Inferring Hierarchical Mixture Structures: A Bayesian Nonparametric Approach
ADVANCES IN KNOWLEDGE DISCOVERY AND DATA MINING, PAKDD 2021, PT III(2021)
摘要
We present a Bayesian Nonparametric model for Hierarchical Clustering (HC). Such a model has two main components. The first component is the random walk process from parent to child in the hierarchy and we apply nested Chinese Restaurant Process (nCRP). Then, the second part is the diffusion process from parent to child where we employ Hierarchical Dirichlet Process Mixture Model (HDPMM). This is different from the common choice which is Gaussian-to-Gaussian. We demonstrate the properties of the model and propose a Markov Chain Monte Carlo procedure with elegantly analytical updating steps for inferring the model variables. Experiments on the real-world datasets show that our method obtains reasonable hierarchies and remarkable empirical results according to some well known metrics.
更多查看译文
关键词
hierarchical mixture structures,bayesian
AI 理解论文
溯源树
样例
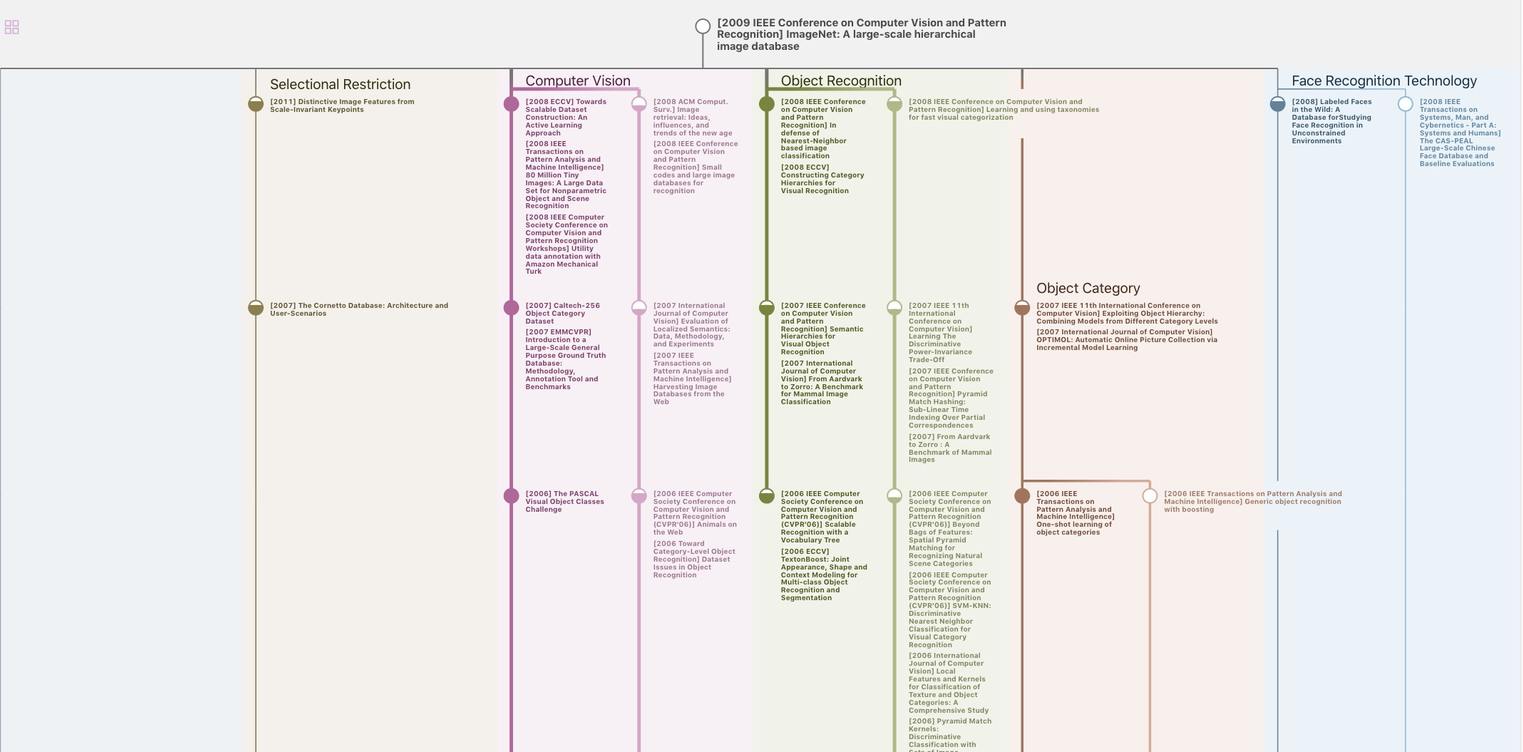
生成溯源树,研究论文发展脉络
Chat Paper
正在生成论文摘要