Machine learning based major complication prediction for patients undergoing transvenous lead extraction
Europace(2021)
摘要
Abstract Funding Acknowledgements Type of funding sources: None. Background Relative benefits of transvenous lead extraction (TLE) should be weighed against the risks on an individual basis. Observational studies have identified disparate risk factors for major adverse events (MAEs), however there are few available tools to aid the clinician risk-stratifying patients. Purpose The purpose of this study was to develop a machine learning (ML)-based risk stratification system to predict risk of MAEs following transvenous lead extraction (TLE). Methods Multiple ML models were derived from a training cohort of 3555 patients undergoing TLE to pre-procedurally identify patients at high and low risk of major adverse events (procedure-related major complication, including procedure-related death). The ML models were then tested on an independent, reference centre cohort of 1171 patients undergoing TLE. Clinical features were selected based on whether they were available in both datasets without large gaps. For model selection we compared the ML algorithms logistic regression, ridge regression, support vector machines (SVM), gradient boosting classifier and random forest. The best performing of these was then compared to a self-normalising network (SNN). Results In total 3122 cases with the required features were included from the training cohort with 53 MAEs (1.7%), and 998 cases in the test cohort with 24 MAEs (2.4%). SVM identified lead dwell time, left ventricular ejection fraction (LVEF), estimated glomerular filtration rate (eGFR), chronic respiratory disease, local infection, heart failure, sepsis and male gender as predictors of MAEs in order of decreasing importance (see figure). For the training data, the SVM provided a more sensitive result compared with SNN (0.83 vs 0.71) at the cost of specificity (0.63 vs 0.79). For the test data, the SVM identified MAEs in 12 out of 123 (9.8%) "high risk" patients, 10 out of 552 (1.8%) "medium risk" patients, and 2 out of 323 (0.006%) "low risk" patients. Conclusion Machine learning provided good discriminative capabilities for identifying patients in a "high risk" and "low risk" category for MAEs. Abstract Figure. 8 most important predictors of MAEs
更多查看译文
AI 理解论文
溯源树
样例
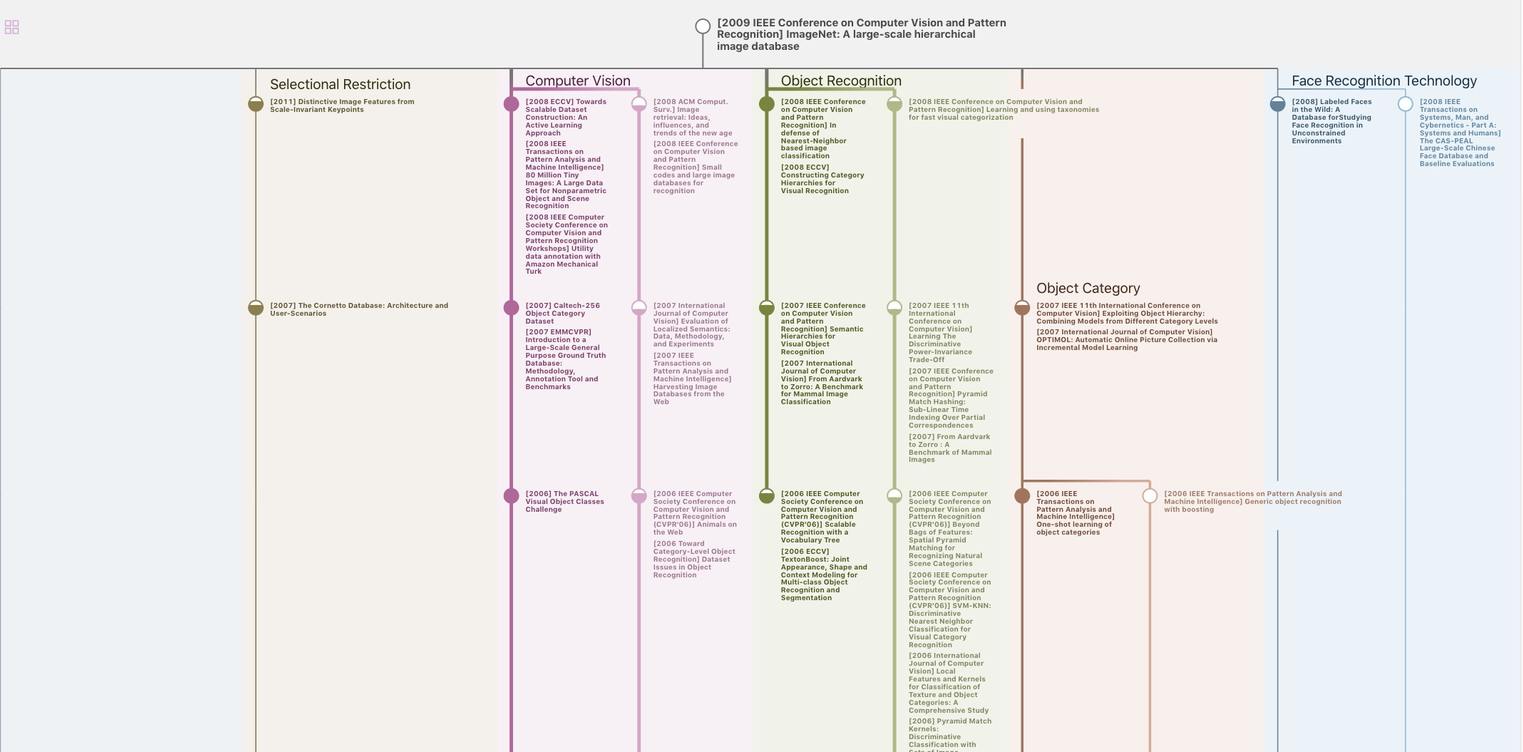
生成溯源树,研究论文发展脉络
Chat Paper
正在生成论文摘要