Data-Driven Many-Objective Crowd Worker Selection for Mobile Crowdsourcing in Industrial IoT
IEEE TRANSACTIONS ON INDUSTRIAL INFORMATICS(2023)
摘要
With the development of mobile networks and intelligent equipment, as a new intelligent data sensing paradigm in large-scale sensor applications such as the industrial Internet of Things, mobile crowd sensing (MCS) assigns industrial sensing tasks to workers for data collection and sharing, which has created a bright future for building a strong industrial system and improving industrial services. How to design an effective worker selection mechanism to maximize the utility of crowdsourcing is the research hotspot of mobile sensing technologies. This article studies the problem of least workers selection to make large MCS system perform sensing tasks more effective and achieve certain coverage with certain constraints being meeting. A many-objective worker selection method is proposed to achieve the desired tradeoff and an optimization mechanism is designed based on the enhanced differential evolution algorithm to ensure data integrity and search solution optimality. The effectiveness of the proposed method is verified through a large scale of experimental evaluation datasets collected from real world.
更多查看译文
关键词
Task analysis,Sensors,Optimization,Quality of service,Informatics,Crowdsourcing,Real-time systems,Enhanced differential evolution,industrial Internet of Things (IIoT),many-objective optimization,mobile crowd sensing (MCS),worker selection
AI 理解论文
溯源树
样例
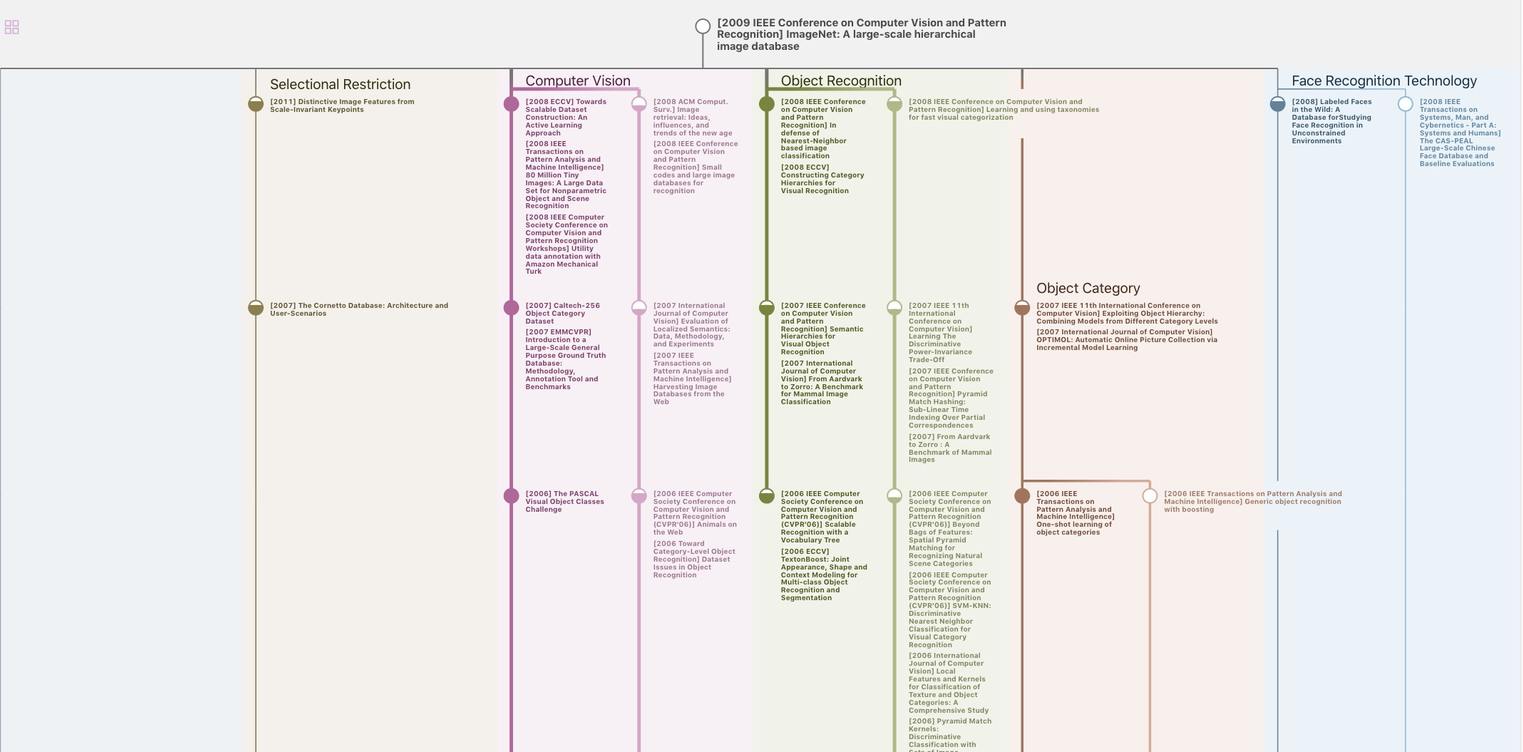
生成溯源树,研究论文发展脉络
Chat Paper
正在生成论文摘要