C 2 -Guard: A Cross-Correlation Gaining Framework for Urban Air Quality Prediction.
pacific-asia conference on knowledge discovery and data mining(2021)
摘要
Predicting air quality is increasingly important for protecting people's daily health and helping government decision-making. The multistep air quality prediction largely depends on the correlations of air quality-related factors. How to model the correlations among factors is a big challenge. In this paper, we propose a cross-correlation gaining framework (C-2-Guard) consisting of a temporal correlation module, factor correlation module, and cross gaining module for air quality (mainly PM2.5) prediction. Specifically, the temporal correlation module is used to extract the temporal dependence of air pollutant time series to gain their distributed representation. In the factor correlation module, a novel convolution and recalibration block is designed for air quality factor correlations extraction to gain their distributed representation in the factor dimension. In the cross gaining module, a joint-representation block is proposed to learn the cross-correlations between time and factor dimensions. Finally, extensive experiments are conducted on two real-world air quality datasets. The results demonstrate that our C-2-Guard outperforms the state-of-the-art methods of air pollutants prediction in terms of RMSE and MAE.
更多查看译文
关键词
Air quality prediction, Temporal correlation, Factor correlation, Cross correlation learning
AI 理解论文
溯源树
样例
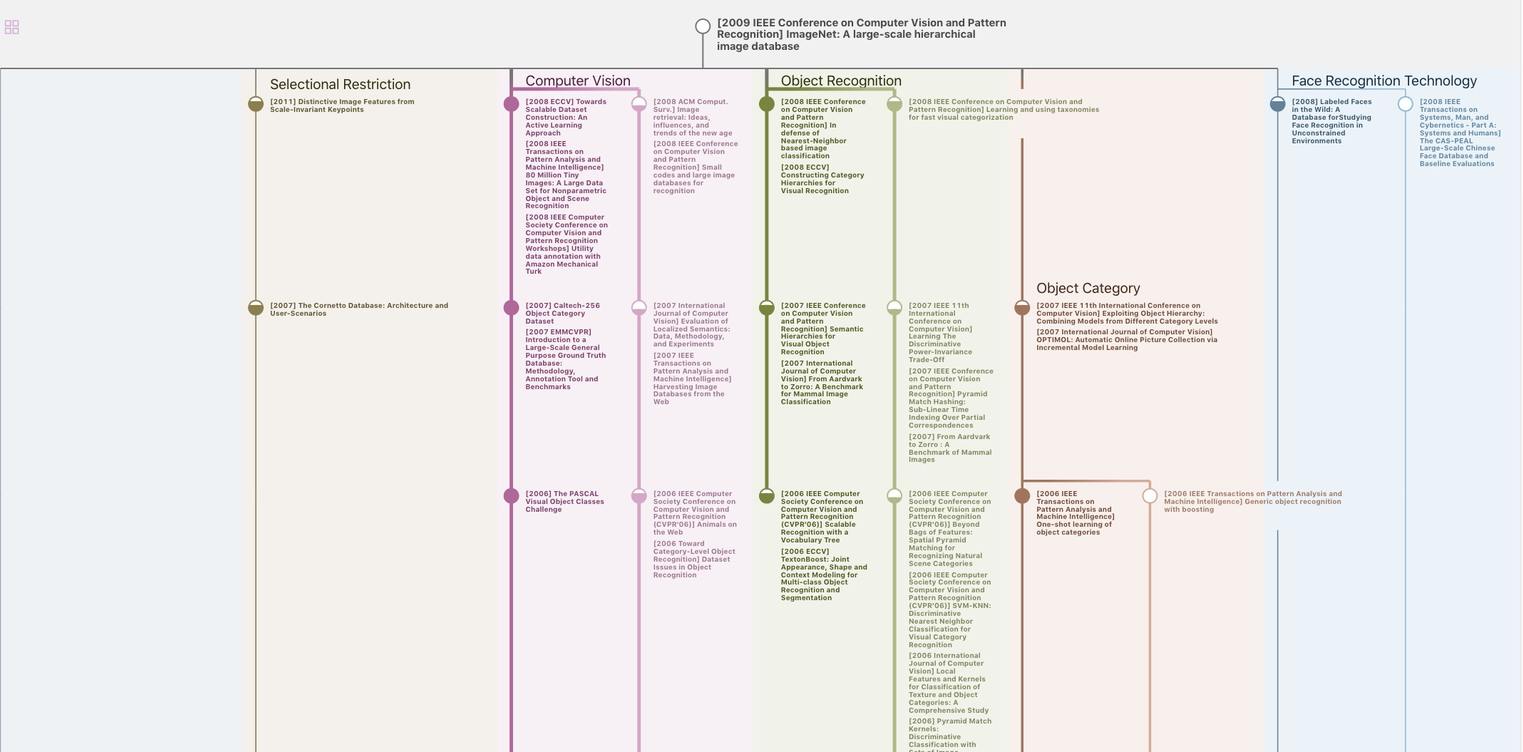
生成溯源树,研究论文发展脉络
Chat Paper
正在生成论文摘要