PRE-TRAINING TRANSFORMER DECODER FOR END-TO-END ASR MODEL WITH UNPAIRED TEXT DATA
2021 IEEE INTERNATIONAL CONFERENCE ON ACOUSTICS, SPEECH AND SIGNAL PROCESSING (ICASSP 2021)(2021)
摘要
This paper presents a method to pre-train transformer-based encoder-decoder automatic speech recognition (ASR) models using sufficient target-domain text. During pre-training, we train the transformer decoder as a conditional language model with empty or artifical states, rather than the real encoder states. By this pre-training strategy, the decoder can learn how to generate grammatical text sequence before learning how to generate correct transcriptions. Contrast to other methods which utilize text only data to improve the ASR performance, our method does not change the network architecture of the ASR model or introduce extra component like text-to-speech (TTS) or text-to-encoder (TTE). Experimental results on LibriSpeech corpus show that the proposed method can relatively reduce the word error rate over 10%, using 960 hours transcriptions.
更多查看译文
关键词
Speech recognition, pre-training, end-to-end, unpaired data, transformer
AI 理解论文
溯源树
样例
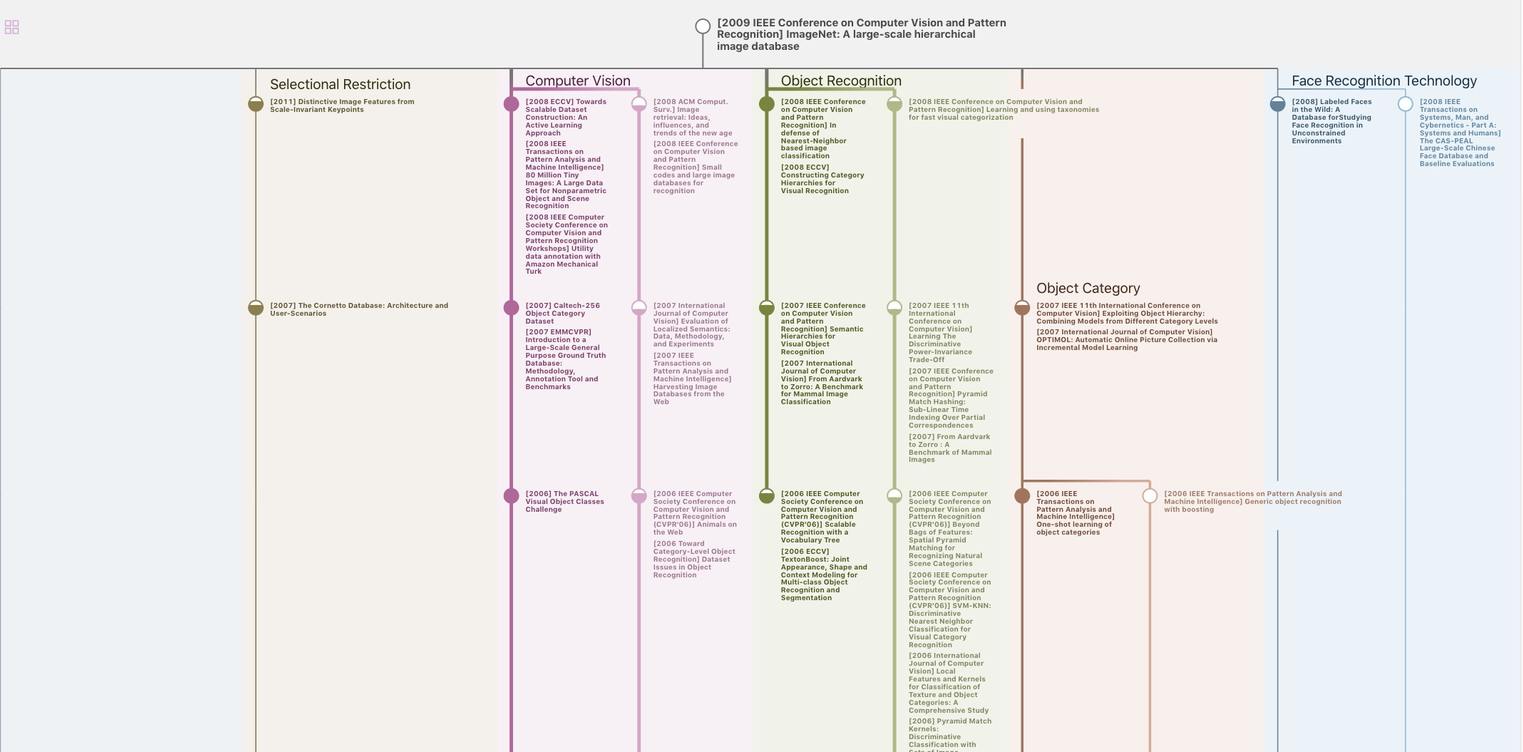
生成溯源树,研究论文发展脉络
Chat Paper
正在生成论文摘要