Bayesian Nonparametric Modelling for Model-Free Reinforcement Learning in LTE-LAA and Wi-Fi Coexistence
arxiv(2021)
摘要
With the arrival of next generation wireless communication, a growing number of new applications like internet of things, autonomous driving systems, and drone are crowding the unlicensed spectrum. Licensed network such as the long-term evolution (LTE) also comes to the unlicensed spectrum for better providing high-capacity contents with low cost. However, LTE was not designed to share resources with others. Previous solutions usually work on fixed scenarios. This work features a Nonparametric Bayesian reinforcement learning algorithm to cope with the coexistence between Wi-Fi and LTE licensed assisted access (LTE-LAA) agents in 5 GHz unlicensed spectrum. The coexistence problem is modeled as a decentralized partially-observable Markov decision process (Dec-POMDP) and Bayesian inference is adopted for policy learning with nonparametric prior to accommodate the uncertainty of policy for different agents. A fairness measure is introduced in the reward function to encourage fair sharing between agents. Variational inference for posterior model approximation is considered to make the algorithm computationally efficient. Simulation results demonstrate that this algorithm can reach high value with compact policy representations in few learning iterations.
更多查看译文
AI 理解论文
溯源树
样例
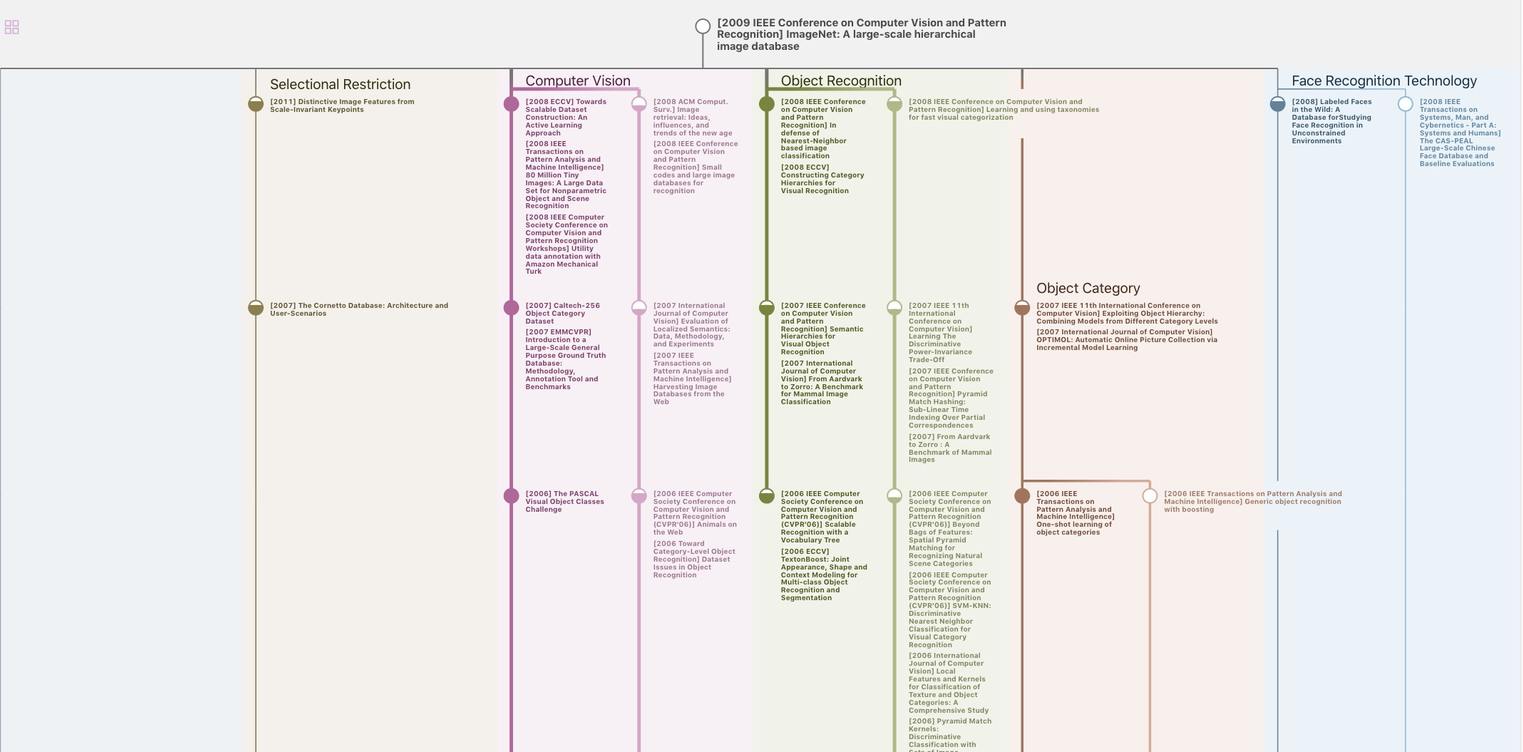
生成溯源树,研究论文发展脉络
Chat Paper
正在生成论文摘要