Time series graphical lasso and sparse VAR estimation
COMPUTATIONAL STATISTICS & DATA ANALYSIS(2022)
摘要
A two-stage sparse vector autoregression method is proposed. It relies on the more recent and powerful technique of time series graphical lasso to estimate sparse inverse spectral density matrices in the first stage, and its second stage refines non-zero entries of the AR coefficient matrices using a false discovery rate (FDR) procedure. Compared to a recent approach, the method has the advantage of avoiding the inversion of the spectral density matrix, but has to deal with optimization over Hermitian matrices with complex -valued entries. Such modifications significantly improve the computational time with a little loss in forecasting performance. The algorithmic and computational properties of the method have been studied and the performance of the two methods is compared using simulated and a real macro-economic dataset. The simulation results show that the proposed modification is preferred over the existing method when the goal is to learn the structure of the AR coefficient matrices while the latter outperforms the former when forecasting is the ultimate task. (C) 2022 Elsevier B.V. All rights reserved.
更多查看译文
关键词
Time series graphical models, Sparse vector autoregression, FDR
AI 理解论文
溯源树
样例
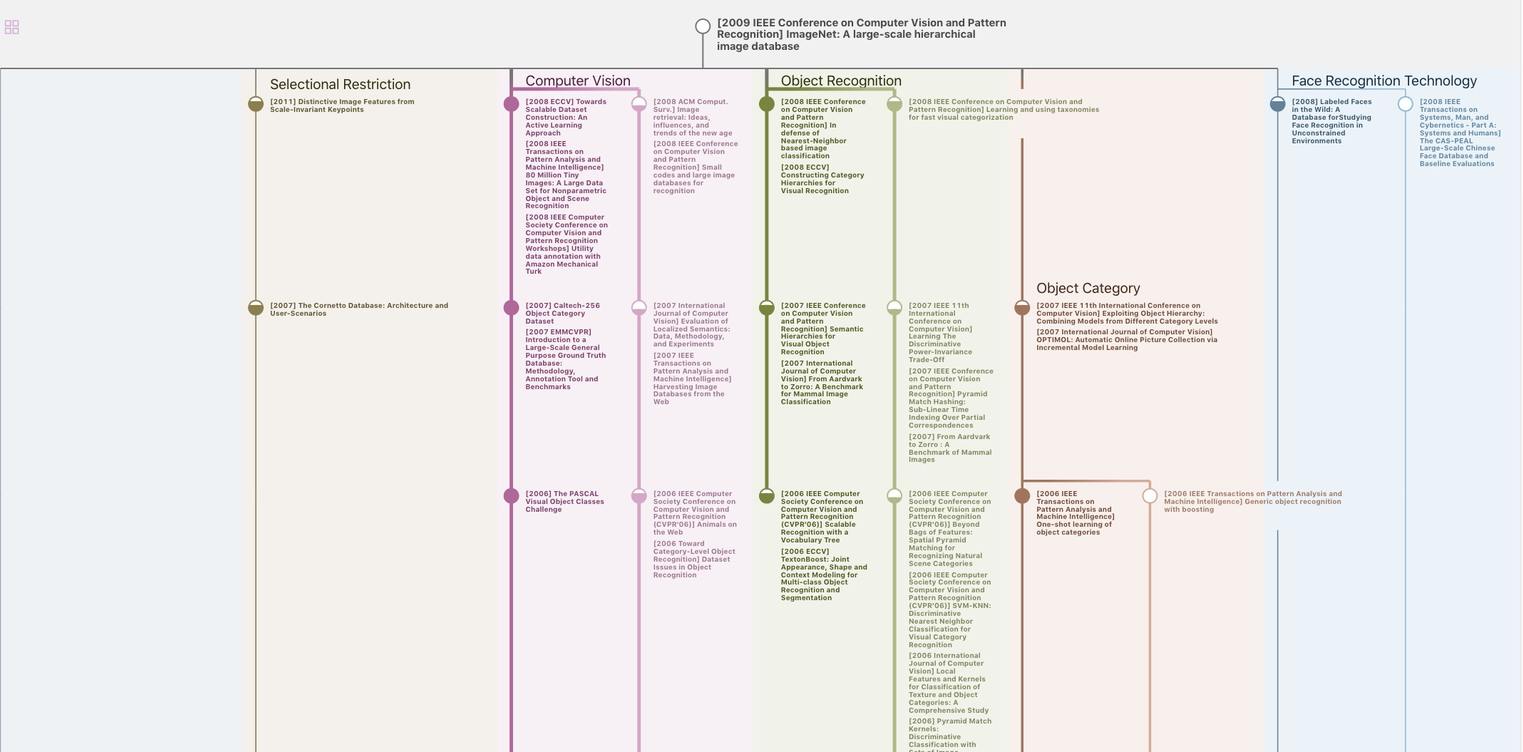
生成溯源树,研究论文发展脉络
Chat Paper
正在生成论文摘要