TGHop: an explainable, efficient, and lightweight method for texture generation
APSIPA TRANSACTIONS ON SIGNAL AND INFORMATION PROCESSING(2021)
摘要
An explainable, efficient, and lightweight method for texture generation, called TGHop (an acronym of Texture Generation PixelHop), is proposed in this work. Although synthesis of visually pleasant texture can be achieved by deep neural networks, the associated models are large in size, difficult to explain in theory, and computationally expensive in training. In contrast, TGHop is small in its model size, mathematically transparent, efficient in training and inference, and able to generate high-quality texture. Given an exemplary texture, TGHop first crops many sample patches out of it to form a collection of sample patches called the source. Then, it analyzes pixel statistics of samples from the source and obtains a sequence of fine-to-coarse subspaces for these patches by using the PixelHop++ framework. To generate texture patches with TGHop, we begin with the coarsest subspace, which is called the core, and attempt to generate samples in each subspace by following the distribution of real samples. Finally, texture patches are stitched to form texture images of a large size. It is demonstrated by experimental results that TGHop can generate texture images of superior quality with a small model size and at a fast speed.
更多查看译文
关键词
Texture generation, Texture synthesis, Generative model, Successive subspace modeling
AI 理解论文
溯源树
样例
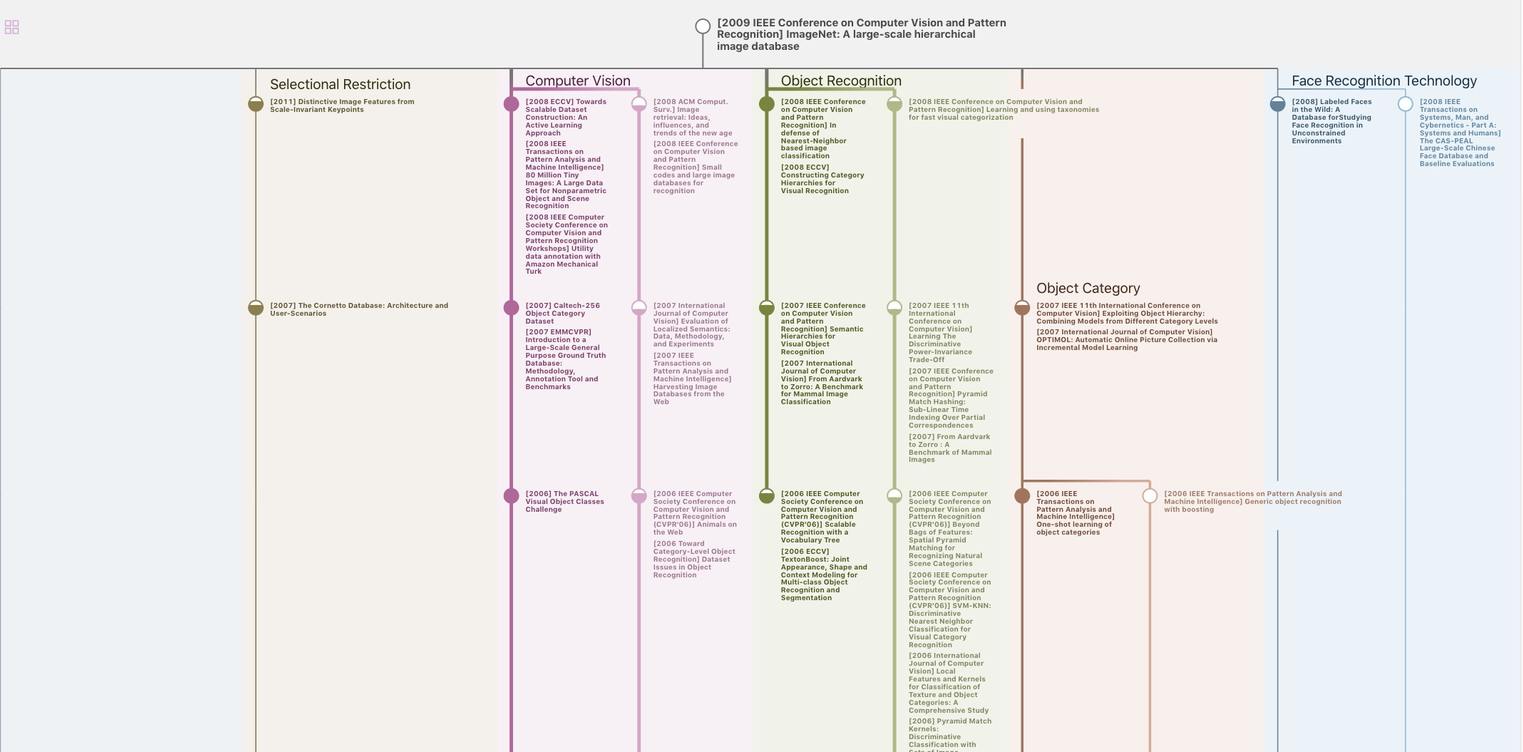
生成溯源树,研究论文发展脉络
Chat Paper
正在生成论文摘要