Short-term probabilistic photovoltaic power forecast based on deep convolutional long short-term memory network and kernel density estimation
arxiv(2021)
摘要
Solar energy is a clean and renewable energy. Photovoltaic (PV) power is an important way to utilize solar energy. Accurate PV power forecast is crucial to the large-scale application of PV power and the stability of electricity grid. This paper proposes a novel method for short-term photovoltaic power forecast using deep convolutional long short-term memory (ConvLSTM) network and kernel density estimation (KDE). In the proposed method, ConvLSTM is used to forecast the future photovoltaic power and KDE is used for estimating the joint probabilistic density function and giving the probabilistic confidence interval. Experiments in an actual photovoltaic power station verify the effectiveness of the proposed method. Comparison experiments with convolutional neural network (CNN) and long short-term memory network (LSTM)shows that ConvLSTM can combine the advantages of both CNN and LSTM and significantly outperform CNN and LSTM in terms of forecast accuracy. Through further comparison with other five conventional methods including multilayer perceptron (MLP), support vector regression (SVR), extreme learning machine (ELM), classification and regression tree (CART) and gradient boosting decision tree (GBDT), ConvLSTM can significantly improve the forecast accuracy by more than 20% for most of the five methods and the superiorities of ConvLSTM are further verified.
更多查看译文
关键词
probabilistic photovoltaic power forecast,kernel density estimation,short-term,short-term
AI 理解论文
溯源树
样例
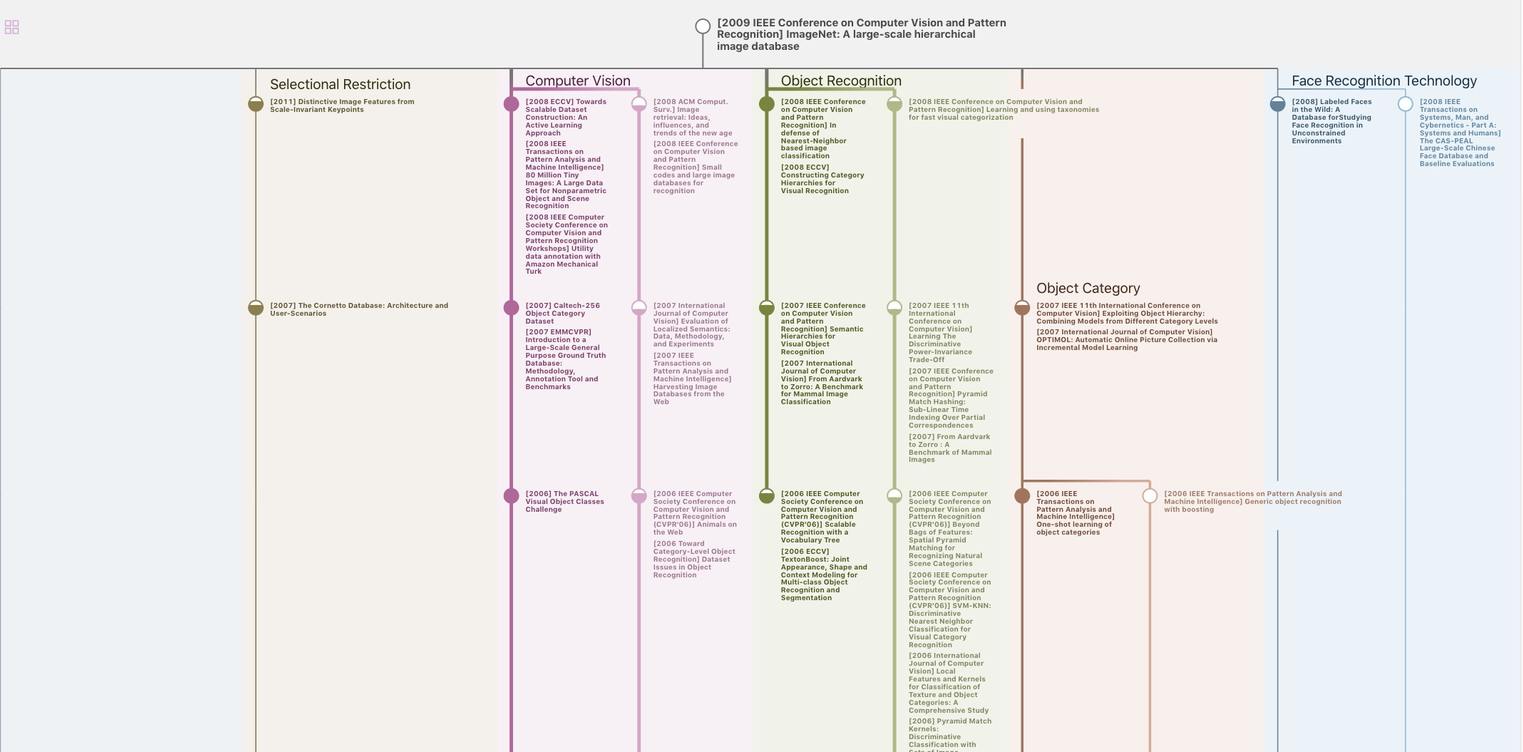
生成溯源树,研究论文发展脉络
Chat Paper
正在生成论文摘要