Multi-Modal Motion Planning Using Composite Pose Graph Optimization
2021 IEEE INTERNATIONAL CONFERENCE ON ROBOTICS AND AUTOMATION (ICRA 2021)(2021)
摘要
In this paper, we present a motion planning framework for multi-modal vehicle dynamics. Our proposed algorithm employs transcription of the optimization objective function, vehicle dynamics, and state and control constraints into sparse factor graphs, which—combined with mode transition constraints—constitute a composite pose graph. By formulating the multi-modal motion planning problem in composite pose graph form, we enable utilization of efficient techniques for optimization on sparse graphs, such as those widely applied in dual estimation problems, e.g., simultaneous localization and mapping (SLAM). The resulting motion planning algorithm optimizes the multi-modal trajectory, including the location of mode transitions, and is guided by the pose graph optimization process to eliminate unnecessary transitions, enabling efficient discovery of optimized mode sequences from rough initial guesses. We demonstrate multi-modal trajectory optimization in both simulation and real-world experiments for vehicles with various dynamics models, such as an airplane with taxi and flight modes, and a vertical take-off and landing (VTOL) fixed-wing aircraft that transitions between hover and horizontal flight modes.
更多查看译文
关键词
composite pose graph optimization,planning,motion,multi-modal
AI 理解论文
溯源树
样例
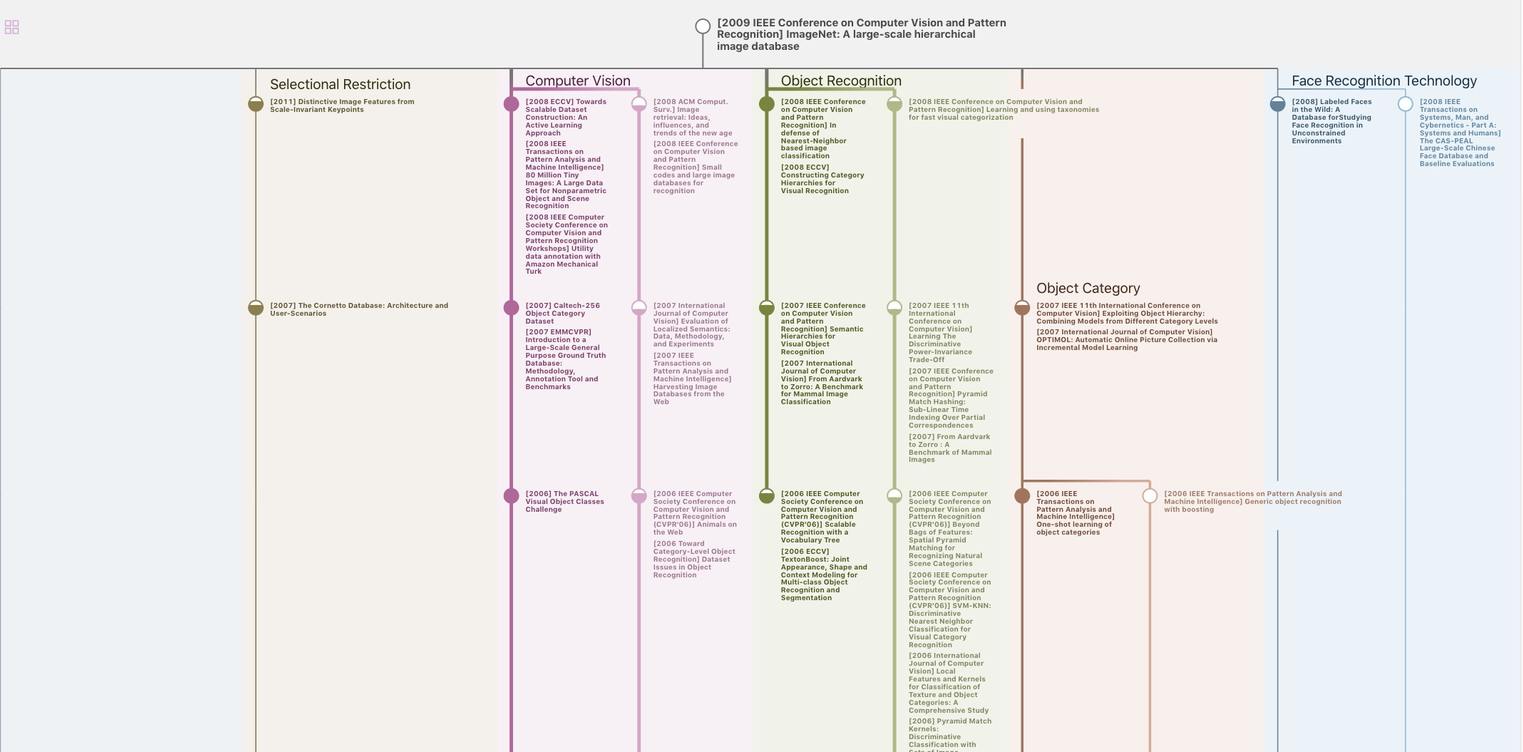
生成溯源树,研究论文发展脉络
Chat Paper
正在生成论文摘要