UNSUPERVISED LEARNING FOR MULTI-STYLE SPEECH SYNTHESIS WITH LIMITED DATA
2021 IEEE INTERNATIONAL CONFERENCE ON ACOUSTICS, SPEECH AND SIGNAL PROCESSING (ICASSP 2021)(2021)
摘要
Existing multi-style speech synthesis methods require either style labels or large amounts of unlabeled training data, making data acquisition difficult. In this paper, we present an unsupervised multi-style speech synthesis method that can be trained with limited data. We leverage instance discriminator to guide a style encoder to learn meaningful style representations from a multi-style dataset. Furthermore, we employ information bottleneck to filter out style-irrelevant information in the representations, which can improve speech quality and style similarity. Our method is able to produce desirable speech using a fairly small dataset, where the baseline GST-Tacotron fails. ABX tests show that our model significantly outperforms GST-Tacotron in both emotional speech synthesis task and multi-speaker speech synthesis task. In addition, we demonstrate that our method is able to learn meaningful style features with only 50 training samples per style.
更多查看译文
关键词
speech synthesis, unsupervised, instance discriminator, information bottleneck
AI 理解论文
溯源树
样例
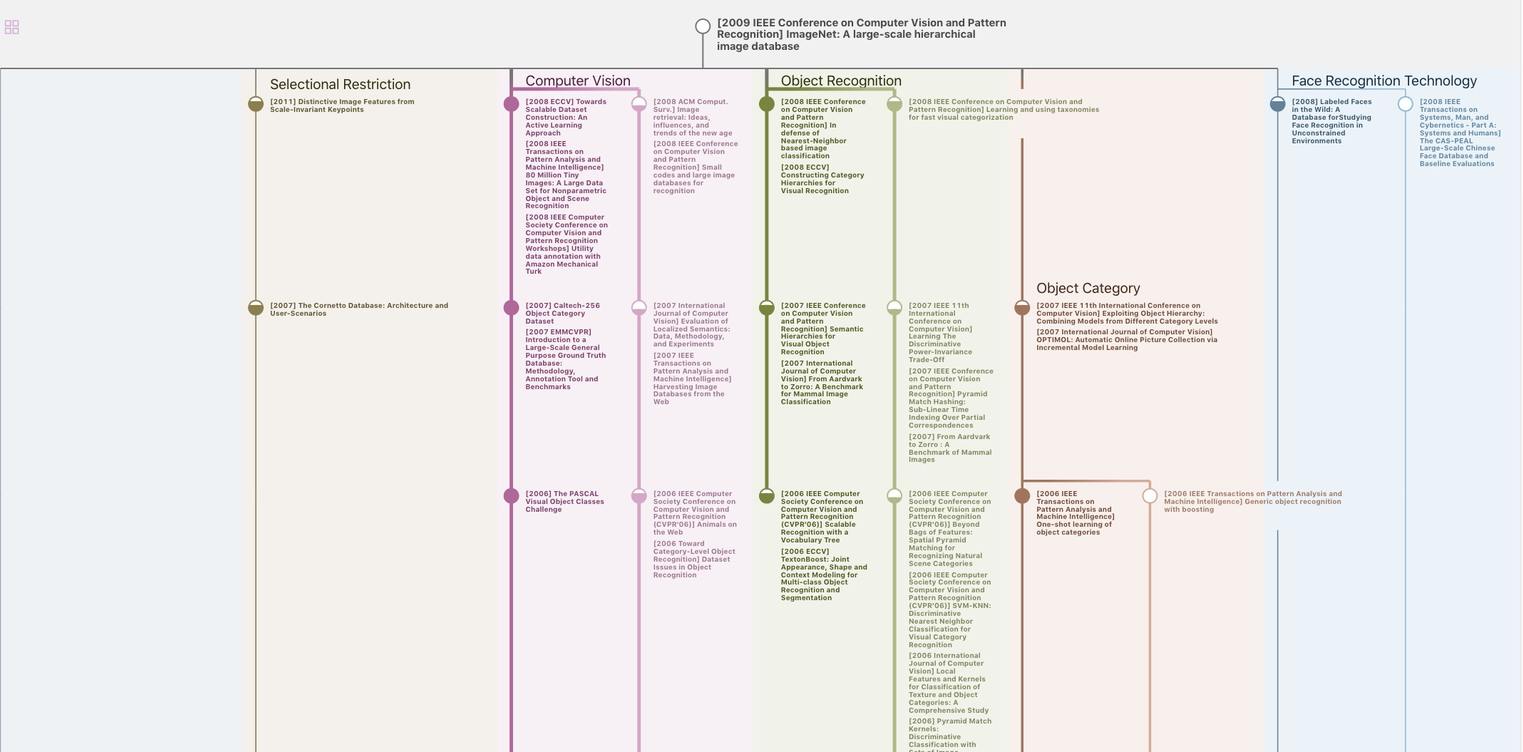
生成溯源树,研究论文发展脉络
Chat Paper
正在生成论文摘要