Towards An Unsupervised Feature Selection Method For Effective Dynamic Features
IEEE ACCESS(2021)
摘要
Dynamic features applications present new obstacles for the selection of streaming features. The dynamic features applications have various characteristics: a) features are processed sequentially while the number of instances is fixed; and b) the feature space does not exist in advance. For example, in a text classification task for spam detection, new features (e.g. words) are dynamically generated and therefore need to be mined to filter out the spams rather than waiting for all features to be collected in order to do so. Traditional feature selection methods, which are not designed for streaming features applications, cannot be used in such an environment, as they require the full feature space in advance in order to statistically determine the representative features. Existing methods that address feature selection in dynamic features applications require the class labels in order to select the representative features. However, most of the real-life data is unlabeled and it is costly to apply manual labeling. In this paper, an efficient unsupervised features selection method is proposed for streaming features applications where the number of features increases while the number of instances remains fixed. In particular, unsupervised Feature Selection for Dynamic Features (UFSSF) is developed to determine the representative streaming features without requiring prior knowledge about data class labels or representative features. The UFSSF extends the k-mean clustering to cumulatively determine whether the newly-arrived feature can be selected as a representative streaming feature, or discarded. Experimental results show significant accuracy results and efficient execution time compared to those of other benchmark methods.
更多查看译文
关键词
Feature selection, streaming features, unsupervised learning
AI 理解论文
溯源树
样例
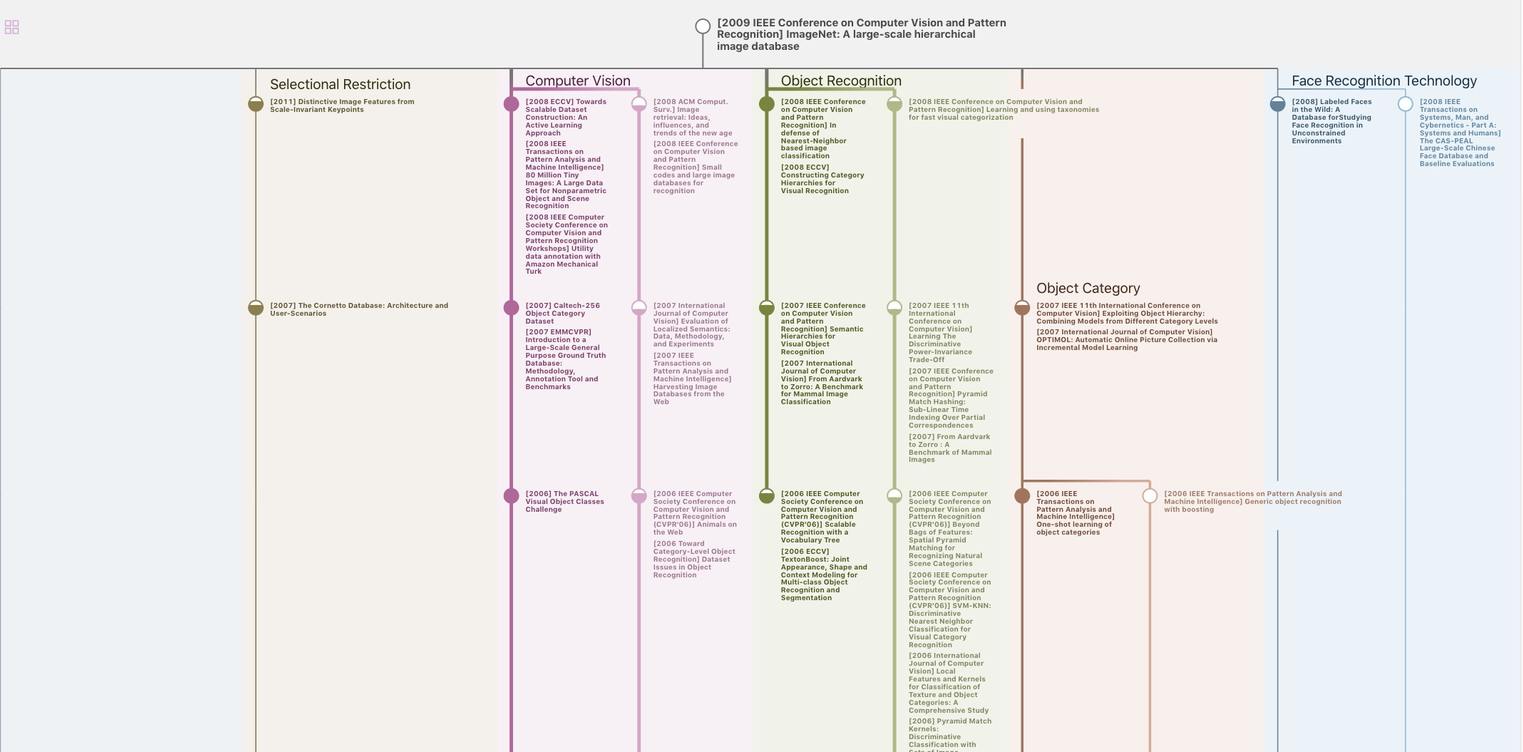
生成溯源树,研究论文发展脉络
Chat Paper
正在生成论文摘要