Mixture Distribution Graph Network for Few Shot Learning
IEEE Transactions on Cognitive and Developmental Systems(2022)
摘要
Few-shot learning aims at heuristically resolving new tasks with limited labeled data; most of the existing approaches are affected by knowledge learned from similar experiences. However, interclass barriers and new samples insufficiency limit the transfer of knowledge. In this article, we propose a novel mixture distribution graph network, in which the interclass relation is explicitly modeled and propagated via graph generation. Owing to the weighted distribution features based on the Gaussian mixture model, we take class diversity into consideration, thereby utilizing information precisely and efficiently. Equipped with minimal gated units, the “memory” of similar tasks can be preserved and reused through episode training, which fills a gap in temporal characteristics and softens the impact of data insufficiency. Extensive trials are carried out based on the MiniImageNet and CIFAR-FS data sets. The results turn out that our method exceeds most state-of-the-art approaches, which shows the validity and universality of our method in few-shot learning.
更多查看译文
关键词
Few-shot learning,graph neural networks,mixture distribution
AI 理解论文
溯源树
样例
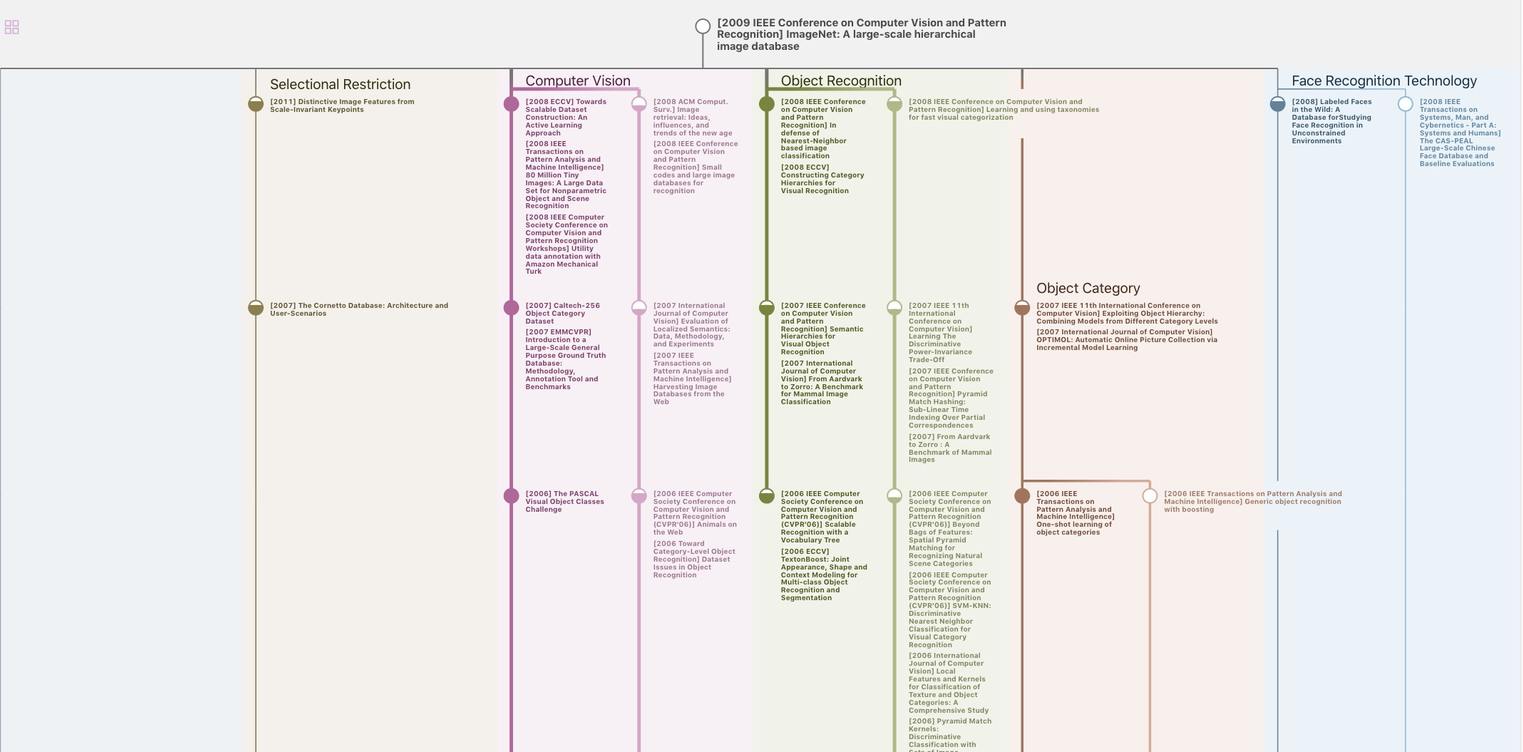
生成溯源树,研究论文发展脉络
Chat Paper
正在生成论文摘要