Accelerate gas diffusion-weighted MRI for lung morphometry with deep learning
European Radiology(2021)
摘要
Objectives Multiple b -value gas diffusion-weighted MRI (DW-MRI) enables non-invasive and quantitative assessment of lung morphometry, but its long acquisition time is not well-tolerated by patients. We aimed to accelerate multiple b -value gas DW-MRI for lung morphometry using deep learning. Methods A deep cascade of residual dense network (DC-RDN) was developed to reconstruct high-quality DW images from highly undersampled k-space data. Hyperpolarized 129 Xe lung ventilation images were acquired from 101 participants and were retrospectively collected to generate synthetic DW-MRI data to train the DC-RDN. Afterwards, the performance of the DC-RDN was evaluated on retrospectively and prospectively undersampled multiple b -value 129 Xe MRI datasets. Results Each slice with size of 64 × 64 × 5 could be reconstructed within 7.2 ms. For the retrospective test data, the DC-RDN showed significant improvement on all quantitative metrics compared with the conventional reconstruction methods ( p < 0.05). The apparent diffusion coefficient (ADC) and morphometry parameters were not significantly different between the fully sampled and DC-RDN reconstructed images ( p > 0.05). For the prospectively accelerated acquisition, the required breath-holding time was reduced from 17.8 to 4.7 s with an acceleration factor of 4. Meanwhile, the prospectively reconstructed results showed good agreement with the fully sampled images, with a mean difference of −0.72% and −0.74% regarding global mean ADC and mean linear intercept ( L m ) values. Conclusions DC-RDN is effective in accelerating multiple b -value gas DW-MRI while maintaining accurate estimation of lung microstructural morphometry, facilitating the clinical potential of studying lung diseases with hyperpolarized DW-MRI. Key Points • The deep cascade of residual dense network allowed fast and high-quality reconstruction of multiple b-value gas diffusion-weighted MRI at an acceleration factor of 4 . • The apparent diffusion coefficient and morphometry parameters were not significantly different between the fully sampled images and the reconstructed results (p > 0.05) . • The required breath-holding time was reduced from 17.8 to 4.7 s and each slice with size of 64 × 64 × 5 could be reconstructed within 7.2 ms .
更多查看译文
关键词
Deep learning,Diffusion magnetic resonance imaging,Lung
AI 理解论文
溯源树
样例
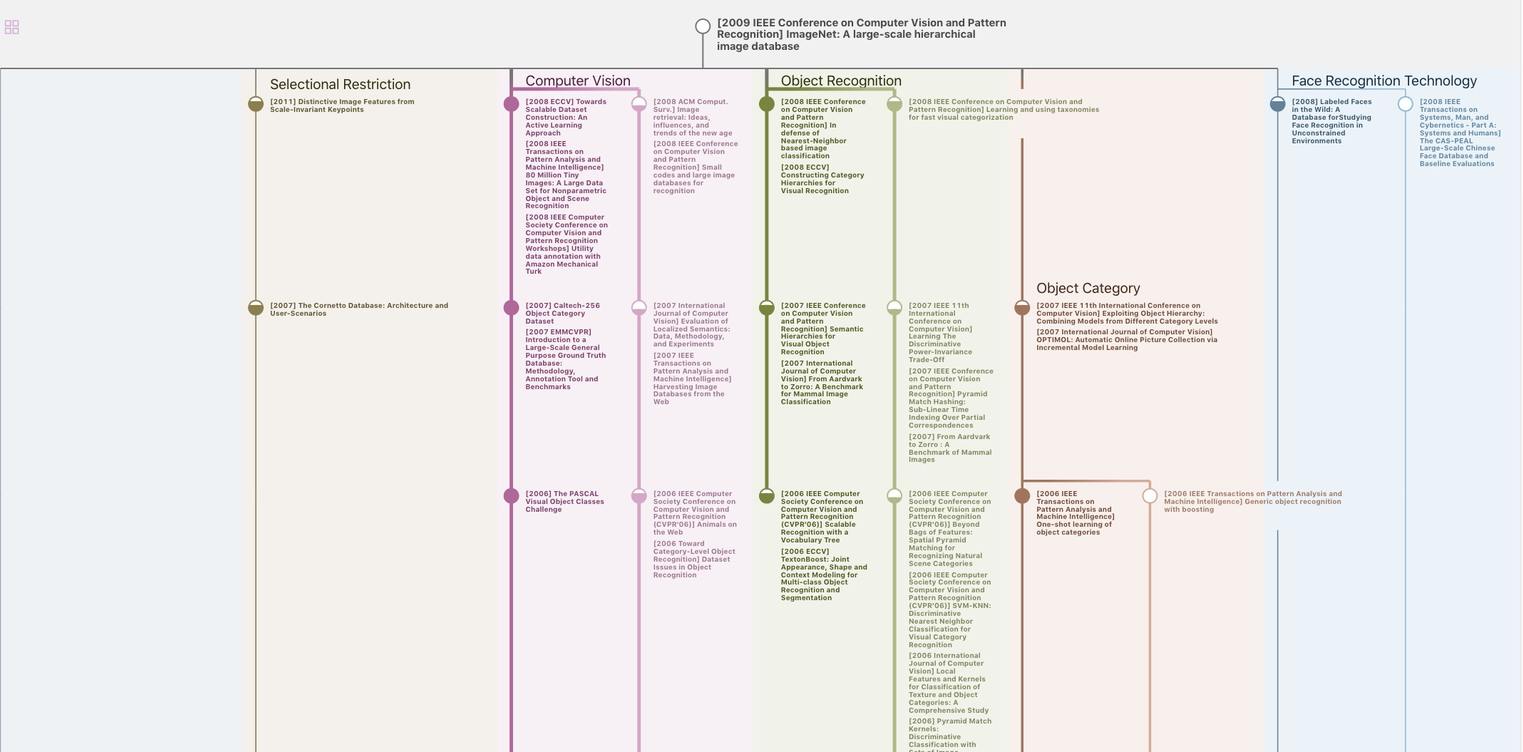
生成溯源树,研究论文发展脉络
Chat Paper
正在生成论文摘要