DECENTRALIZED OPTIMIZATION ON TIME-VARYING DIRECTED GRAPHS UNDER COMMUNICATION CONSTRAINTS
2021 IEEE INTERNATIONAL CONFERENCE ON ACOUSTICS, SPEECH AND SIGNAL PROCESSING (ICASSP 2021)(2021)
摘要
We consider the problem of decentralized optimization where a collection of agents, each having access to a local cost function, communicate over a time-varying directed network and aim to minimize the sum of those functions. In practice, the amount of information that can be exchanged between the agents is limited due to communication constraints. We propose a communication-efficient algorithm for decentralized convex optimization that rely on sparsification of local updates exchanged between neighboring agents in the network. In directed networks, message sparsification alters column-stochasticity - a property that plays an important role in establishing convergence of decentralized learning tasks. We propose a decentralized optimization scheme that relies on local modification of mixing matrices, and show that it achieves O(lnT/root T) convergence rate in the considered settings. Experiments validate theoretical results and demonstrate efficacy of the proposed algorithm.
更多查看译文
关键词
decentralized optimization, convex programming
AI 理解论文
溯源树
样例
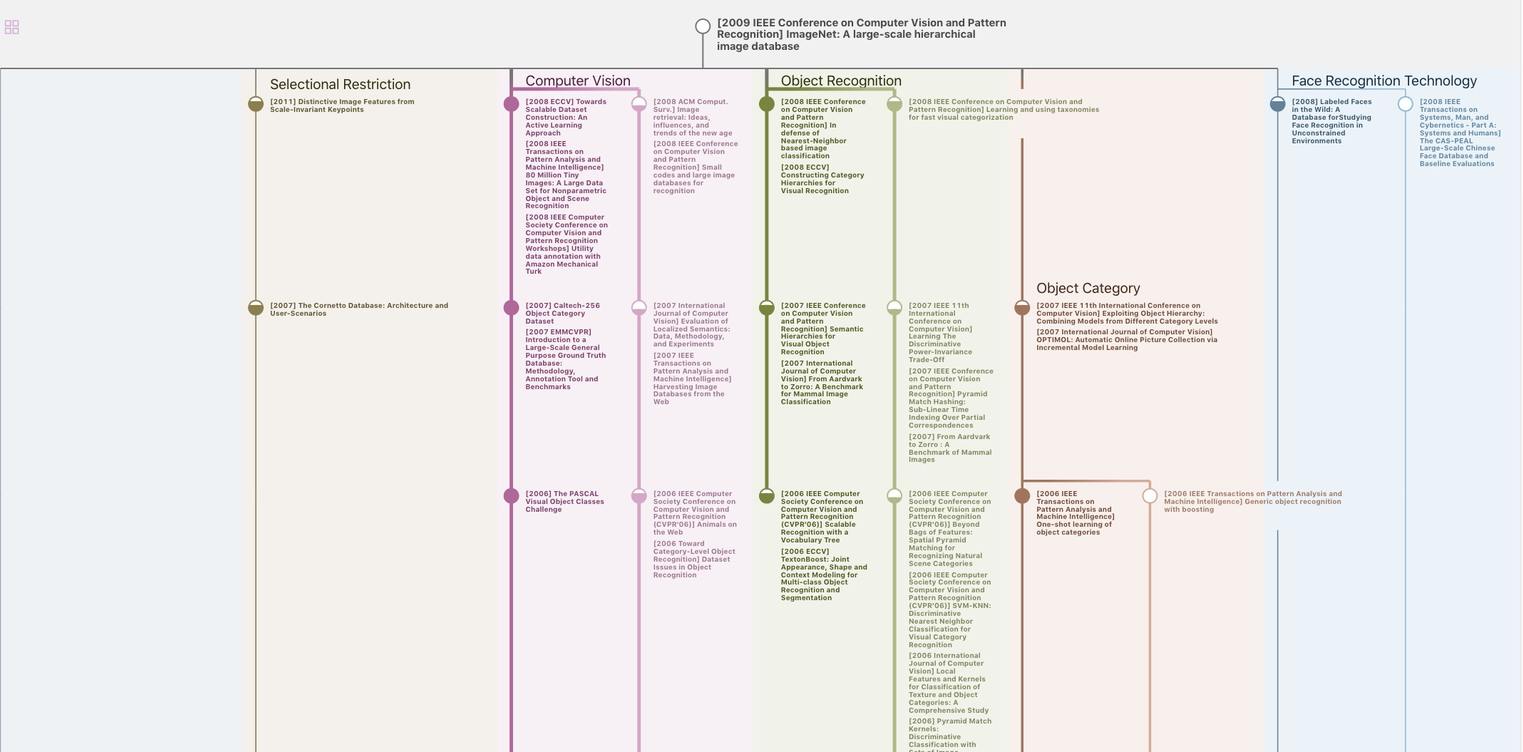
生成溯源树,研究论文发展脉络
Chat Paper
正在生成论文摘要