A Classification Framework of Identifying Major Documents With Search Engine Suggestions and Unsupervised Subtopic Clustering
INTERNATIONAL JOURNAL OF COGNITIVE INFORMATICS AND NATURAL INTELLIGENCE(2021)
摘要
This paper addresses the problem of automatic recognition of out-of-topic documents from a small set of similar documents that are expected to be on some common topic. The objective is to remove documents of noise from a set. A topic model-based classification framework is proposed for the task of discovering out-of-topic documents. This paper introduces a new concept of annotated {\ it search engine suggests}, where this paper takes whichever search queries were used to search for a page as representations of content in that page. This paper adopted word embedding to create distributed representation of words and documents and perform similarity comparison on search engine suggestions. It is shown that search engine suggestions can be highly accurate semantic representations of textual content and demonstrate that the document analysis algorithm using such representation for relevance measure gives satisfactory performance in terms of in-topic content filtering compared to the baseline technique of topic probability ranking.
更多查看译文
关键词
Document Processing, Embedding, Search Engine Suggests, Subtopic, Text Mining, Topic Model, Unsupervised Learning, Word2vec
AI 理解论文
溯源树
样例
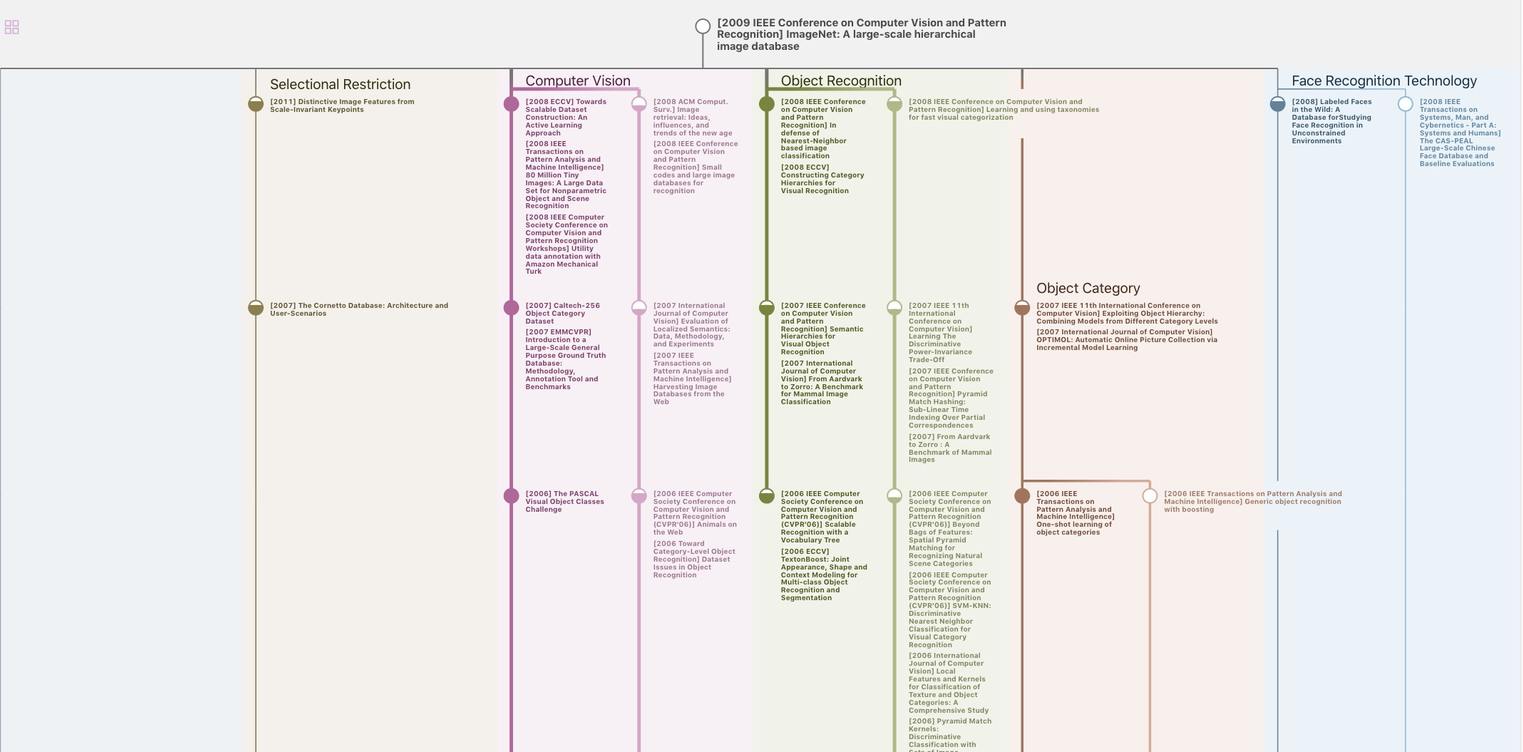
生成溯源树,研究论文发展脉络
Chat Paper
正在生成论文摘要