Ensemble of loss functions to improve generalizability of deep metric learning methods
Multimedia Tools and Applications(2024)
摘要
The success of a Deep metric learning (DML) algorithm greatly depends on its loss function. However, no loss function is perfect and deals only with some aspects of an optimal similarity embedding. Besides, they omit the generalizability of the DML on unseen categories. To address these challenges, we propose novel approaches to combine different losses built on top of a shared deep network. The proposed ensemble of losses enforces the model to extract compatible features with all losses. Since the selected losses are diverse and emphasize different aspects of an optimal embedding, our effective combining method yields a considerable improvement over any individual loss and generalize well on unseen classes. It can optimize each loss function and its weight without imposing an additional hyper-parameter. We evaluate our methods on some popular datasets in a Zero-Shot-Learning setting. The results are very encouraging and show that our methods outperform all baseline losses by a large margin in all datasets. Specifically, the proposed method surpasses the best individual loss on the Cars-196 dataset by 10.37% and 9.54% in terms of Recall@1 and kNN accuracy respectively. Moreover, we develop a novel distance-based compression method that compresses the coefficient and embedding of losses into a single embedding vector. The size of the resulting embedding is identical to each baseline learner. Thus, it is fast as each baseline DML in the evaluation stage. Meanwhile, it outperforms the best individual loss on the Cars-196 dataset by 8.28% and 7.76% in terms of Recall@1 and kNN accuracy respectively.
更多查看译文
关键词
Deep metric learning,Semantic embedding,Similarity embedding,Ensemble of Loss function,Combining Losses,Zero Shot Learning
AI 理解论文
溯源树
样例
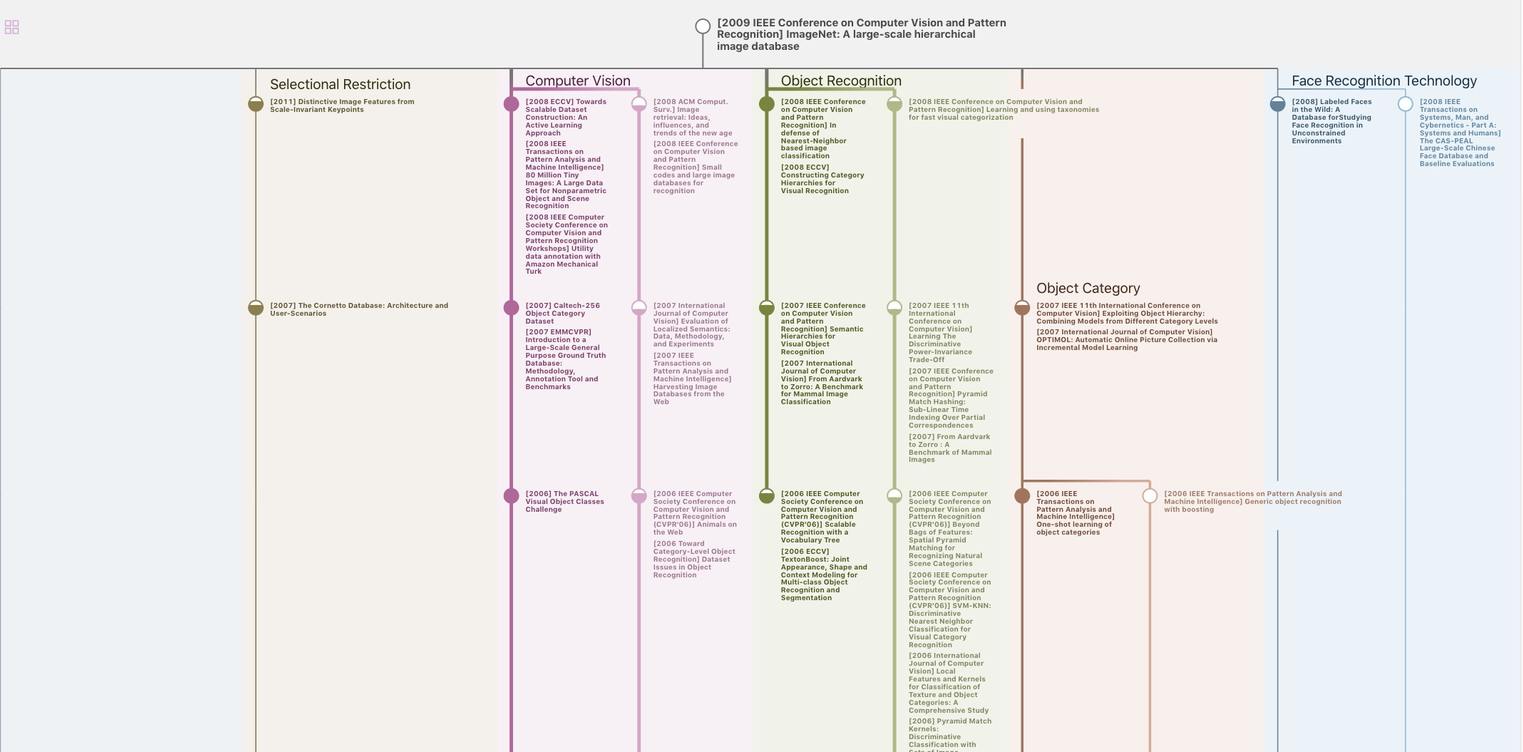
生成溯源树,研究论文发展脉络
Chat Paper
正在生成论文摘要