A Bayesian Compressive Sensing Approach to Robust Near-Field Antenna Characterization
IEEE TRANSACTIONS ON ANTENNAS AND PROPAGATION(2022)
摘要
A novel probabilistic sparsity-promoting method for robust near-field (NF) antenna characterization is proposed. It leverages on the measurements-by-design (MebD) paradigm, and it exploits some a priori information on the antenna under test (AUT) to generate an overcomplete representation basis. Accordingly, the problem at hand is reformulated in a compressive sensing (CS) framework as the retrieval of a maximally sparse distribution (with respect to the overcomplete basis) from a reduced set of measured data, and then, it is solved by means of a Bayesian strategy. Representative numerical results are presented to, also comparatively, assess the effectiveness of the proposed approach in reducing the "burden/cost" of the acquisition process and mitigate (possible) truncation errors when dealing with space-constrained probing systems.
更多查看译文
关键词
Noise measurement, Antenna measurements, Signal to noise ratio, Antennas, Uncertainty, Bayes methods, Probabilistic logic, Antenna measurements, antenna qualification, compressive sensing (CS), near-field (NF) pattern estimation, near-field to far-field (NF-FF) transformation, sparsity retrieval, truncation error
AI 理解论文
溯源树
样例
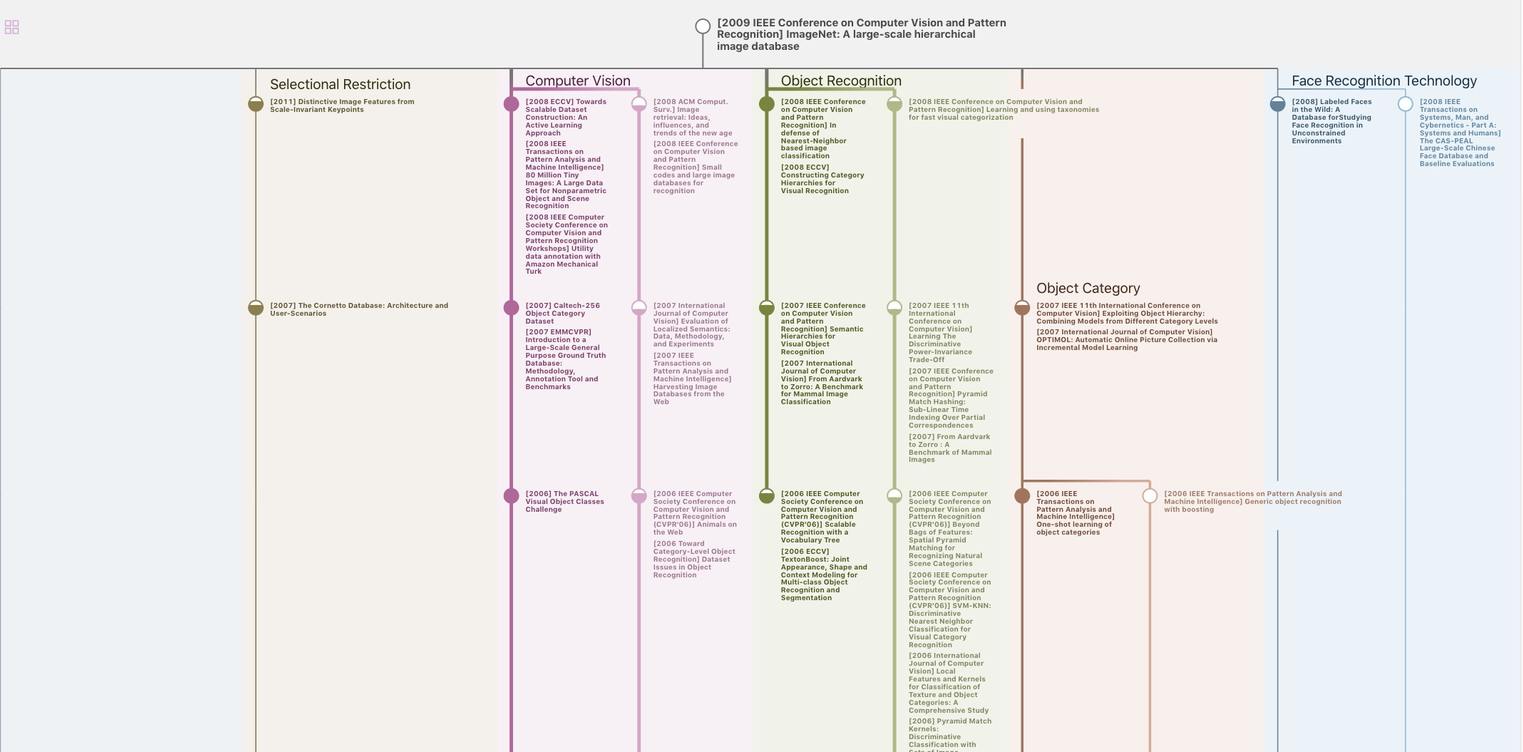
生成溯源树,研究论文发展脉络
Chat Paper
正在生成论文摘要