Robust Central Difference Kalman Filter With Mixture Correntropy: A Case Study For Integrated Navigation
IEEE ACCESS(2021)
摘要
In this paper, a robust central difference Kalman filter is proposed to address the process uncertainty and non-Gaussian measurement noise induced by the vehicle's severe maneuver and abnormal measurements in MEMS-SINS/GNSS integrated navigation system. Compared with the state-of-the-art noise distribution based robust filter, in the proposed filter, the process uncertainty and measurement uncertainty are simultaneously suppressed based on a new constructed cost function, which is independent of noise distribution and more insensitive to the non-Gaussian noise. To be specific, the statistical linearization approach is first presented to derive a linear-like regression model. Then, by resorting to the innovation orthogonal theory and Cholesky triangular decomposition, the fading factor of cost function is adaptively and robustly determined in the process of iteration, where the filtering performance and the stability of the algorithm under the condition of process uncertainty are extremely enhanced. Furthermore, the correntropy using the mixture of two Gaussian functions as the kernel function is incorporated into the cost function to prevent the non-Gaussian measurement noise. Our extensive simulation and car-mounted experiment demonstrate that the proposed filter can achieve higher estimation accuracy and better robustness as compared with the related state-of-the-art methods.
更多查看译文
关键词
MEMS-SINS/GNSS integrated navigation, robust central difference Kalman filter, mixture correntropy
AI 理解论文
溯源树
样例
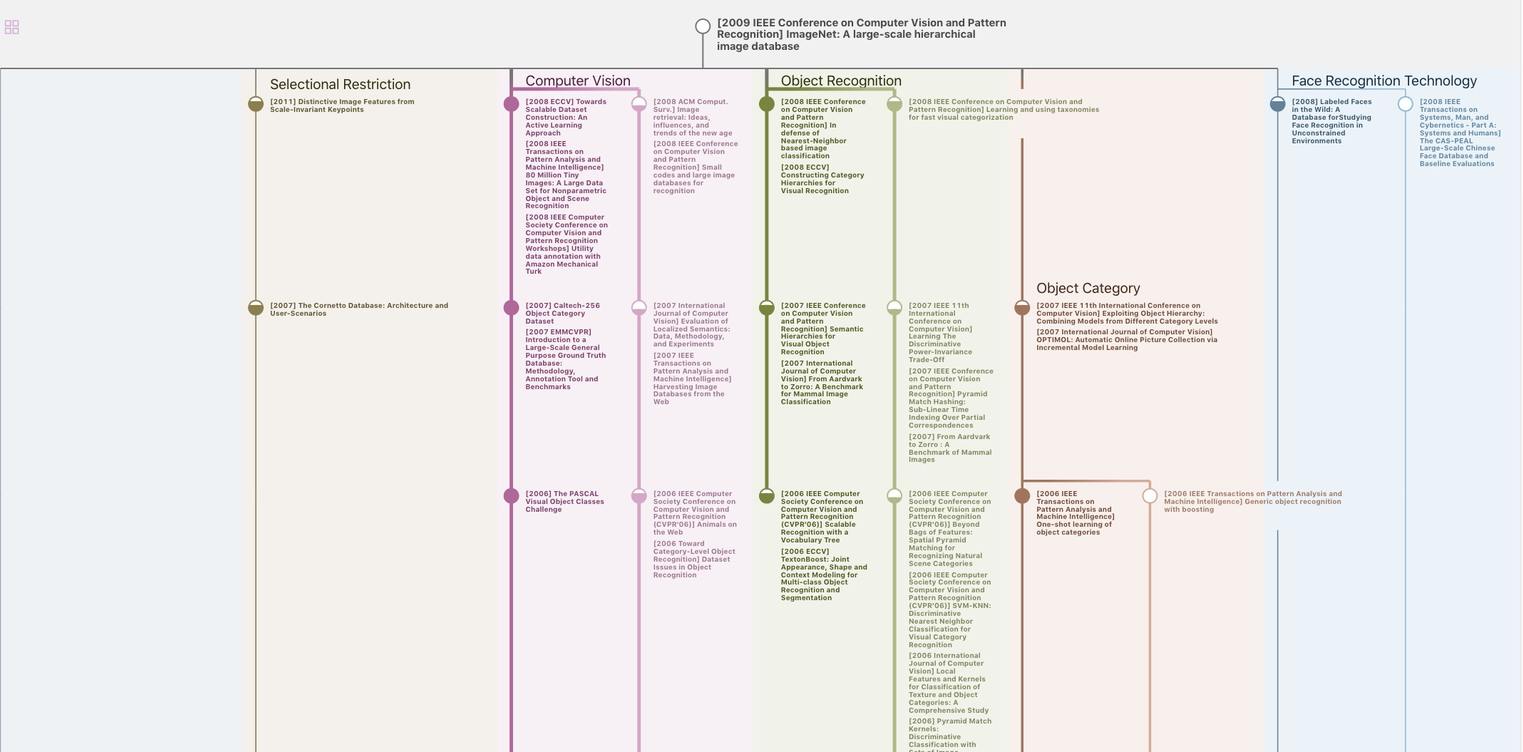
生成溯源树,研究论文发展脉络
Chat Paper
正在生成论文摘要