AdaL: Adaptive Gradient Transformation Contributes to Convergences and Generalizations
arxiv(2021)
摘要
Adaptive optimization methods have been widely used in deep learning. They scale the learning rates adaptively according to the past gradient, which has been shown to be effective to accelerate the convergence. However, they suffer from poor generalization performance compared with SGD. Recent studies point that smoothing exponential gradient noise leads to generalization degeneration phenomenon. Inspired by this, we propose AdaL, with a transformation on the original gradient. AdaL accelerates the convergence by amplifying the gradient in the early stage, as well as dampens the oscillation and stabilizes the optimization by shrinking the gradient later. Such modification alleviates the smoothness of gradient noise, which produces better generalization performance. We have theoretically proved the convergence of AdaL and demonstrated its effectiveness on several benchmarks.
更多查看译文
关键词
adaptive gradient transformation contributes,generalizations
AI 理解论文
溯源树
样例
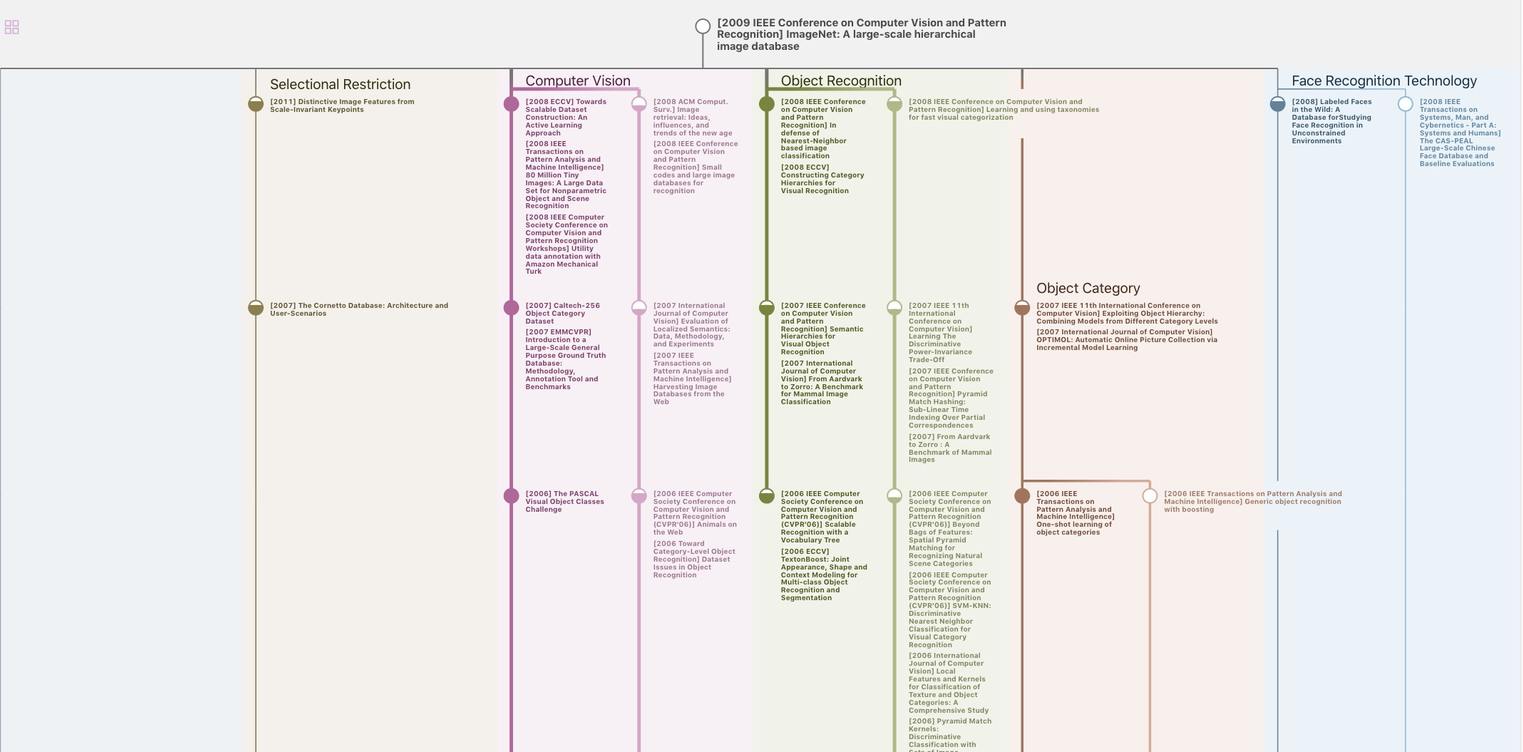
生成溯源树,研究论文发展脉络
Chat Paper
正在生成论文摘要