Fast implementation of partial least squares for function-on-function regression
Journal of Multivariate Analysis(2021)
摘要
People employ the function-on-function regression to model the relationship between two stochastic processes. Fitting this model, widely used strategies include functional partial least squares algorithms which typically require iterative eigen-decomposition. Here we introduce a route of functional partial least squares based upon Krylov subspace. Our route can be expressed in two forms equivalent to each other in exact arithmetic: One is non-iterative with explicit expressions of the estimator and prediction, facilitating the theoretical derivation and potential extensions; the other one stabilizes numerical outputs. The consistency of estimation and prediction is established under regularity conditions. It is highlighted that our proposal is competitive in terms of both estimation and prediction accuracy but consumes much less execution time.
更多查看译文
关键词
primary,secondary
AI 理解论文
溯源树
样例
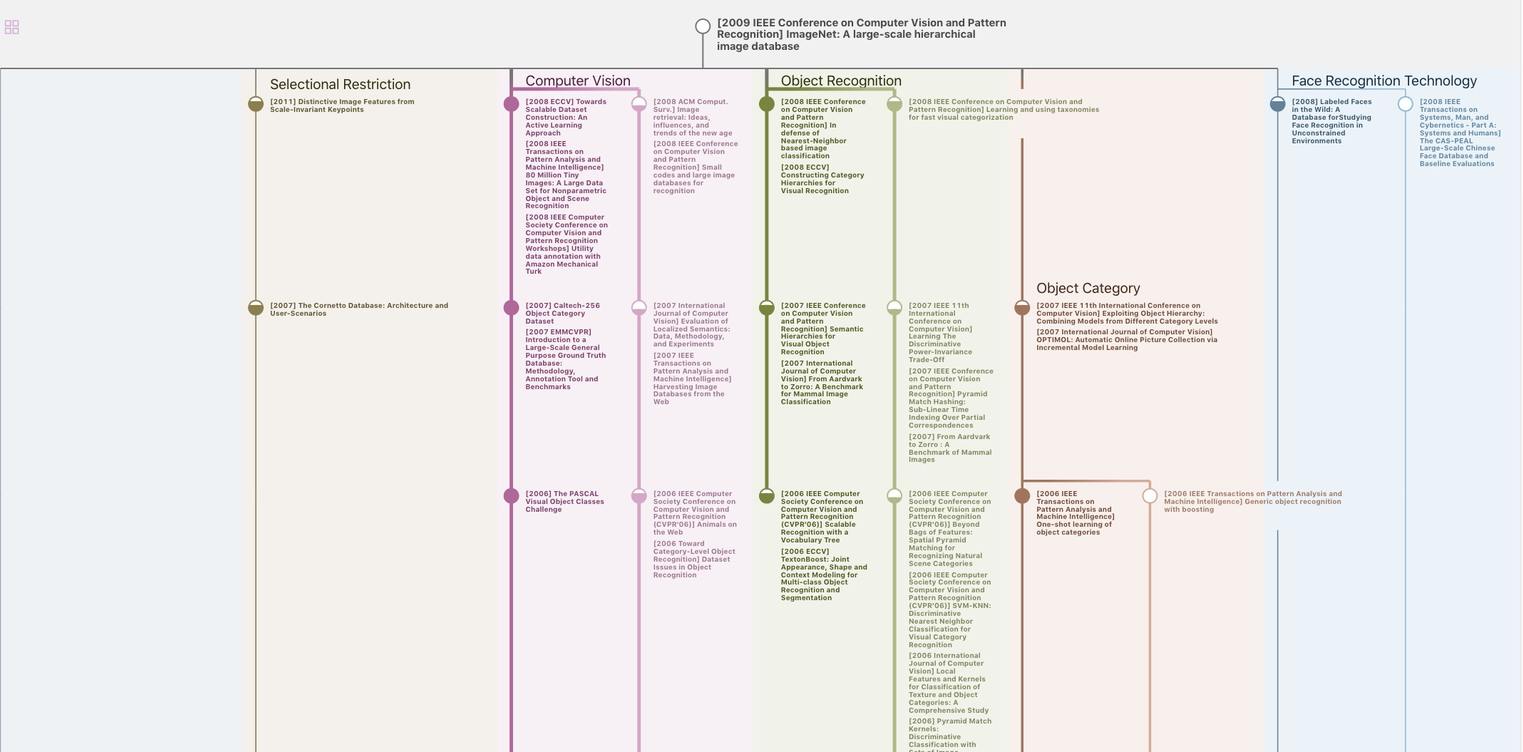
生成溯源树,研究论文发展脉络
Chat Paper
正在生成论文摘要