SYNTHESIZE & LEARN: JOINTLY OPTIMIZING GENERATIVE AND CLASSIFIER NETWORKS FOR IMPROVED DROWSINESS DETECTION
2021 IEEE INTERNATIONAL CONFERENCE ON ACOUSTICS, SPEECH AND SIGNAL PROCESSING (ICASSP 2021)(2021)
摘要
Driving in a state of drowsiness is a major cause of road accidents, resulting in tremendous damage to life and property. Developing robust, automatic, real-time systems that can infer drowsiness states of drivers has the potential of making life-saving impact. However, real-world drowsy driving datasets are unbalanced, due to the sparsity of drowsy driving events. We focus on the problem of alleviating the class imbalance problem by using generative adversarial networks (GAN) to synthesize examples of sparse classes directly in the feature-space. Our GAN-based framework simultaneously generates realistic examples of sparse classes while using the generated samples to improve the performance of a separate drowsiness classifier. We validate this approach in a real-world drowsiness dataset, where we demonstrate a classifier trained using this approach outperforms a stand-alone classifier trained without any GAN-based augmentations.
更多查看译文
关键词
Drowsiness Detection, Facial Expressions, GAN, Feature Synthesis, Joint Optimization
AI 理解论文
溯源树
样例
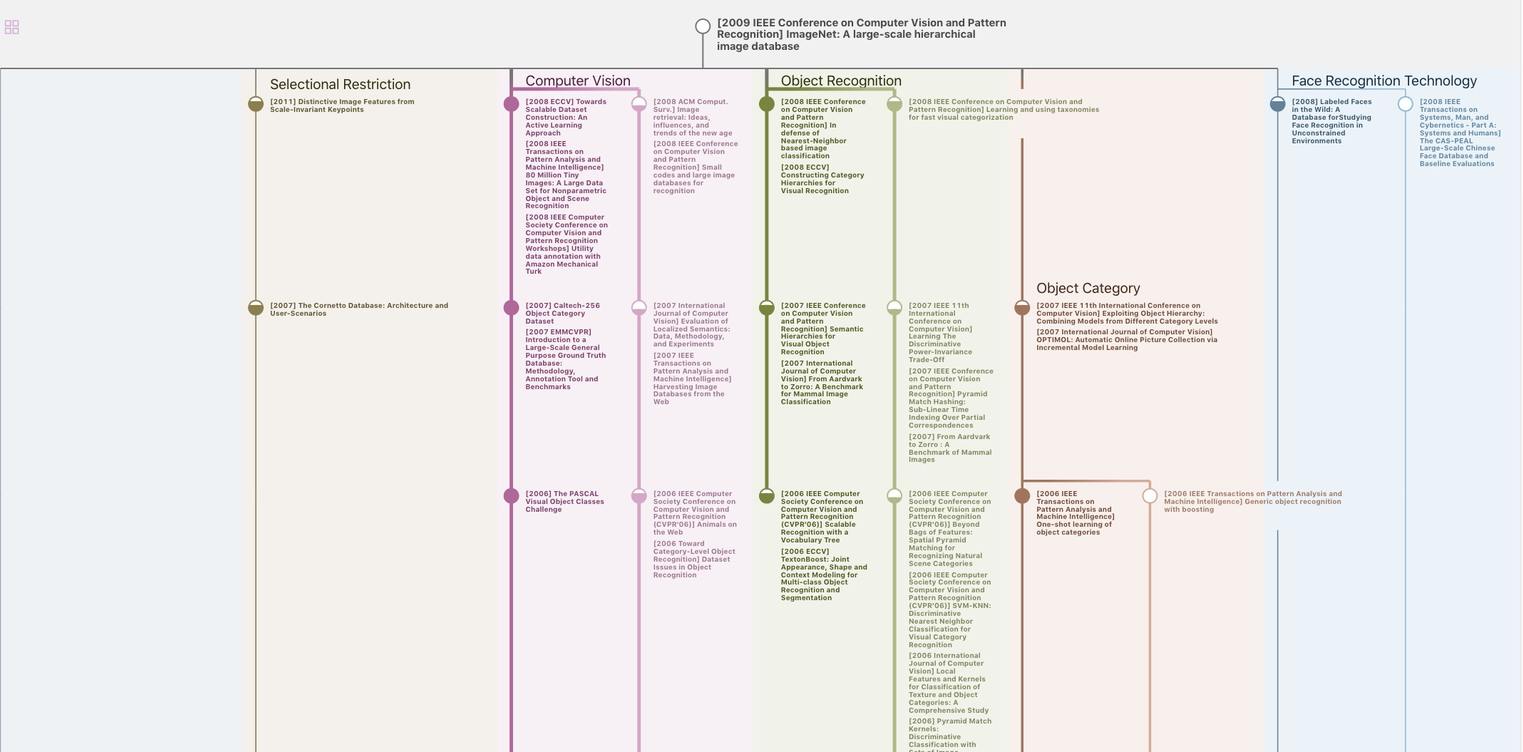
生成溯源树,研究论文发展脉络
Chat Paper
正在生成论文摘要