Atlas-based Segmentation of Intracochlear Anatomy in Metal Artifact Affected CT Images of the Ear with Co-trained Deep Neural Networks
MEDICAL IMAGE COMPUTING AND COMPUTER ASSISTED INTERVENTION - MICCAI 2021, PT IV(2021)
摘要
We propose an atlas-based method to segment the intracochlear anatomy (ICA) in the post-implantation CT (Post-CT) images of cochlear implant (CI) recipients that preserves the point-to-point correspondence between the meshes in the atlas and the segmented volumes. To solve this problem, which is challenging because of the strong artifacts produced by the implant, we use a pair of co-trained deep networks that generate dense deformation fields (DDFs) in opposite directions. One network is tasked with registering an atlas image to the Post-CT images and the other network is tasked with registering the Post-CT images to the atlas image. The networks are trained using loss functions based on voxel-wise labels, image content, fiducial registration error, and cycle-consistency constraint. The segmentation of the ICA in the Post-CT images is subsequently obtained by transferring the predefined segmentation meshes of the ICA in the atlas image to the Post-CT images using the corresponding DDFs generated by the trained registration networks. Our model can learn the underlying geometric features of the ICA even though they are obscured by the metal artifacts. We show that our end-to-end network produces results that are comparable to the current state of the art (SOTA) that relies on a two-steps approach that first uses conditional generative adversarial networks to synthesize artifact-free images from the Post-CT images and then uses an active shape model-based method to segment the ICA in the synthetic images. Our method requires a fraction of the time needed by the SOTA, which is important for end-user acceptance.
更多查看译文
关键词
Non-rigid registration, Atlas-based segmentation, Metal artifact, Cochlear implant
AI 理解论文
溯源树
样例
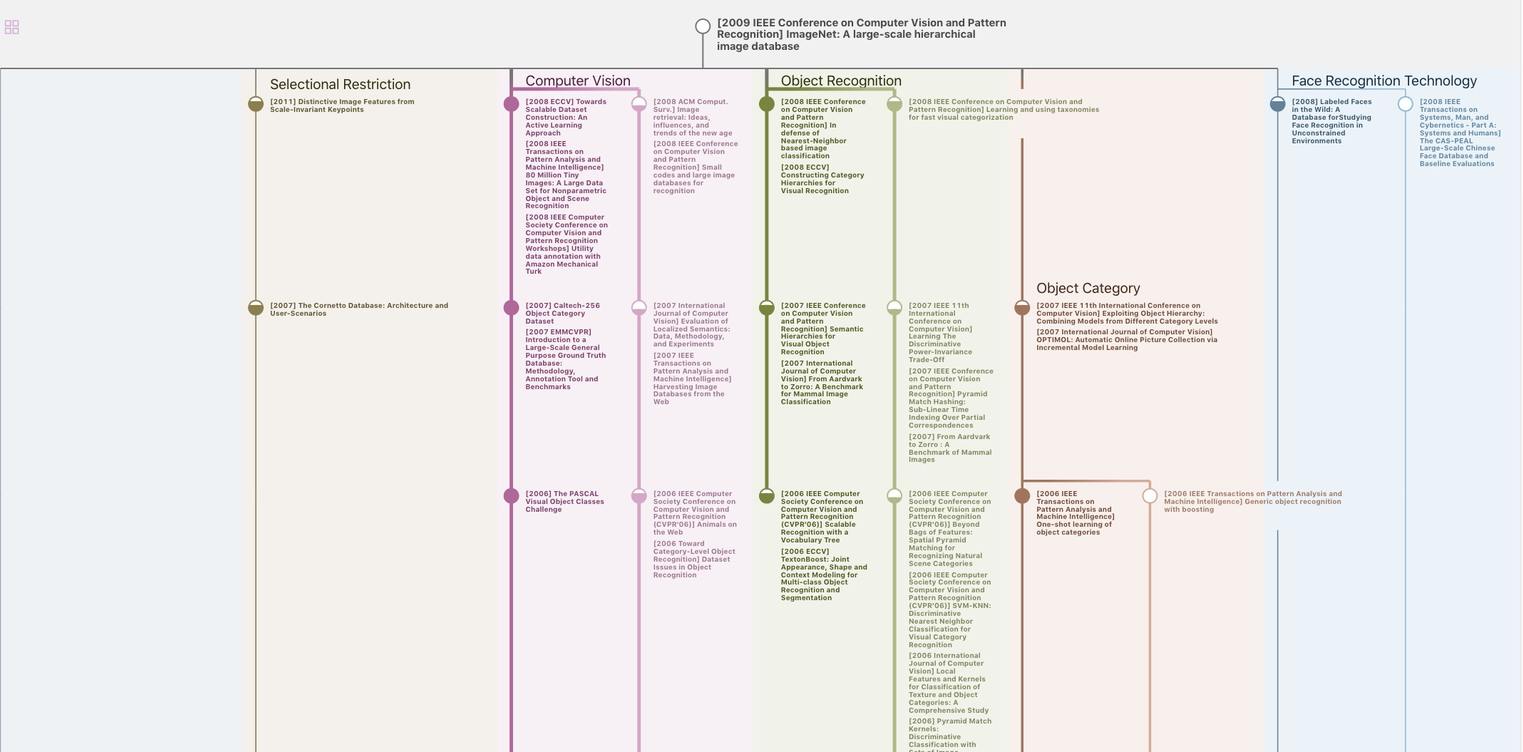
生成溯源树,研究论文发展脉络
Chat Paper
正在生成论文摘要