AdaReNet - Adaptive Reweighted Semi-supervised Active Learning to Accelerate Label Acquisition.
PETRA(2021)
摘要
Data scarcity and quality pose significant challenges to supervised learning. The process of generating informative annotations can be time-consuming and often requires high domain expertise. Active and semi-supervised learning methods can reduce labeling effort by either automatically expanding the training set or by selecting the most informative examples to request domain expert annotation. As most selection methods are heuristic, the performance varies widely across datasets and tasks. Bootstrapping approaches such as self-training can result in negative effects due to the addition of incorrectly pseudo-labeled instances. In this work, we take a holistic approach to label acquisition and consider the expansion of clean and pseudo-labeled subsets jointly. To address the challenge of producing high-quality pseudo-labels, we introduce a collaborative teacher-student framework, where the teacher, termed AdaReNet, learns a data-driven curriculum. Experimental results on several natural language processing (NLP) tasks demonstrate that the proposed framework outperforms baselines.
更多查看译文
AI 理解论文
溯源树
样例
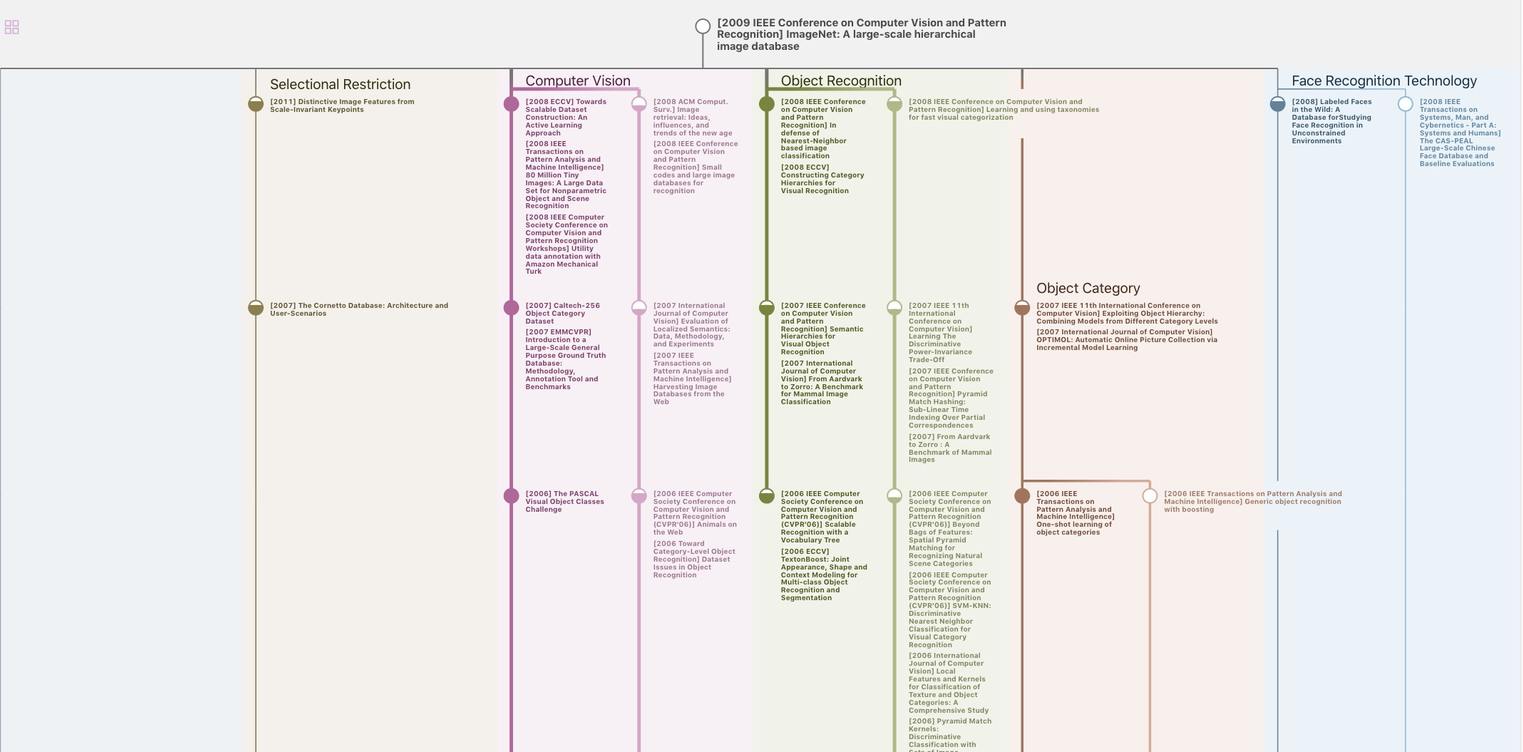
生成溯源树,研究论文发展脉络
Chat Paper
正在生成论文摘要