Solving distribution problems in content-based recommendation system with gaussian mixture model
APPLIED INTELLIGENCE(2021)
摘要
Recommendation systems play an important role in boosting purchasing consumption for many manufacturers by helping consumers find the most appropriate items. Furthermore, there are quite range of recommendation algorithms so far that can be efficient; however, a content-based algorithm is always the most popular, powerful, and productive method taken at the begin time of any project. In the negative aspect, somehow the accuracy of content-based algorithm results is still a concern that correlates to probabilistic similarity. In addition, the similarity calculation method is another crucial that affect the accuracy of content-based recommendation in probabilistic problems. In order to solve these problems, we propose a new content-based recommendation based on the Gaussian Mixture Model to improve the accuracy with more sensitive results for probabilistic recommendation problems. Our proposed method is experimented on a liquor dataset including six main flavour tastes, liquor main taste tags, and some other criteria. The method clusters n liquor records relied on n vectors of six dimensions into k group ( k < n ) before applying a formula to sort the results. Compared our proposed algorithm with three other popular models on the above dataset, the accuracy of the experimental results not only outweighs the comparison to those of three other models but also attain a very speedy response time in real-life applications.
更多查看译文
关键词
Recommendation system, Content-based, Gaussian Mixture Model (GMM), Distribution recommendation
AI 理解论文
溯源树
样例
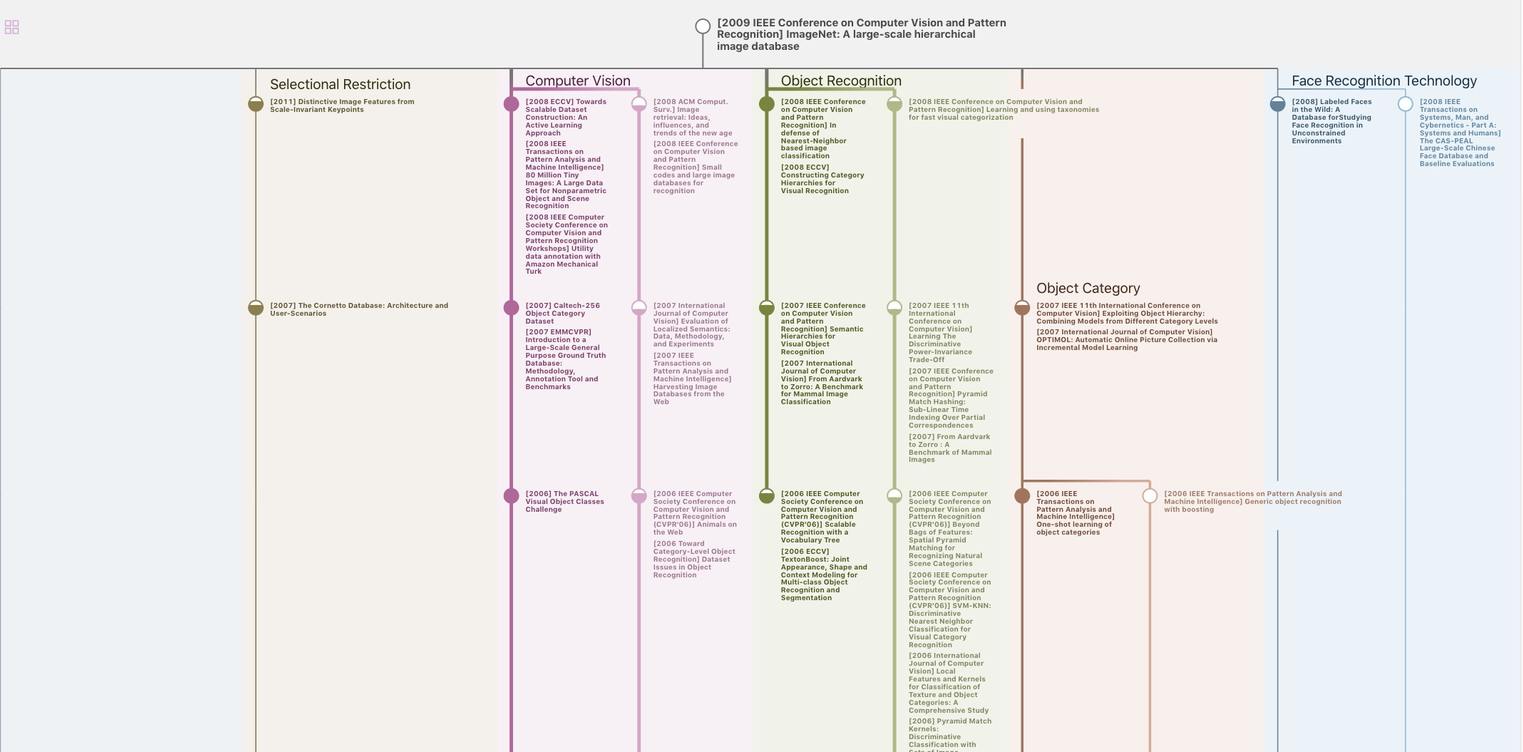
生成溯源树,研究论文发展脉络
Chat Paper
正在生成论文摘要