Da-Roadnet: A Dual-Attention Network For Road Extraction From High Resolution Satellite Imagery
IEEE JOURNAL OF SELECTED TOPICS IN APPLIED EARTH OBSERVATIONS AND REMOTE SENSING(2021)
摘要
Recent advances in deep-learning methods have shown extraordinary performance in road extraction from high resolution satellite imagery. However, most existing deep-learning network models yield discontinuous and incomplete results because of shadows and occlusions. To address this problem, a dual-attention road extraction network (DA-RoadNet) with a certain semantic reasoning ability is proposed. First, DA-RoadNet is designed based on a shallow encoder-to-decoder network with densely connected blocks, which can minimize the loss of road structure information caused by multiple down-sampling operations. Moreover, by constructing a novel attention mechanism module, the proposed network is able to explore and integrate the invisible correlations among road features with their global dependency in spatial and channel dimension respectively. Finally, considering that the proportion of road samples is small in the satellite imagery, a hybrid loss function is appended to handle class imbalance, which enables the network model to train stablely and avoid local optimum. The validation experiments using two open road datasets demonstrate that the proposed DA-RoadNet can effectively solve discontinuous problems and preserve integrity of the extracted roads, thus resulting in a higher accuracy of road extraction compared with other developed state-of-the-arts. The considerable performance on the two challenging benchmarks also proves the powerful generation ability of our method.
更多查看译文
关键词
Roads, Feature extraction, Data mining, Satellites, Image resolution, Image segmentation, Semantics, Attention mechanism, deep learning, high resolution satellite imagery, road extraction
AI 理解论文
溯源树
样例
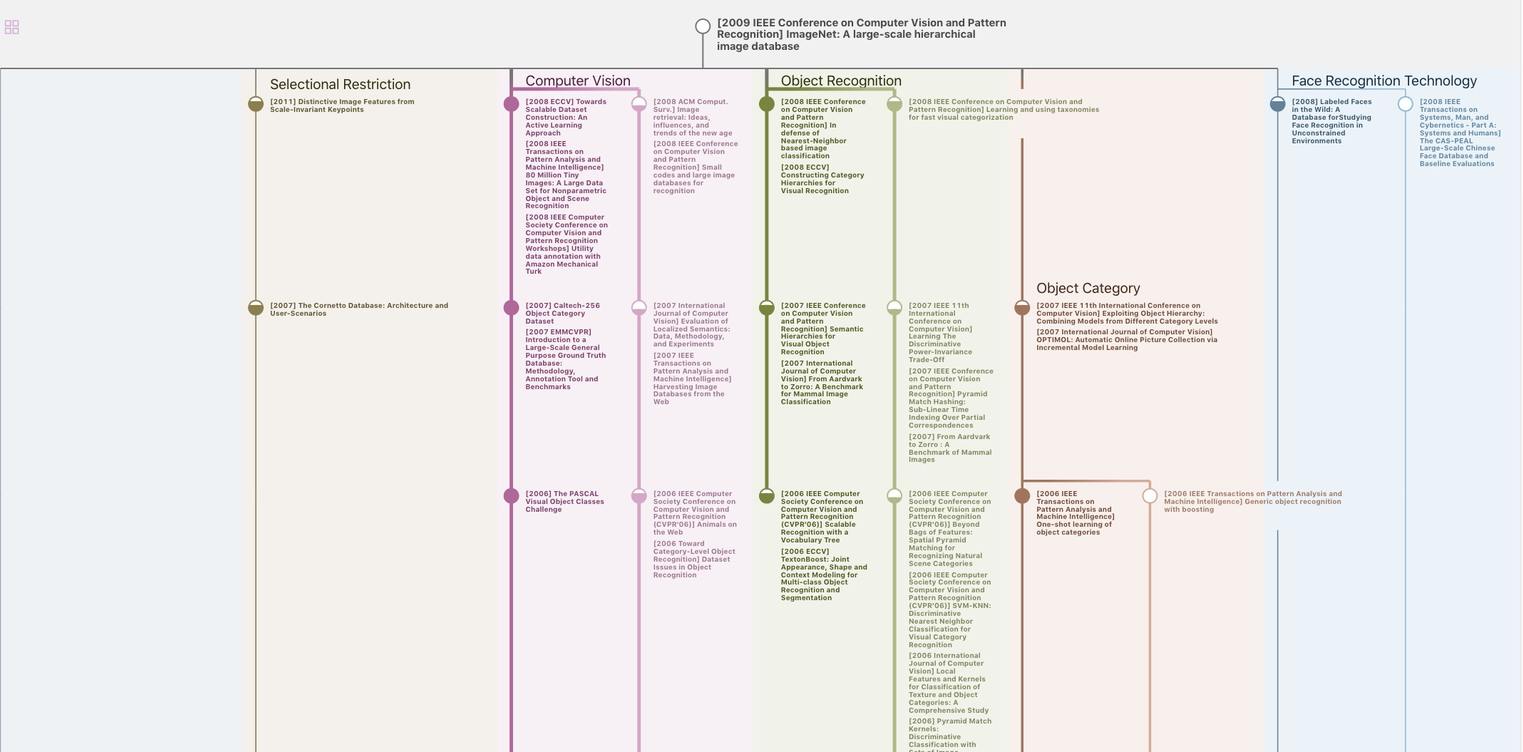
生成溯源树,研究论文发展脉络
Chat Paper
正在生成论文摘要