Cs-Capsfpn: A Context-Augmentation And Self-Attention Capsule Feature Pyramid Network For Road Network Extraction From Remote Sensing Imagery
CANADIAN JOURNAL OF REMOTE SENSING(2021)
摘要
The information-accurate road network database is greatly significant and provides essential input to many transportation-related activities. Recently, remote sensing images have been an important data source for assisting rapid road network updating tasks. However, due to the diverse challenging scenarios of roads in remote sensing images, such as occlusions, shadows, material diversities, and topology variations, it is still difficult to realize highly accurate extraction of roads. This paper proposes a novel context-augmentation and self-attention capsule feature pyramid network (CS-CapsFPN) to extract roads from remote sensing images. By designing a capsule feature pyramid network architecture, the proposed CS-CapsFPN can extract and fuze different-level and different-scale high-order capsule features to provide a high-resolution and semantically strong feature representation for predicting the road region maps. By integrating the context-augmentation and self-attention modules, the proposed CS-CapsFPN can exploit multi-scale contextual properties at a high-resolution perspective and emphasize channel-wise informative features to further enhance the feature representation robustness. Quantitative evaluations on two test datasets show that the proposed CS-CapsFPN achieves a competitive performance with a precision, recall, intersection-over-union, and F-score of 0.9470, 0.9407, 0.8957, and 0.9438, respectively. Comparative studies also confirm the feasibility and superiority of the proposed CS-CapsFPN in road extraction tasks.
更多查看译文
AI 理解论文
溯源树
样例
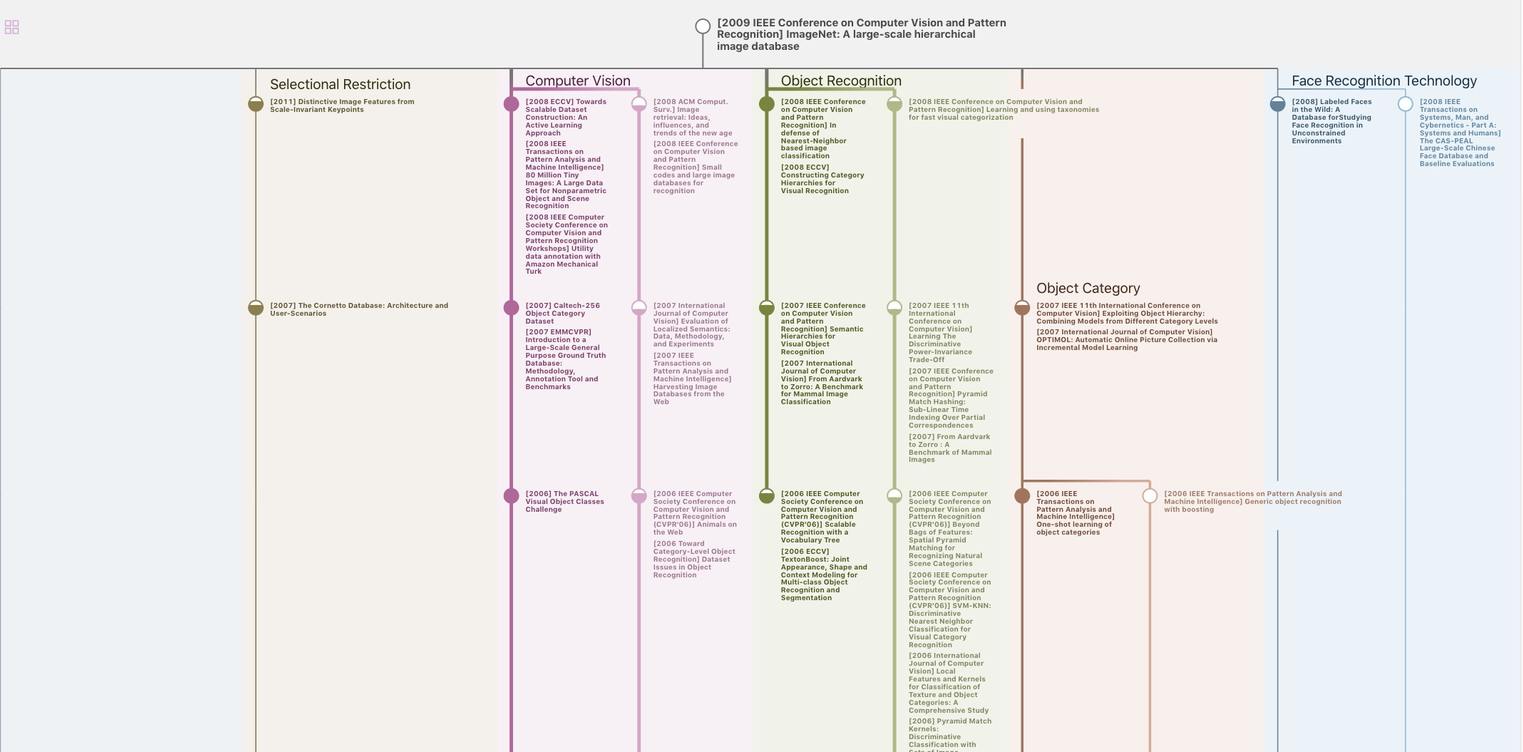
生成溯源树,研究论文发展脉络
Chat Paper
正在生成论文摘要