Detection Of Banana Plants Using Multi-Temporal Multispectral Uav Imagery
REMOTE SENSING(2021)
摘要
Unoccupied aerial vehicles (UAVs) have become increasingly commonplace in aiding planning and management decisions in agricultural and horticultural crop production. The ability of UAV-based sensing technologies to provide high spatial (<1 m) and temporal (on-demand) resolution data facilitates monitoring of individual plants over time and can provide essential information about health, yield, and growth in a timely and quantifiable manner. Such applications would be beneficial for cropped banana plants due to their distinctive growth characteristics. Limited studies have employed UAV data for mapping banana crops and to our knowledge only one other investigation features multi-temporal detection of banana crowns. The purpose of this study was to determine the suitability of multiple-date UAV-captured multi-spectral data for the automated detection of individual plants using convolutional neural network (CNN), template matching (TM), and local maximum filter (LMF) methods in a geographic object-based image analysis (GEOBIA) software framework coupled with basic classification refinement. The results indicate that CNN returns the highest plant detection accuracies, with the developed rule set and model providing greater transferability between dates (F-score ranging between 0.93 and 0.85) than TM (0.86-0.74) and LMF (0.86-0.73) approaches. The findings provide a foundation for UAV-based individual banana plant counting and crop monitoring, which may be used for precision agricultural applications to monitor health, estimate yield, and to inform on fertilizer, pesticide, and other input requirements for optimized farm management.
更多查看译文
关键词
unoccupied aerial vehicle, UAV, banana plant, geographic object-based image analysis, convolutional neural network, CNN, template matching, local maximum filter
AI 理解论文
溯源树
样例
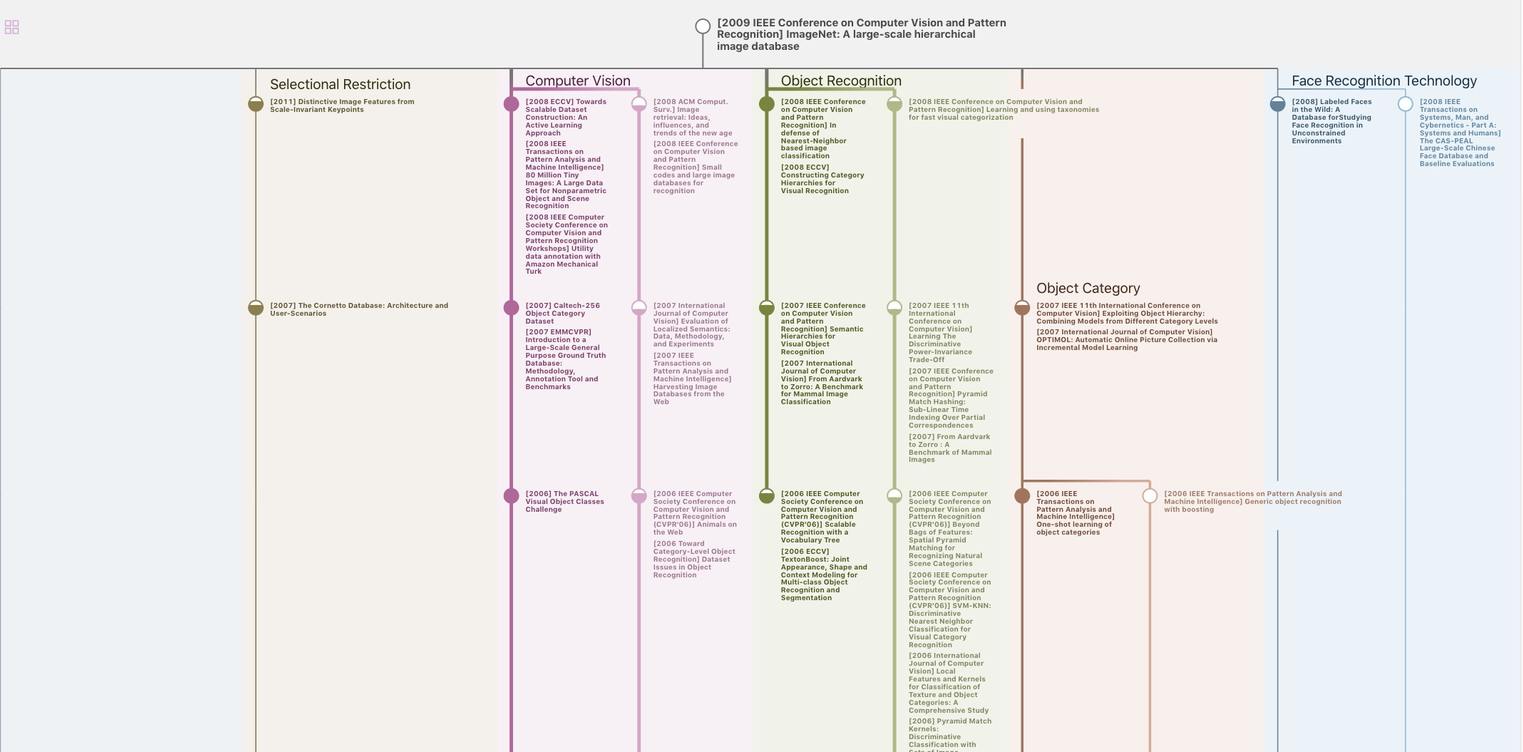
生成溯源树,研究论文发展脉络
Chat Paper
正在生成论文摘要