Comparing Supervised Models and Learned Speech Representations for Classifying Intelligibility of Disordered Speech on Selected Phrases.
Interspeech(2021)
摘要
Automatic classification of disordered speech can provide an objective tool for identifying the presence and severity of speech impairment. Classification approaches can also help identify hard-to-recognize speech samples to teach ASR systems about the variable manifestations of impaired speech. Here, we develop and compare different deep learning techniques to classify the intelligibility of disordered speech on selected phrases. We collected samples from a diverse set of 661 speakers with a variety of self-reported disorders speaking 29 words or phrases, which were rated by speech-language pathologists for their overall intelligibility using a five-point Likert scale. We then evaluated classifiers developed using 3 approaches: (1) a convolutional neural network (CNN) trained for the task, (2) classifiers trained on non-semantic speech representations from CNNs that used an unsupervised objective [1], and (3) classifiers trained on the acoustic (encoder) embeddings from an ASR system trained on typical speech [2]. We found that the ASR encoder's embeddings considerably outperform the other two on detecting and classifying disordered speech. Further analysis shows that the ASR embeddings cluster speech by the spoken phrase, while the non-semantic embeddings cluster speech by speaker. Also, longer phrases are more indicative of intelligibility deficits than single words.
更多查看译文
关键词
atypical speech,classification,intelligibility,speech disorders
AI 理解论文
溯源树
样例
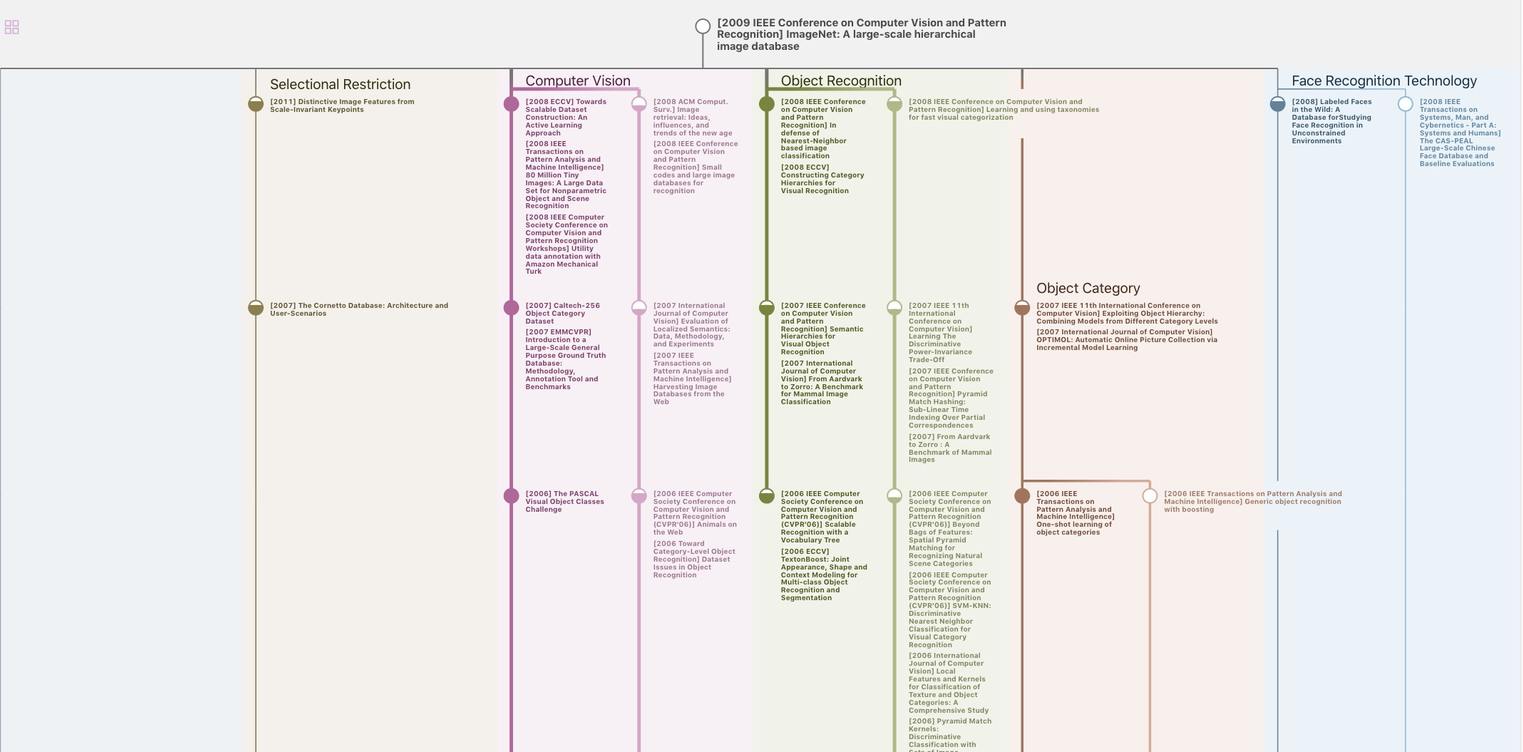
生成溯源树,研究论文发展脉络
Chat Paper
正在生成论文摘要