Hierarchical Analysis of Visual COVID-19 Features from Chest Radiographs
arxiv(2021)
摘要
Chest radiography has been a recommended procedure for patient triaging and resource management in intensive care units (ICUs) throughout the COVID-19 pandemic. The machine learning efforts to augment this workflow have been long challenged due to deficiencies in reporting, model evaluation, and failure mode analysis. To address some of those shortcomings, we model radiological features with a human-interpretable class hierarchy that aligns with the radiological decision process. Also, we propose the use of a data-driven error analysis methodology to uncover the blind spots of our model, providing further transparency on its clinical utility. For example, our experiments show that model failures highly correlate with ICU imaging conditions and with the inherent difficulty in distinguishing certain types of radiological features. Also, our hierarchical interpretation and analysis facilitates the comparison with respect to radiologists' findings and inter-variability, which in return helps us to better assess the clinical applicability of models.
更多查看译文
关键词
chest radiographs,visual
AI 理解论文
溯源树
样例
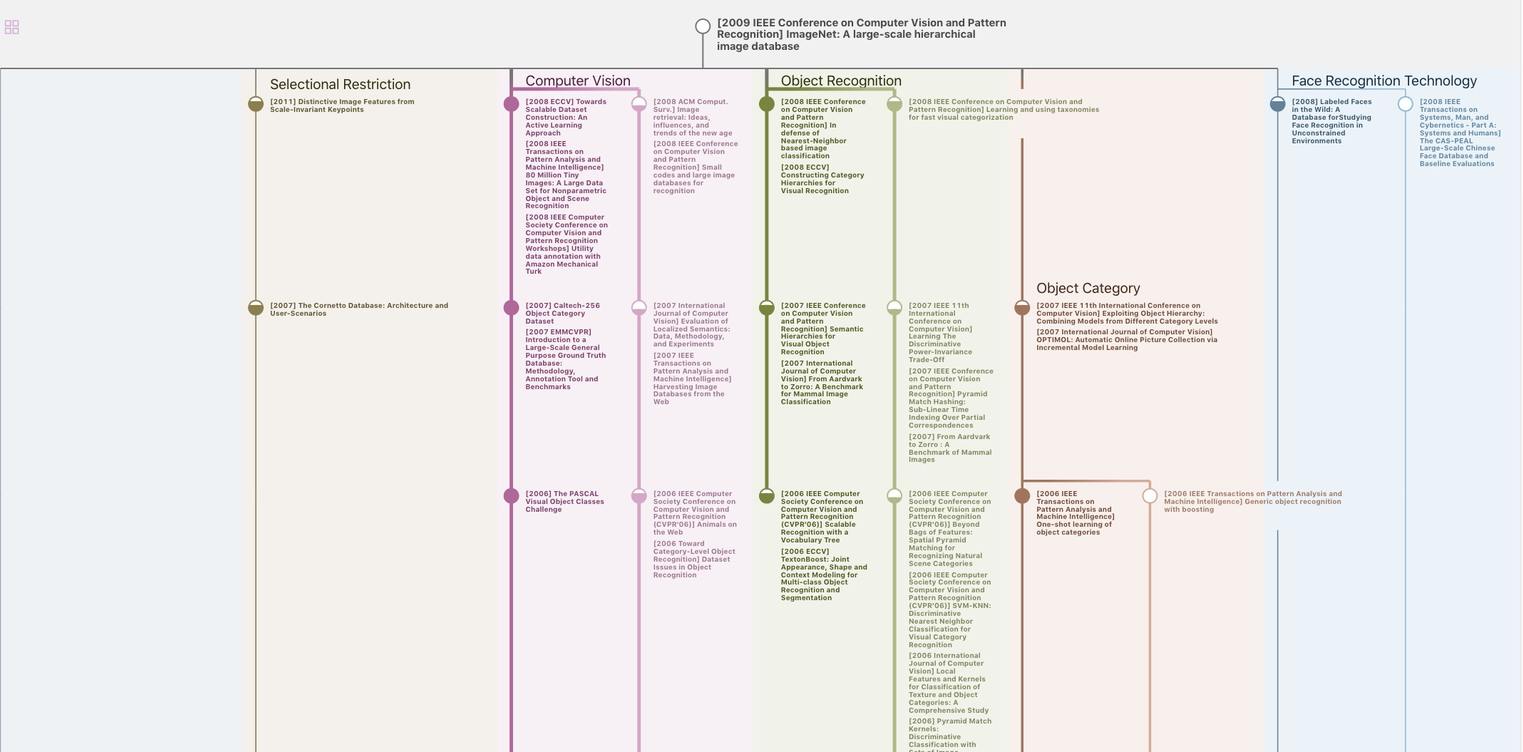
生成溯源树,研究论文发展脉络
Chat Paper
正在生成论文摘要