Dual-Stage Bayesian Sequence to Sequence Embeddings for Energy Demand Forecasting
ADVANCES IN KNOWLEDGE DISCOVERY AND DATA MINING, PAKDD 2021, PT I(2021)
摘要
Bayesian methods provide a robust framework to model uncertainty estimation. However, conventional Bayesian models are hard to tune and often fail to scale over high dimensional data. We propose a novel Dual-Stage Bayesian Sequence to Sequence (DBS2S) model by extending Sequence to Sequence (S2S) deep learning architecture with exogenous variable input to capture uncertainty and forecast energy consumption accurately. DBS2S model is trained with a two-stage S2S encoder-decoder network and benefits from the feature representation capability of S2S to capture complex multimodal posterior distributions within embeddings. We evaluate the proposed model for probabilistic energy consumption forecasting using four real-world public datasets and achieve improved prediction accuracy up to 64% in terms of mean absolute percentage error over existing, state-of-the-art Bayesian neural networks. Additionally, probabilistic prediction intervals forecasted by DBS2S is utilized to detect outliers and flag diverse consumer behavior.
更多查看译文
关键词
Energy demand forecasting, Bayesian neural networks, Prediction uncertainty, Time series, Anomaly detection
AI 理解论文
溯源树
样例
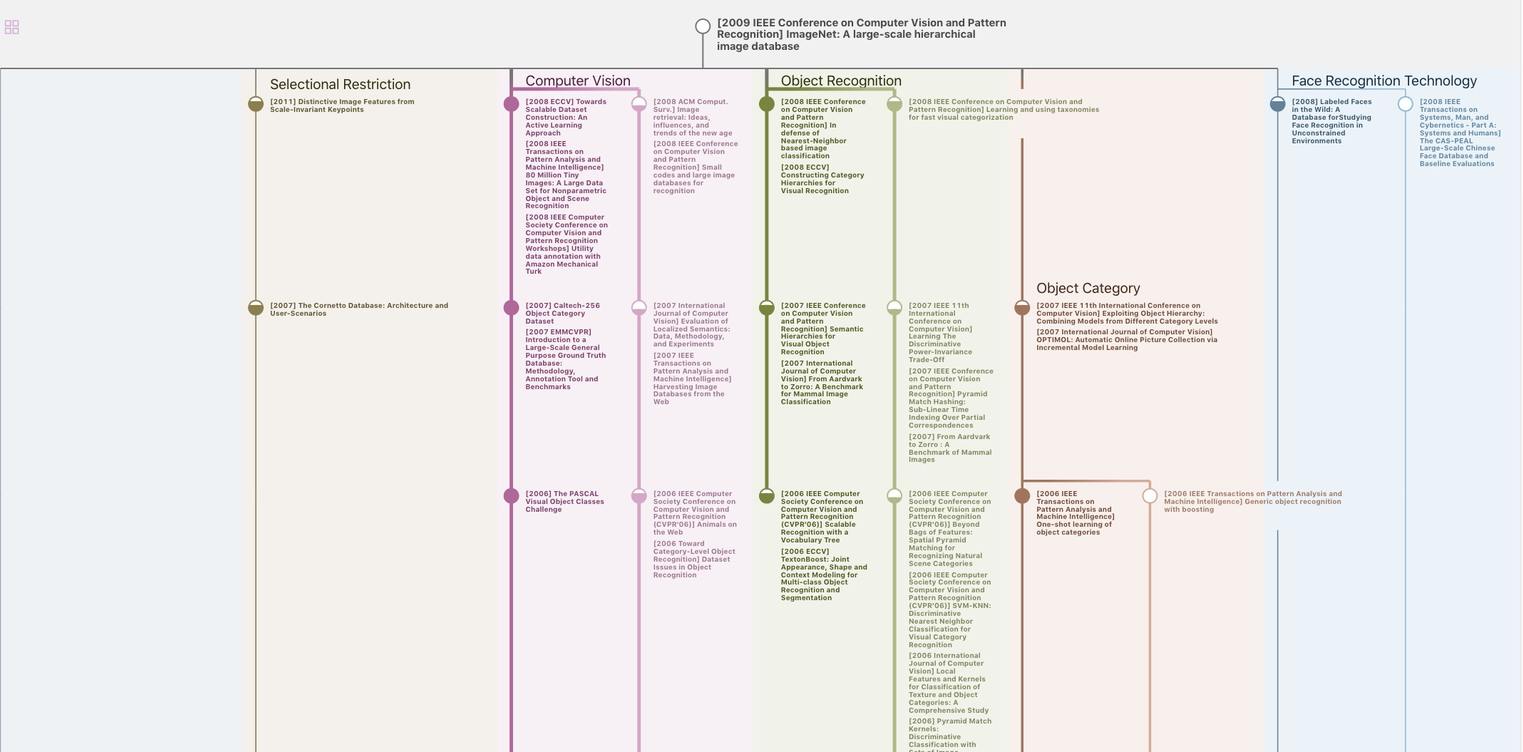
生成溯源树,研究论文发展脉络
Chat Paper
正在生成论文摘要