A comparison of statistical relational learning and graph neural networks for aggregate graph queries
MACHINE LEARNING(2021)
摘要
Statistical relational learning (SRL) and graph neural networks (GNNs) are two powerful approaches for learning and inference over graphs. Typically, they are evaluated in terms of simple metrics such as accuracy over individual node labels. Complex aggregate graph queries (AGQ) involving multiple nodes, edges, and labels are common in the graph mining community and are used to estimate important network properties such as social cohesion and influence. While graph mining algorithms support AGQs, they typically do not take into account uncertainty, or when they do, make simplifying assumptions and do not build full probabilistic models. In this paper, we examine the performance of SRL and GNNs on AGQs over graphs with partially observed node labels. We show that, not surprisingly, inferring the unobserved node labels as a first step and then evaluating the queries on the fully observed graph can lead to sub-optimal estimates, and that a better approach is to compute these queries as an expectation under the joint distribution. We propose a sampling framework to tractably compute the expected values of AGQs. Motivated by the analysis of subgroup cohesion in social networks, we propose a suite of AGQs that estimate the community structure in graphs. In our empirical evaluation, we show that by estimating these queries as an expectation, SRL-based approaches yield up to a 50-fold reduction in average error when compared to existing GNN-based approaches.
更多查看译文
关键词
Probabilistic soft logic,Markov logic networks,Graph neural networks,Aggregate graph queries,Citation networks,Graph convolutional networks,Graph attention networks,Graph markov neural networks
AI 理解论文
溯源树
样例
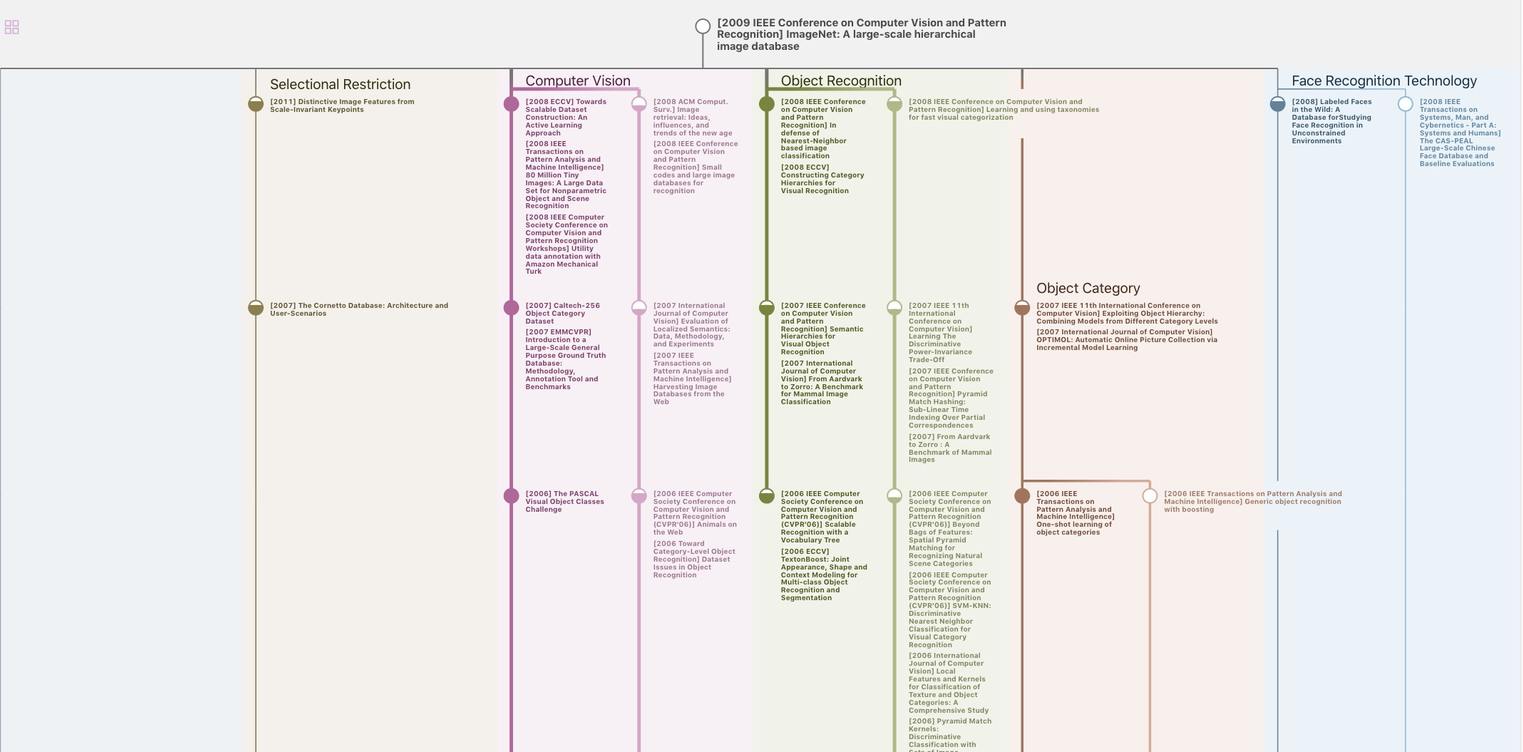
生成溯源树,研究论文发展脉络
Chat Paper
正在生成论文摘要