Joint Motion Correction and Super Resolution for Cardiac Segmentation via Latent Optimisation
MEDICAL IMAGE COMPUTING AND COMPUTER ASSISTED INTERVENTION - MICCAI 2021, PT III(2021)
摘要
In cardiac magnetic resonance (CMR) imaging, a 3D high-resolution segmentation of the heart is essential for detailed description of its anatomical structures. However, due to the limit of acquisition duration and respiratory/cardiac motion, stacks of multi-slice 2D images are acquired in clinical routine. The segmentation of these images provides a low-resolution representation of cardiac anatomy, which may contain artefacts caused by motion. Here we propose a novel latent optimisation framework that jointly performs motion correction and super resolution for cardiac image segmentations. Given a low-resolution segmentation as input, the framework accounts for inter-slice motion in cardiac MR imaging and super-resolves the input into a high-resolution segmentation consistent with input. A multi-view loss is incorporated to leverage information from both short-axis view and long-axis view of cardiac imaging. To solve the inverse problem, iterative optimisation is performed in a latent space, which ensures the anatomical plausibility. This alleviates the need of paired low-resolution and high-resolution images for supervised learning. Experiments on two cardiac MR datasets show that the proposed framework achieves high performance, comparable to state-of-the-art super-resolution approaches and with better cross-domain generalisability and anatomical plausibility. The codes are available at https:// github.com/shuowang26/SRHeart.
更多查看译文
关键词
Super-resolution, Motion correction, Cardiac MR
AI 理解论文
溯源树
样例
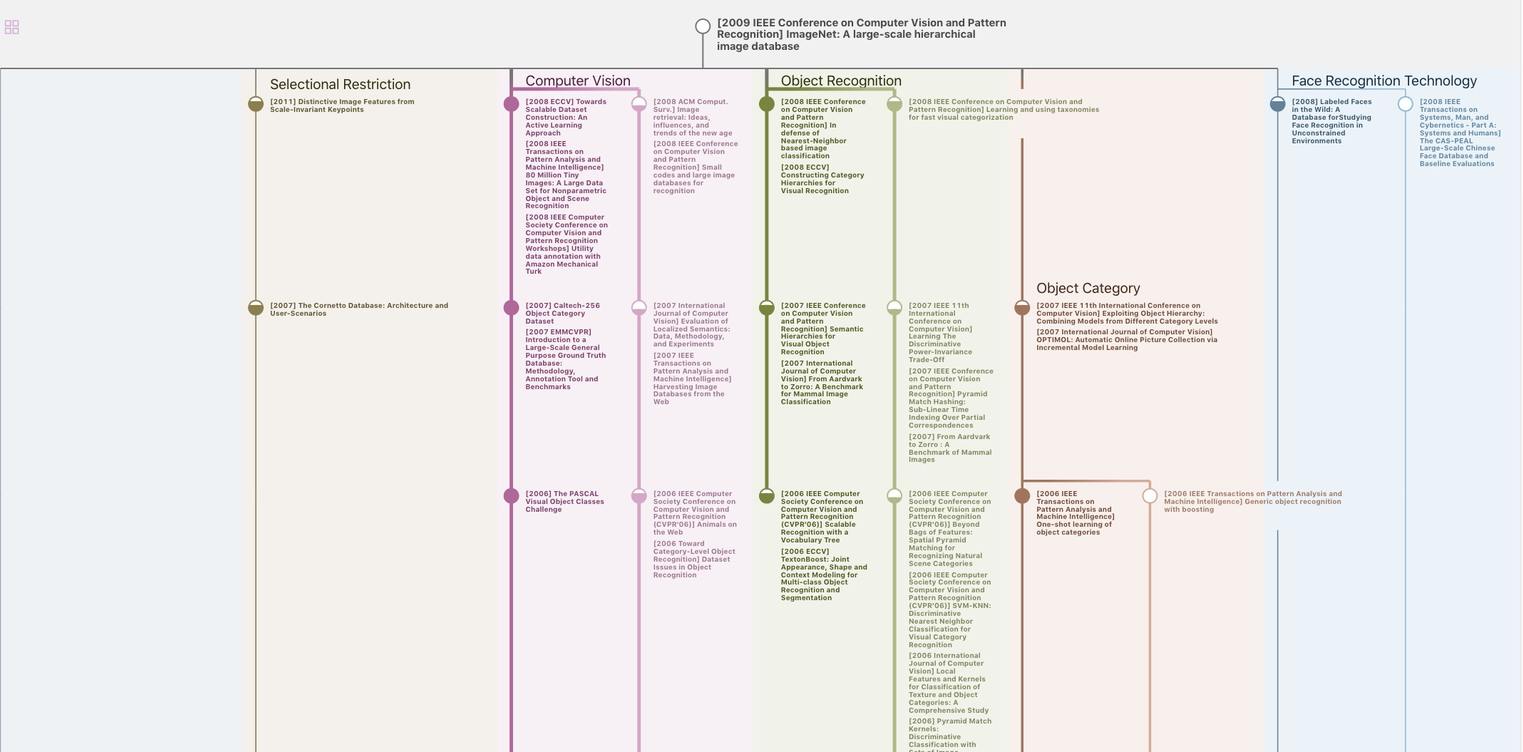
生成溯源树,研究论文发展脉络
Chat Paper
正在生成论文摘要