Greedy structure learning from data that contain systematic missing values
Machine Learning(2022)
摘要
Learning from data that contain missing values represents a common phenomenon in many domains. Relatively few Bayesian Network structure learning algorithms account for missing data, and those that do tend to rely on standard approaches that assume missing data are missing at random, such as the Expectation-Maximisation algorithm. Because missing data are often systematic, there is a need for more pragmatic methods that can effectively deal with data sets containing missing values not missing at random. The absence of approaches that deal with systematic missing data impedes the application of BN structure learning methods to real-world problems where missingness are not random. This paper describes three variants of greedy search structure learning that utilise pairwise deletion and inverse probability weighting to maximally leverage the observed data and to limit potential bias caused by missing values. The first two of the variants can be viewed as sub-versions of the third and best performing variant, but are important in their own in illustrating the successive improvements in learning accuracy. The empirical investigations show that the proposed approach outperforms the commonly used and state-of-the-art Structural EM algorithm, both in terms of learning accuracy and efficiency, as well as both when data are missing at random and not at random.
更多查看译文
关键词
Expectation-maximisation, Inverse probability weighting, Missing data, Score-based learning, Structure learning
AI 理解论文
溯源树
样例
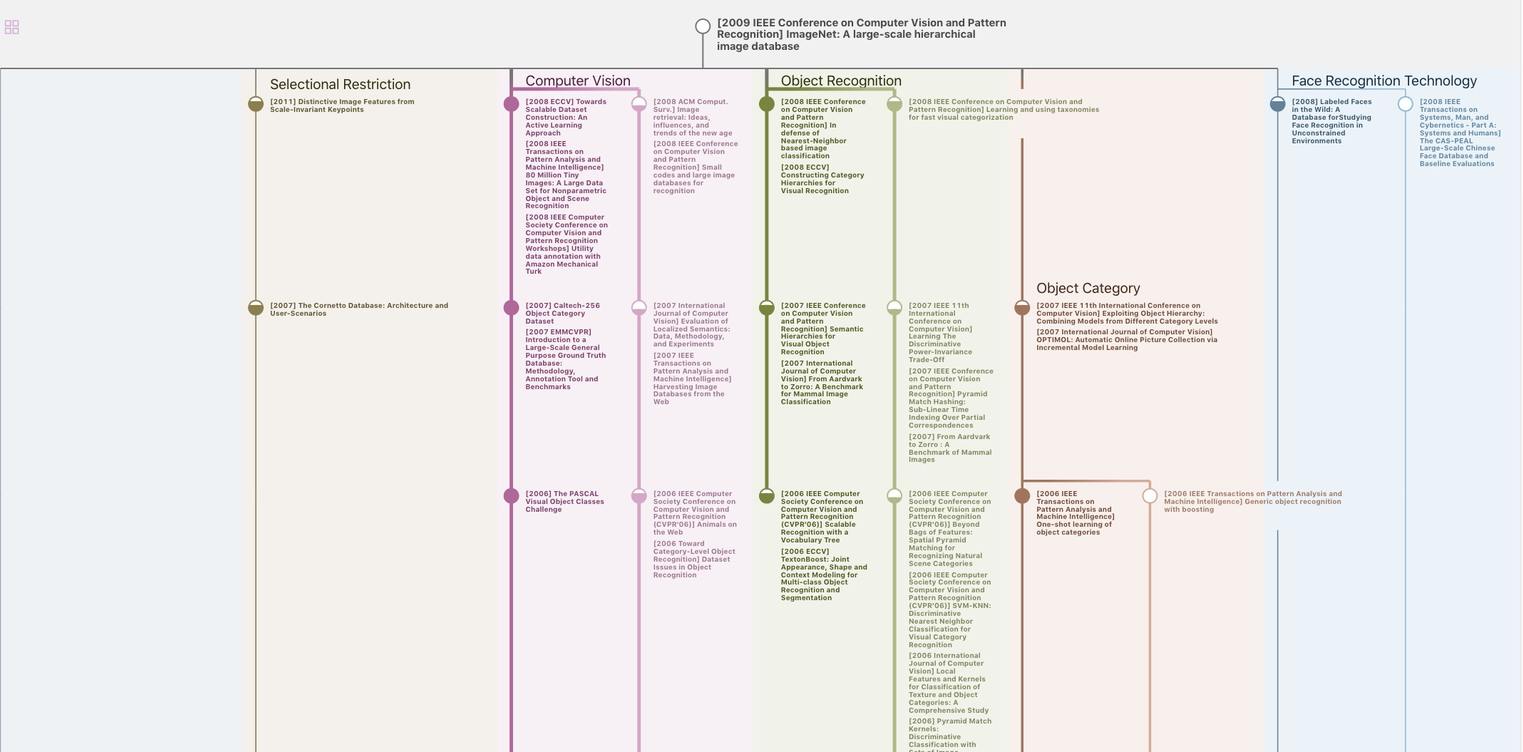
生成溯源树,研究论文发展脉络
Chat Paper
正在生成论文摘要