ClueReader: Heterogeneous Graph Attention Network for Multi-Hop Machine Reading Comprehension
arxiv(2023)
摘要
Multi-hop machine reading comprehension is a challenging task in natural language processing as it requires more reasoning ability across multiple documents. Spectral models based on graph convolutional networks have shown good inferring abilities and lead to competitive results. However, the analysis and reasoning of some are inconsistent with those of humans. Inspired by the concept of grandmother cells in cognitive neuroscience, we propose a heterogeneous graph attention network model named ClueReader to imitate the grandmother cell concept. The model is designed to assemble the semantic features in multi-level representations and automatically concentrate or alleviate information for reasoning through the attention mechanism. The name ClueReader is a metaphor for the pattern of the model: it regards the subjects of queries as the starting points of clues, takes the reasoning entities as bridge points, considers the latent candidate entities as grandmother cells, and the clues end up in candidate entities. The proposed model enables the visualization of the reasoning graph, making it possible to analyze the importance of edges connecting entities and the selectivity in the mention and candidate nodes, which is easier to comprehend empirically. Evaluations on the open-domain multi-hop reading dataset WikiHop and drug-drug interaction dataset MedHop proved the validity of ClueReader and showed the feasibility of its application of the model in the molecular biology domain.
更多查看译文
关键词
machine reading comprehension, knowledge graph, graph neural networks, attention mechanism
AI 理解论文
溯源树
样例
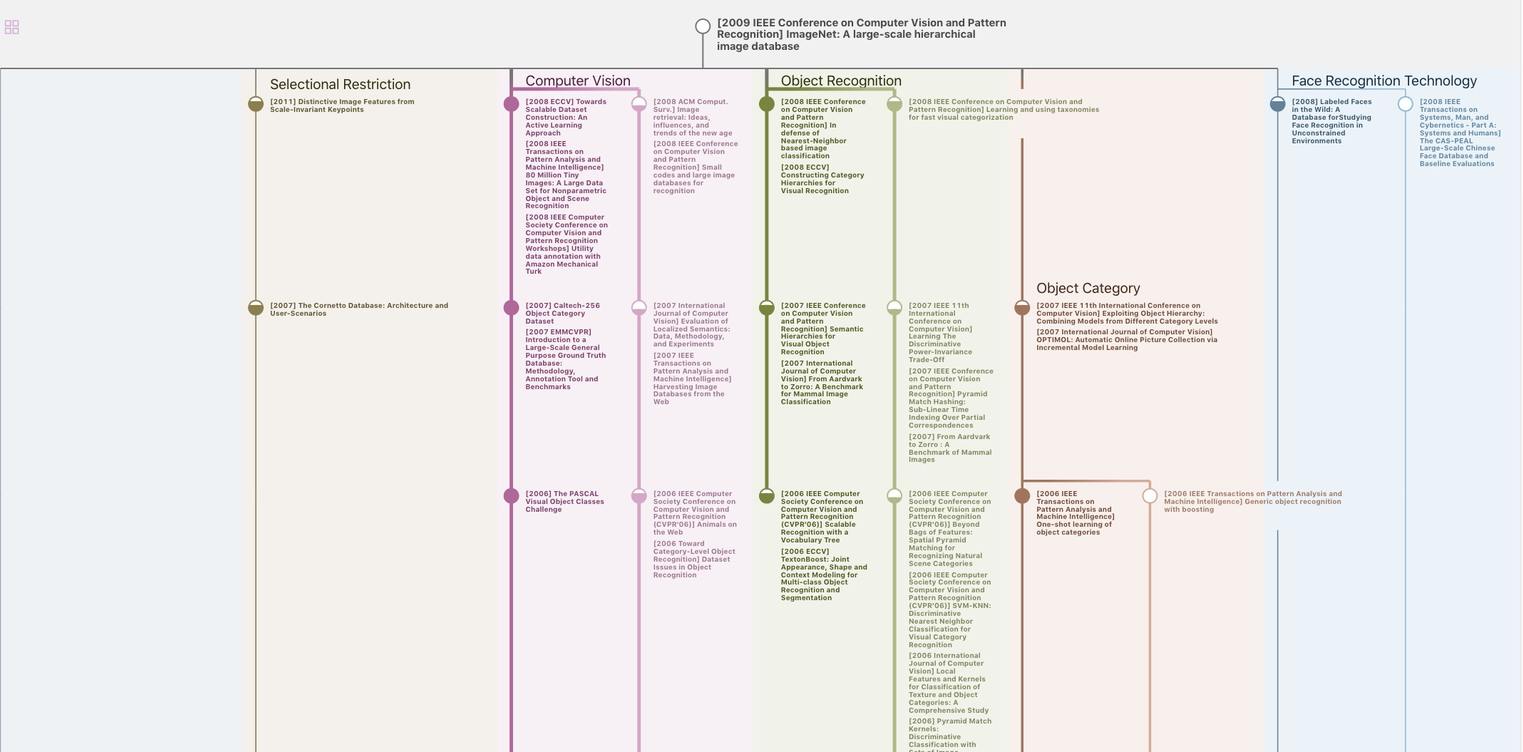
生成溯源树,研究论文发展脉络
Chat Paper
正在生成论文摘要