to minimize the total data loss in the network, where the sensors have uncertain time windows for uploading. Due to the so-called curse-of-dimensionality, we propose a reinforcement learning (RL) algorithm in which the UAV is trained as an agent to explore the network. The computational study shows that our proposed algorithm outperforms two benchmark approaches and achieves significant reduction in data loss.
更多查看译文
关键词
UAV,internet-of-things,reinforcement learning,battery swapping,time windows,uncertainty
AI 理解论文
溯源树
样例
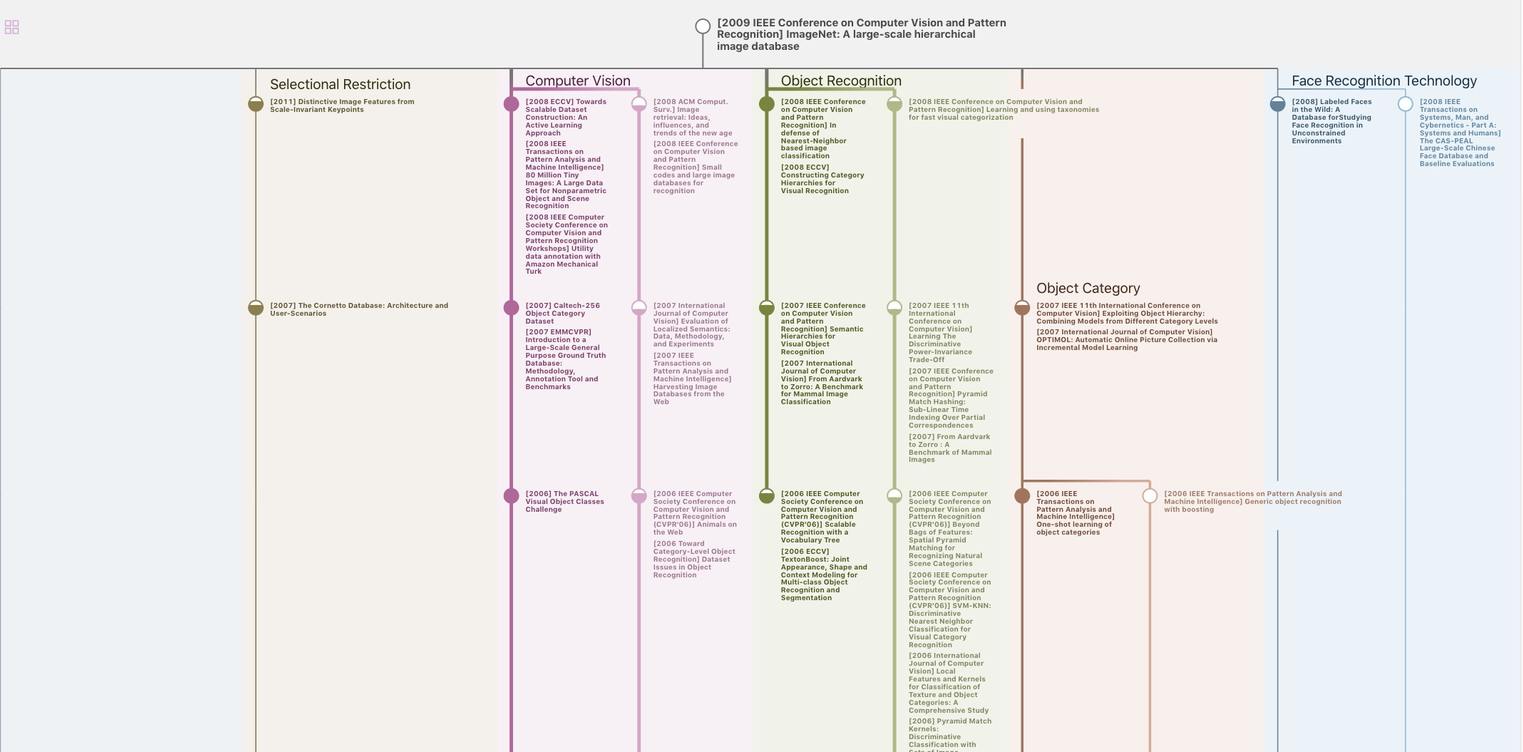
生成溯源树,研究论文发展脉络
Chat Paper
正在生成论文摘要