Zeroth-order optimization with orthogonal random directions
arxiv(2022)
摘要
We propose and analyze a randomized zeroth-order optimization method based on approximating the exact gradient by finite differences computed in a set of orthogonal random directions that changes with each iteration. A number of previously proposed methods are recovered as special cases including spherical smoothing, coordinate descent, as well as discretized gradient descent. Our main contribution is proving convergence guarantees as well as convergence rates under different parameter choices and assumptions. In particular, we consider convex objectives, but also possibly non-convex objectives satisfying the Polyak-Łojasiewicz (PL) condition. Theoretical results are complemented and illustrated by numerical experiments.
更多查看译文
关键词
Zeroth-order optimization, Derivative-free methods, Stochastic algorithms, Polyak-Lojasiewicz inequality, Convex programming, Finite differences approximation, Random search
AI 理解论文
溯源树
样例
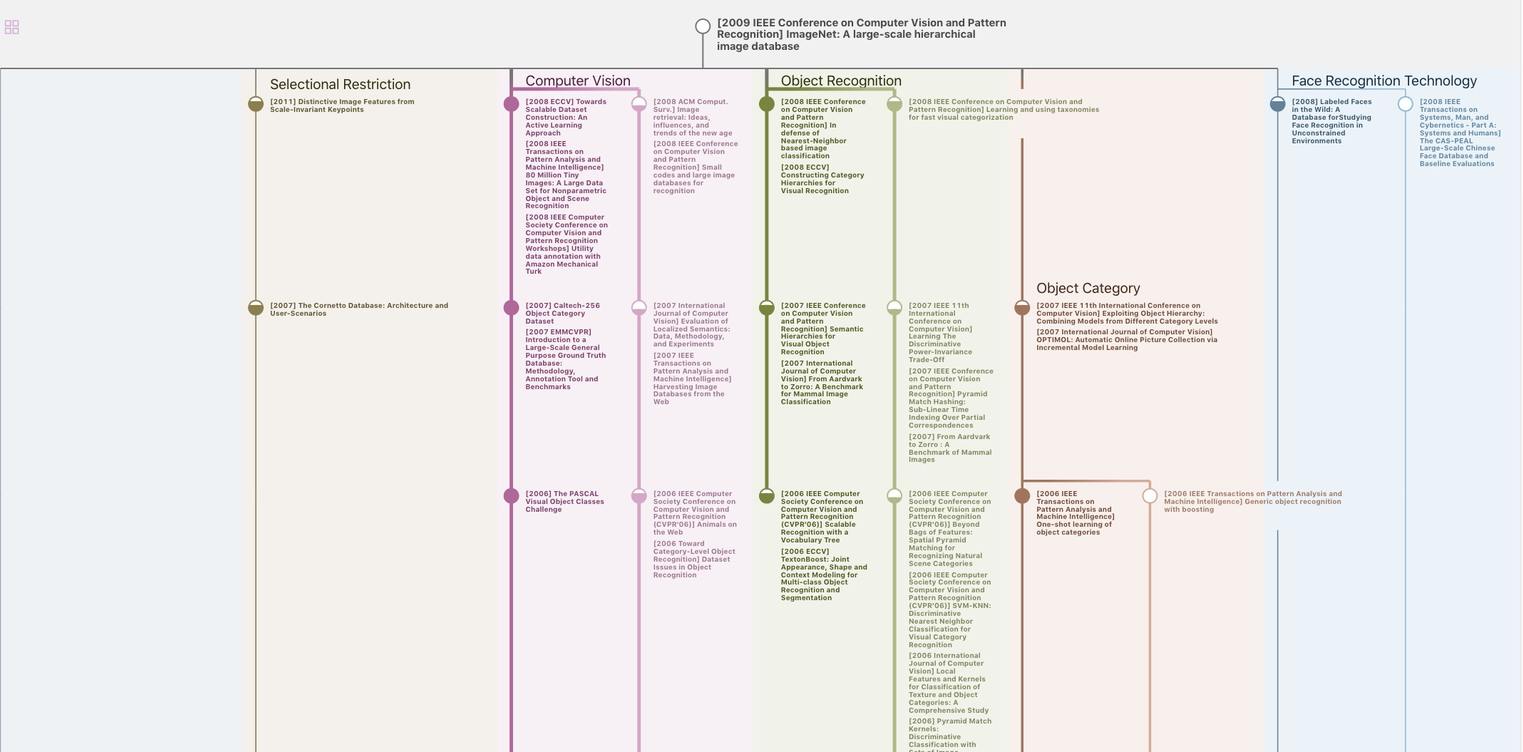
生成溯源树,研究论文发展脉络
Chat Paper
正在生成论文摘要