Improved Topology Extraction Using Discriminative Parameter Mining of Logs
ADVANCES IN KNOWLEDGE DISCOVERY AND DATA MINING, PAKDD 2021, PT I(2021)
摘要
Analytics on log data from various sources like application, middleware and infrastructure plays a very important role in troubleshooting of distributed applications. The existing tools for log analytics work by mining log templates and template sequences. The template sequences are then used to derive the application control flow or topology. In this work, we show how the use of parameters in logs enables discovery of a more accurate application topology, thereby aiding troubleshooting. An accurate application topology information helps in better correlation of logs at runtime, enabling troubleshooting tasks like anomaly detection, fault localization and root cause analysis to be more accurate. To this end, we propose a novel log template mining approach which uses parameter mining combined with fuzzy clustering on historical runtime logs to mine better quality templates. We also leverage parameter flows between log templates using a novel discriminative parameter mining approach for better topology extraction. In our method we do not assume any source code instrumentation or application specific assumptions like presence of transaction identifiers. We demonstrate the effectiveness of our approach in mining templates and application topology using real world as well as simulated data.
更多查看译文
关键词
Observability, Application topology, Parameter mining, Root cause analysis
AI 理解论文
溯源树
样例
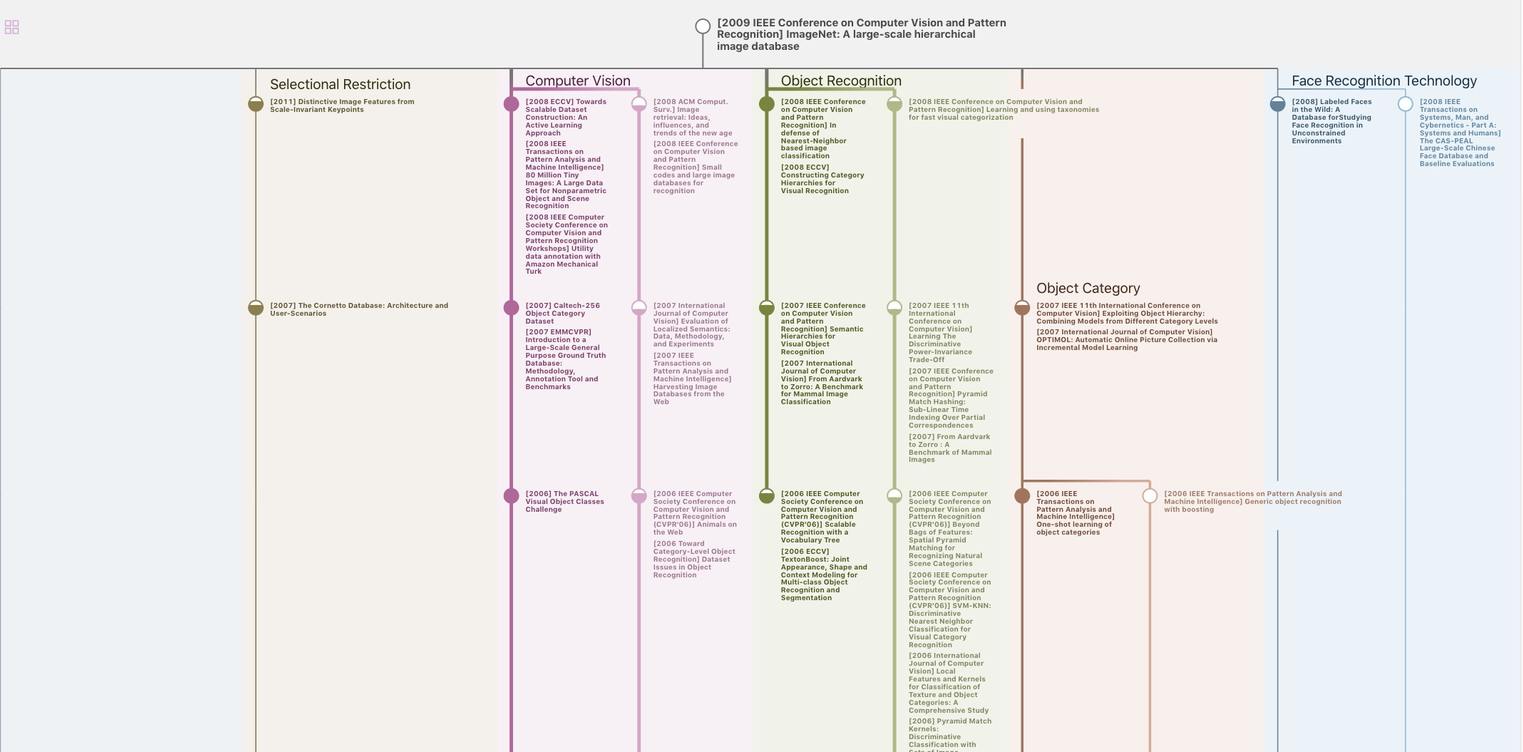
生成溯源树,研究论文发展脉络
Chat Paper
正在生成论文摘要