Predicting Exporters with Machine Learning
arxiv(2022)
摘要
In this contribution, we exploit machine learning techniques to evaluate whether and how close firms are to becoming successful exporters. First, we train and test various algorithms using financial information on both exporters and non-exporters in France in 2010-2018. Thus, we show that we are able to predict the distance of non-exporters from export status. In particular, we find that a Bayesian Additive Regression Tree with Missingness In Attributes (BART-MIA) performs better than other techniques with an accuracy of up to 0.90. Predictions are robust to changes in definitions of exporters and in the presence of discontinuous exporting activity. Eventually, we discuss how our exporting scores can be helpful for trade promotion, trade credit, and assessing aggregate trade potential. For example, back-of-the-envelope estimates show that a representative firm with just below-average exporting scores needs up to 44% more cash resources and up to 2.5 times more capital to get to foreign markets.
更多查看译文
关键词
exporting, machine learning, trade promotion, trade finance, competitiveness
AI 理解论文
溯源树
样例
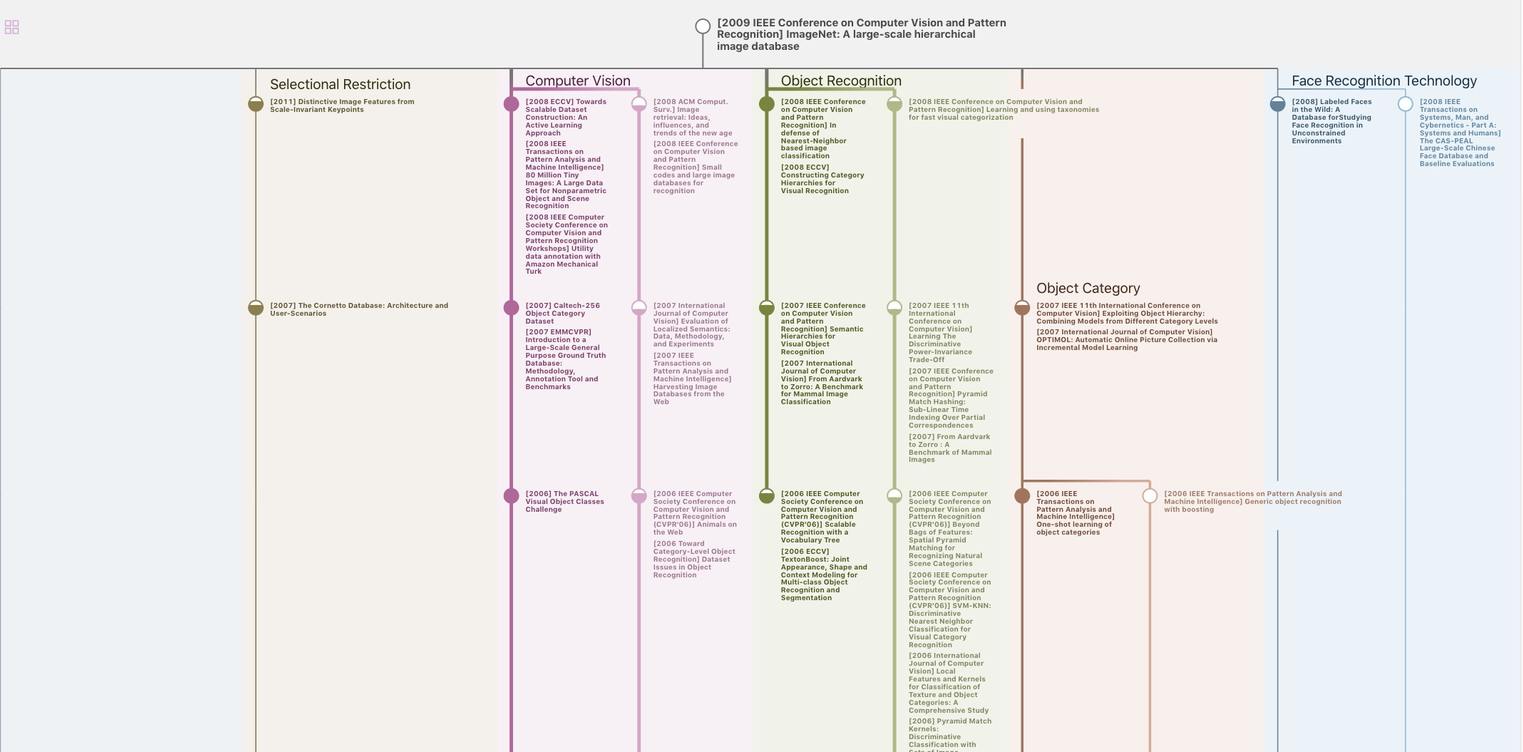
生成溯源树,研究论文发展脉络
Chat Paper
正在生成论文摘要