Single image super resolution based on multi-scale structure and non-local smoothing
EURASIP JOURNAL ON IMAGE AND VIDEO PROCESSING(2021)
摘要
In this paper, we propose a hybrid super-resolution method by combining global and local dictionary training in the sparse domain. In order to present and differentiate the feature mapping in different scales, a global dictionary set is trained in multiple structure scales, and a non-linear function is used to choose the appropriate dictionary to initially reconstruct the HR image. In addition, we introduce the Gaussian blur to the LR images to eliminate a widely used but inappropriate assumption that the low resolution (LR) images are generated by bicubic interpolation from high-resolution (HR) images. In order to deal with Gaussian blur, a local dictionary is generated and iteratively updated by K -means principal component analysis (K-PCA) and gradient decent (GD) to model the blur effect during the down-sampling. Compared with the state-of-the-art SR algorithms, the experimental results reveal that the proposed method can produce sharper boundaries and suppress undesired artifacts with the present of Gaussian blur. It implies that our method could be more effect in real applications and that the HR-LR mapping relation is more complicated than bicubic interpolation.
更多查看译文
关键词
Super-resolution,Sparse representation,Dictionary learning,K-PCA,Non-local mean
AI 理解论文
溯源树
样例
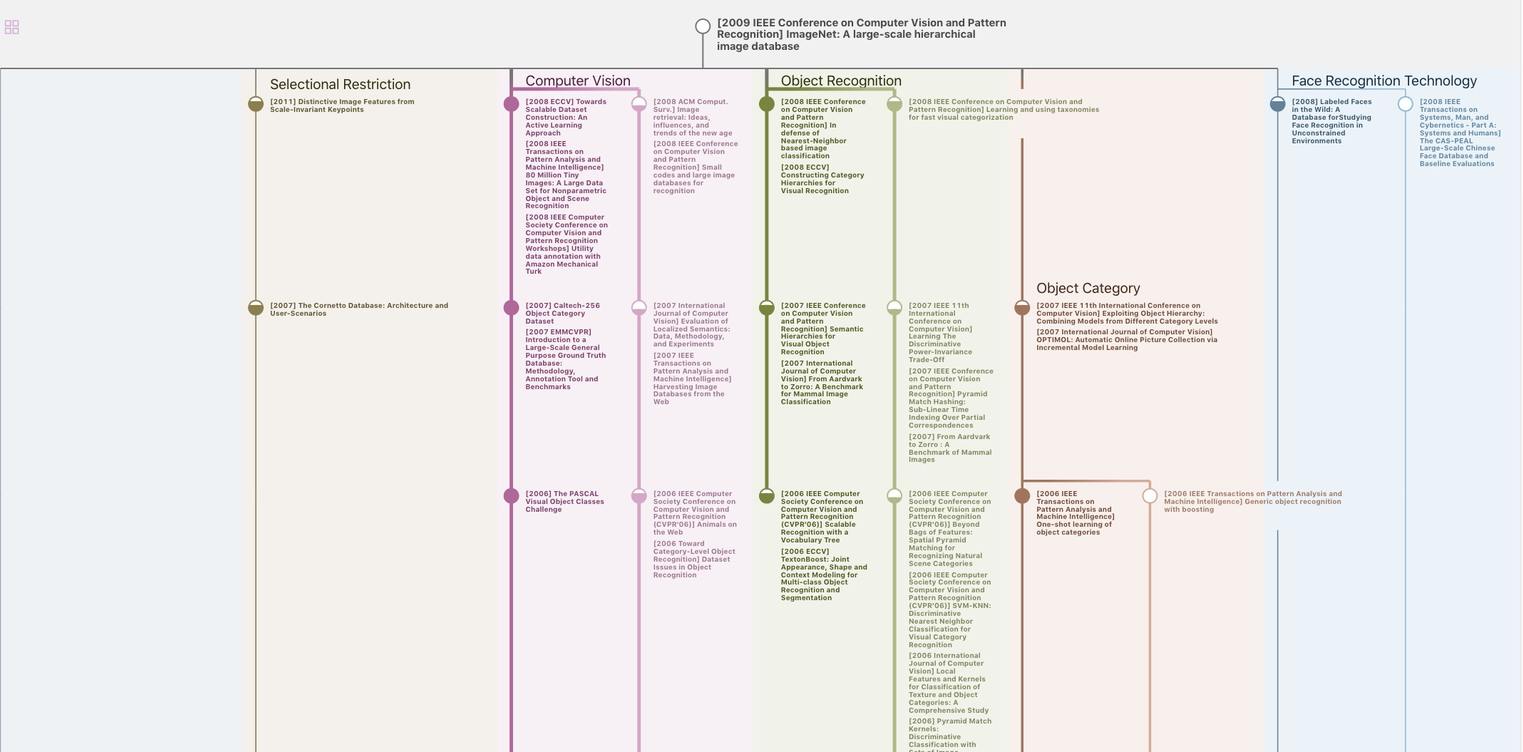
生成溯源树,研究论文发展脉络
Chat Paper
正在生成论文摘要