What executives get wrong about statistics: Moving from statistical significance to effect sizes and practical impact
Business Horizons(2022)
摘要
Statistical significance functions as an arbiter of sorts for data analysis purporting to show a relationship between two or more variables. Unfortunately, in far too many situations, statistical significance may lead decision-makers relying on data and analytics to improve business decisions astray, particularly in the context of big data. In this article, I outline reasons why executives should develop a healthy discernment when they see the phrase “statistically significant” in media outlets, internal analyses, consulting reports, and other sources. To overcome the limitations of focusing on statistical significance, I propose executives shift their attention toward the effect size reported from a statistical model. While not without limitation, effect sizes are more useful to decision-makers, highlight the practical implication of analyses, and help in quantifying the uncertainty inherent to working with data.
更多查看译文
关键词
Statistical significance,Statistical correlation,Data analysis,Omitted variables,P value
AI 理解论文
溯源树
样例
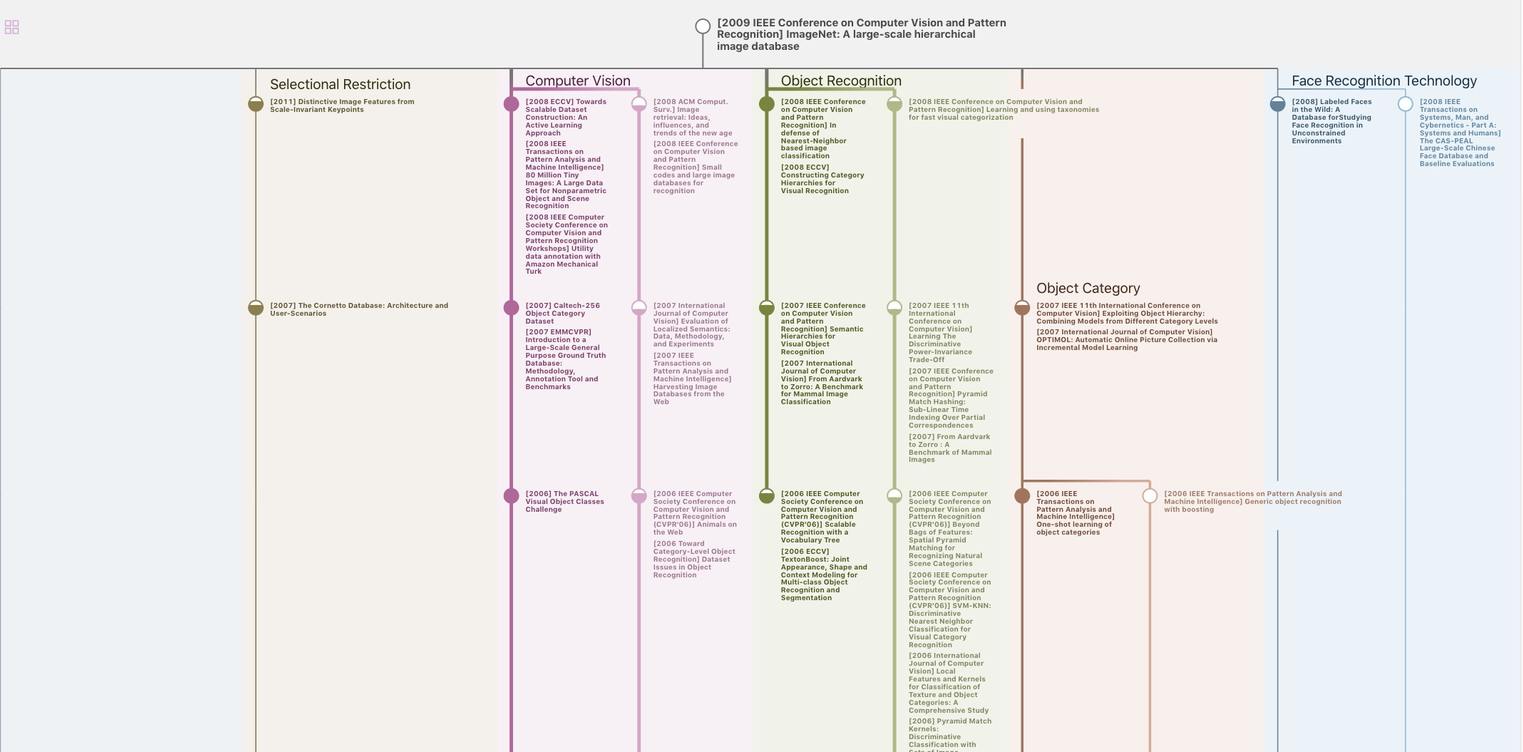
生成溯源树,研究论文发展脉络
Chat Paper
正在生成论文摘要