Poster: < SmartTE: Partially Deployed Segment Routing for Smart Traffic Engineering with Deep Reinforcement Learning >
2021 IFIP NETWORKING CONFERENCE AND WORKSHOPS (IFIP NETWORKING)(2021)
摘要
Segment Routing (SR) provides Traffic Engineering (TE) with the ability of explicit path control by steering traffic passing through specific SR routers along a desired path. However, large-scale migration from a legacy IP network to a full SR-enabled one requires prohibitive hardware replacement and software update. Therefore, network operators prefer to upgrade a subset of IP routers into SR routers during a transitional period. This paper proposes SmartTE to optimize TE performance in hybrid IP/SR networks where partially deployed SR routers coexist with legacy IP routers. We use two centrality criteria in graph theory to decide which IP routers should be upgraded into SR routers under a given upgrading ratio. SmartTE leverages Deep Reinforcement Learning (DRL) to infer the optimal traffic splitting ratio across multiple pre-defined paths between source-destination pairs. Extensive experimental results with real-world topologies show that SmartTE outperforms other baseline TE solutions in minimizing the maximum link utilization and achieves comparable performance as a full SR network by upgrading only 30% IP routers.
更多查看译文
关键词
Traffic Engineering, Segment Routing, Deep Reinforcement Learning
AI 理解论文
溯源树
样例
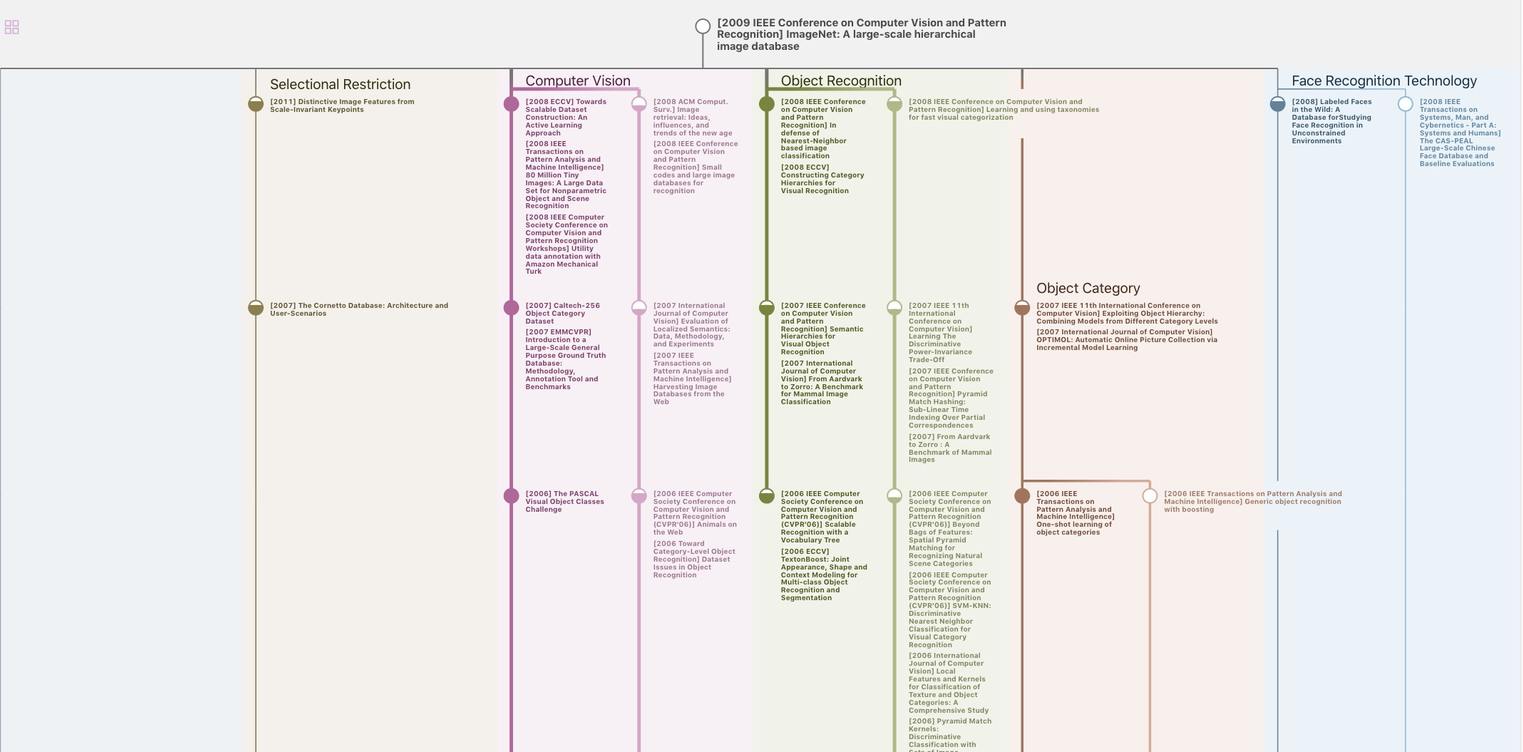
生成溯源树,研究论文发展脉络
Chat Paper
正在生成论文摘要