Behavior Constraining in Weight Space for Offline Reinforcement Learning.
The European Symposium on Artificial Neural Networks (ESANN)(2021)
摘要
In offline reinforcement learning, a policy needs to be learned from a single pre-collected dataset. Typically, policies are thus regularized during training to behave similarly to the data generating policy, by adding a penalty based on a divergence between action distributions of generating and trained policy. We propose a new algorithm, which constrains the policy directly in its weight space instead, and demonstrate its effectiveness in experiments.
更多查看译文
关键词
reinforcement learning,weight space,behavior
AI 理解论文
溯源树
样例
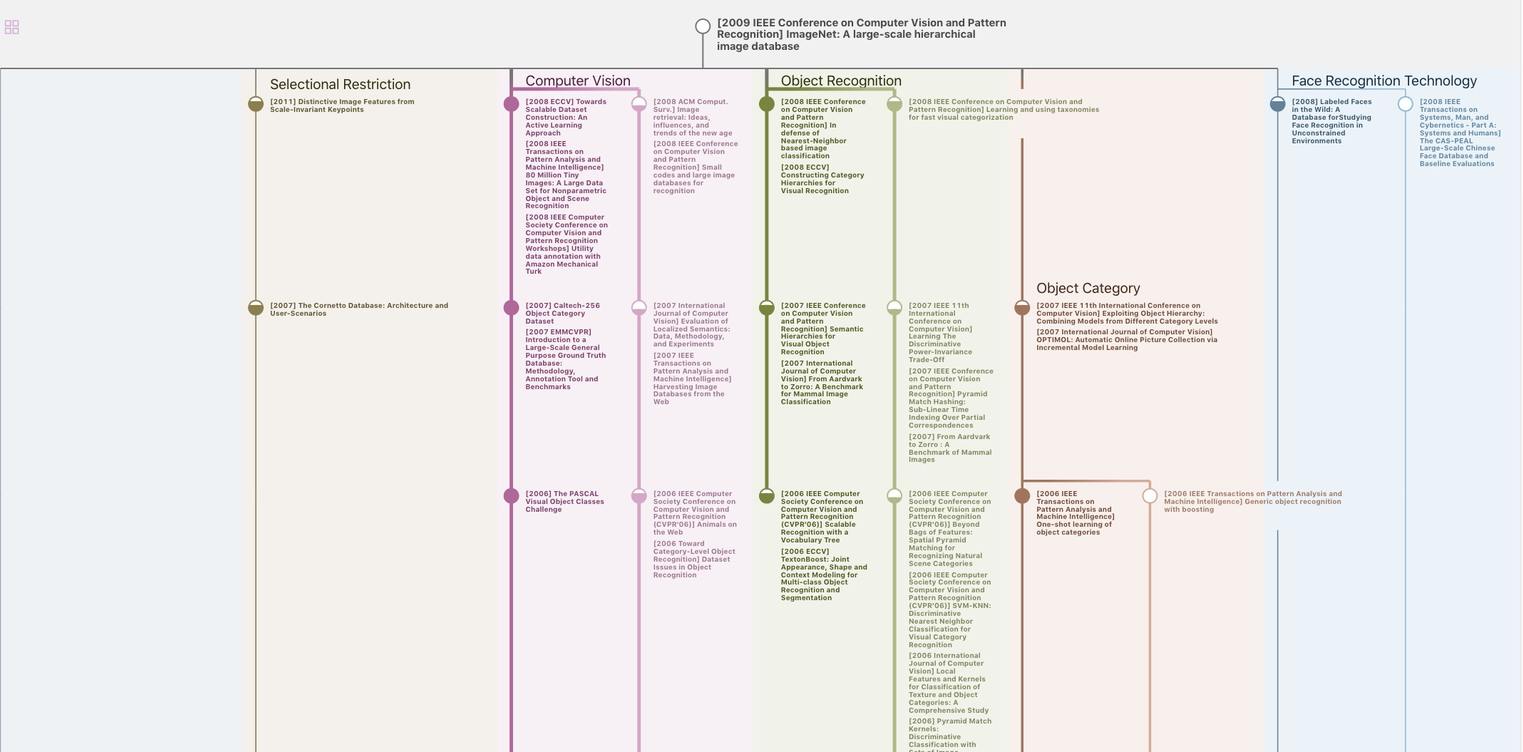
生成溯源树,研究论文发展脉络
Chat Paper
正在生成论文摘要