ENSURE: ENSEMBLE STEIN'S UNBIASED RISK ESTIMATOR FOR UNSUPERVISED LEARNING
2021 IEEE INTERNATIONAL CONFERENCE ON ACOUSTICS, SPEECH AND SIGNAL PROCESSING (ICASSP 2021)(2021)
摘要
Deep learning algorithms are emerging as powerful alternatives to compressed sensing methods, offering improved image quality and computational efficiency. Unfortunately, fully sampled training images may not be available or are difficult to acquire in several applications, including high-resolution and dynamic imaging. Previous studies in image reconstruction have utilized Stein's Unbiased Risk Estimator (SURE) as a mean square error (MSE) estimate for the image denoising step in an unrolled network. Unfortunately, the end-to-end training of a network using SURE remains challenging since the projected SURE loss is a poor approximation to the MSE, especially in the heavily undersampled setting. We propose an ENsemble SURE (ENSURE) approach to train a deep network only from undersampled measurements. In particular, we show that training a network using an ensemble of images, each acquired with a different sampling pattern, can closely approximate the MSE. Our preliminary experimental results show that the proposed ENSURE approach gives comparable reconstruction quality to supervised learning and a recent unsupervised learning method.
更多查看译文
关键词
Unsupervised learning, SURE, parallel MRI
AI 理解论文
溯源树
样例
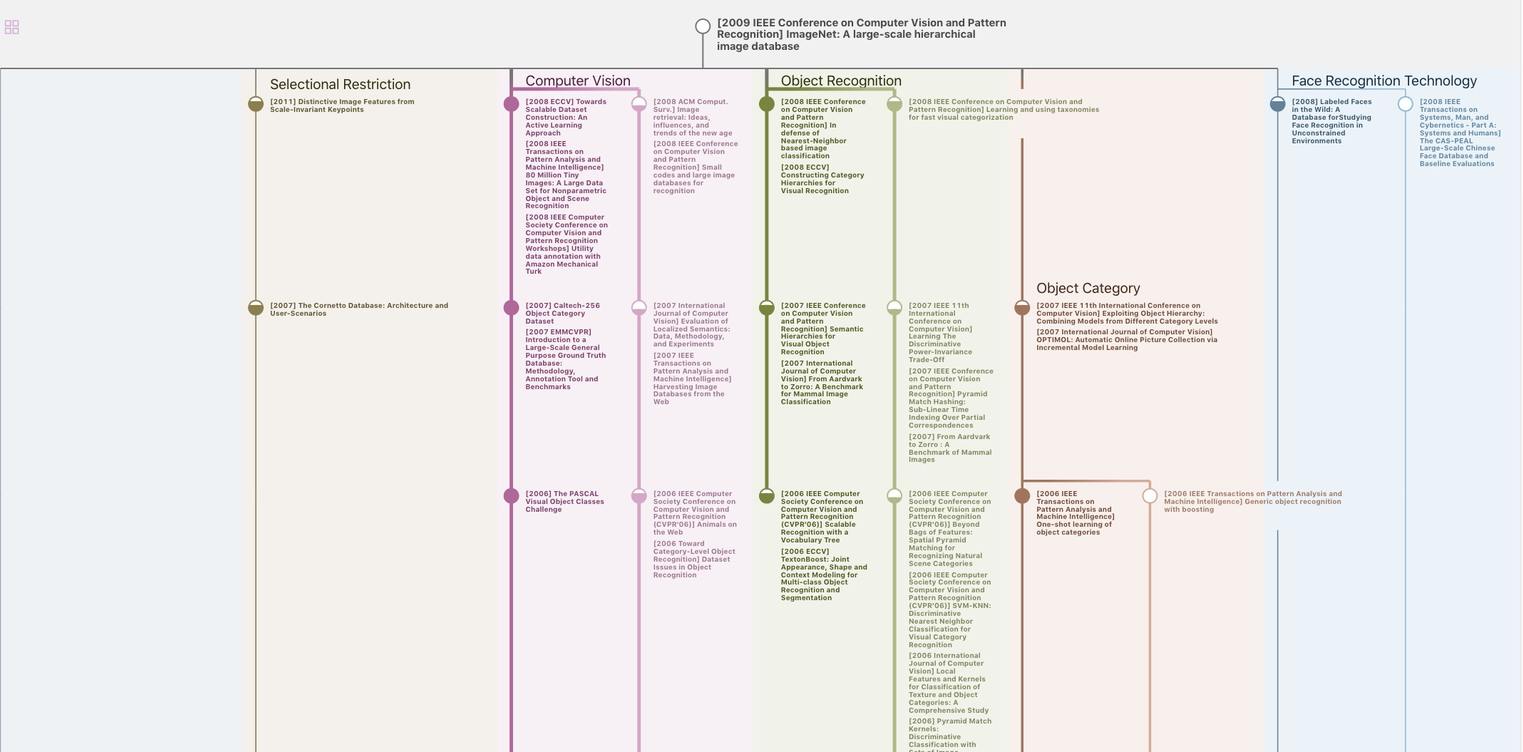
生成溯源树,研究论文发展脉络
Chat Paper
正在生成论文摘要