Meta-learning PINN loss functions
Journal of Computational Physics(2022)
摘要
•We propose a meta-learning technique for offline discovery of physics-informed neural network (PINN) loss functions.•Based on new theory, we identify two desirable properties of meta-learned losses in PINN problems.•We enforce the identified properties by proposing a regularization method or using a specific loss parametrization.•In the examples, the meta-learned losses are employed at test time for addressing regression and PDE task distributions.•We achieve significant performance improvement by using a meta-learned loss function even for out-of-distribution data.•We consider various loss parametrizations and algorithm design options and describe how they may affect performance.
更多查看译文
关键词
Physics-informed neural networks,Meta-learning,Meta-learned loss function
AI 理解论文
溯源树
样例
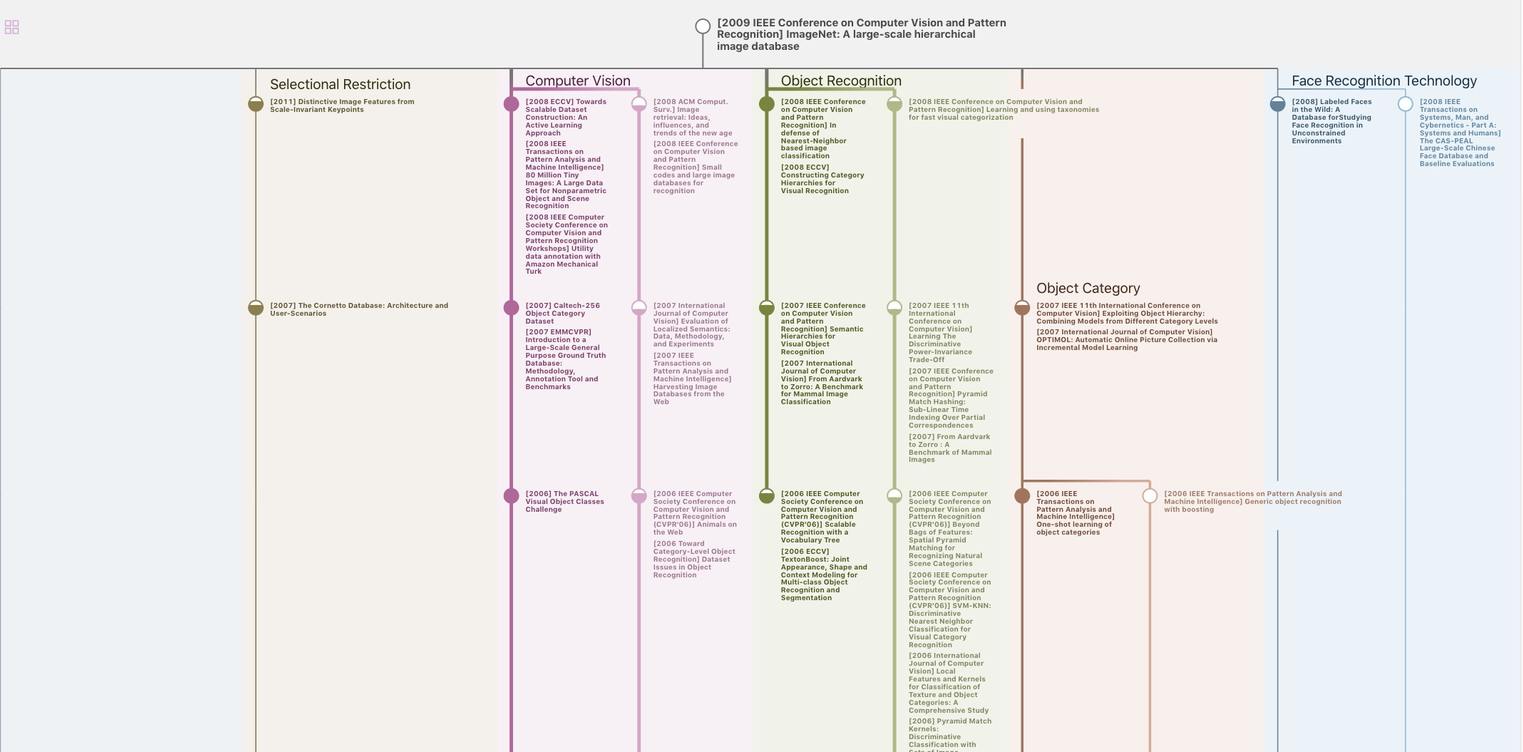
生成溯源树,研究论文发展脉络
Chat Paper
正在生成论文摘要