SenCS: Enabling Real-time Indoor Proximity Verification via Contextual Similarity
ACM Transactions on Sensor Networks(2021)
摘要
Editorial NotesThe authors have requested minor, non-substantive changes to the VoR and, in accordance with ACM policies, a Corrected VoR was published on September 28, 2021. For reference purposes the VoR may still be accessed via the Supplemental Material section on this page.AbstractIndoor proximity verification has become an increasingly useful primitive for the scenarios where access is granted to the previously unknown users when they enter a given area (e.g., a hotel room). Existing solutions either rely on homogeneous sensing modalities shared by two parties or require additional human interactions. In this article, we propose a context-based indoor proximity verification scheme, called SenCS, to enable real-time autonomous access for mobile devices, utilizing the available heterogeneous sensors at the user side and at the room side. The intuition is that only when the user is within a room can sensors from both sides observe the same events in the room. Yet such a solution is challenging, because the events may not provide enough entropy within the required time and the heterogeneity in sensing modalities may not always agree on the sensed events. To overcome the challenges, we exploit the time intervals between successively human actions to create heterogeneous contextual fingerprints (HCF) at a millisecond level. By comparing the contextual similarity between the HCF s from both the room and user sides, SenCS accomplishes the indoor proximity verification. Through proof-of-concept implementation and evaluations on 30 participants, SenCS achieves an accuracy of 99.77% and an equal error rate (EER) of 0.23% across various hardware configurations.
更多查看译文
关键词
Indoor proximity verification, real-time, heterogeneous sensor, contextual similarity
AI 理解论文
溯源树
样例
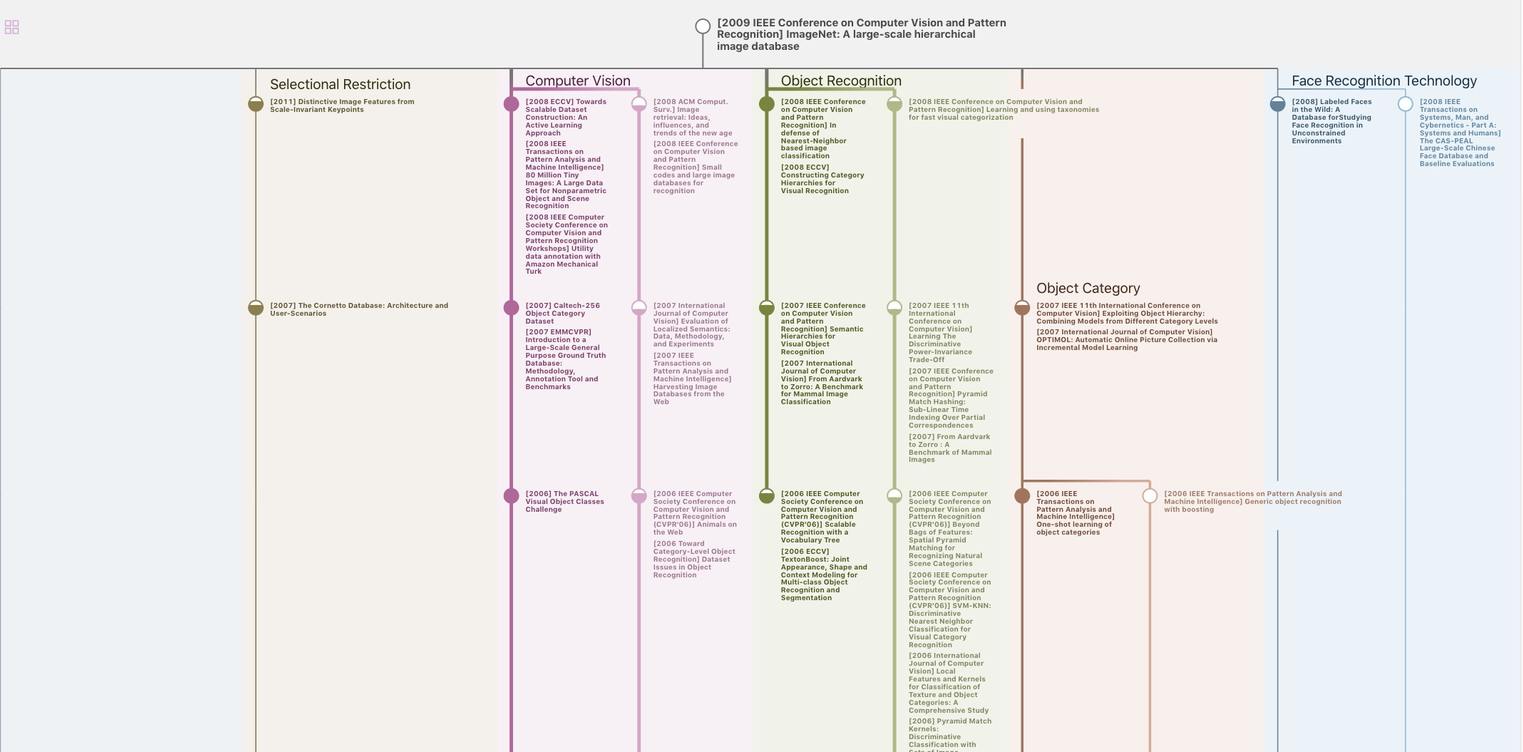
生成溯源树,研究论文发展脉络
Chat Paper
正在生成论文摘要