HCAG: A HIERARCHICAL CONTEXT-AWARE GRAPH ATTENTION MODEL FOR DEPRESSION DETECTION
2021 IEEE INTERNATIONAL CONFERENCE ON ACOUSTICS, SPEECH AND SIGNAL PROCESSING (ICASSP 2021)(2021)
摘要
Depression is one of the most common mental health disorders, it's crucial to design an effective and robust model for automatic depression detection (ADD). Although current approaches rely on extra topic models or manually topic-selection procedures which is time-consuming, they still haven't thoroughly explored the sufficient context information among clinical interviews. In this paper, we propose HCAG, a novel Hierarchical Context-Aware Graph attention model for ADD. Our model mirrors the hierarchical structure of depression assessment and leverages the Graph Attention Network (GAT) to grasp relational contextual information of text/audio modality. Experiments on the DAIC-WOZ dataset show a great performance improvement, with the F1-score of 0.92, a Mean Absolute Error (MAE) of 2.94, and a Root Mean Square Error (RMSE) of 3.80. To the best of our knowledge, our model outperforms the existing state-of-the-art methods.
更多查看译文
关键词
Automatic depression detection, context-aware, Graph attention network
AI 理解论文
溯源树
样例
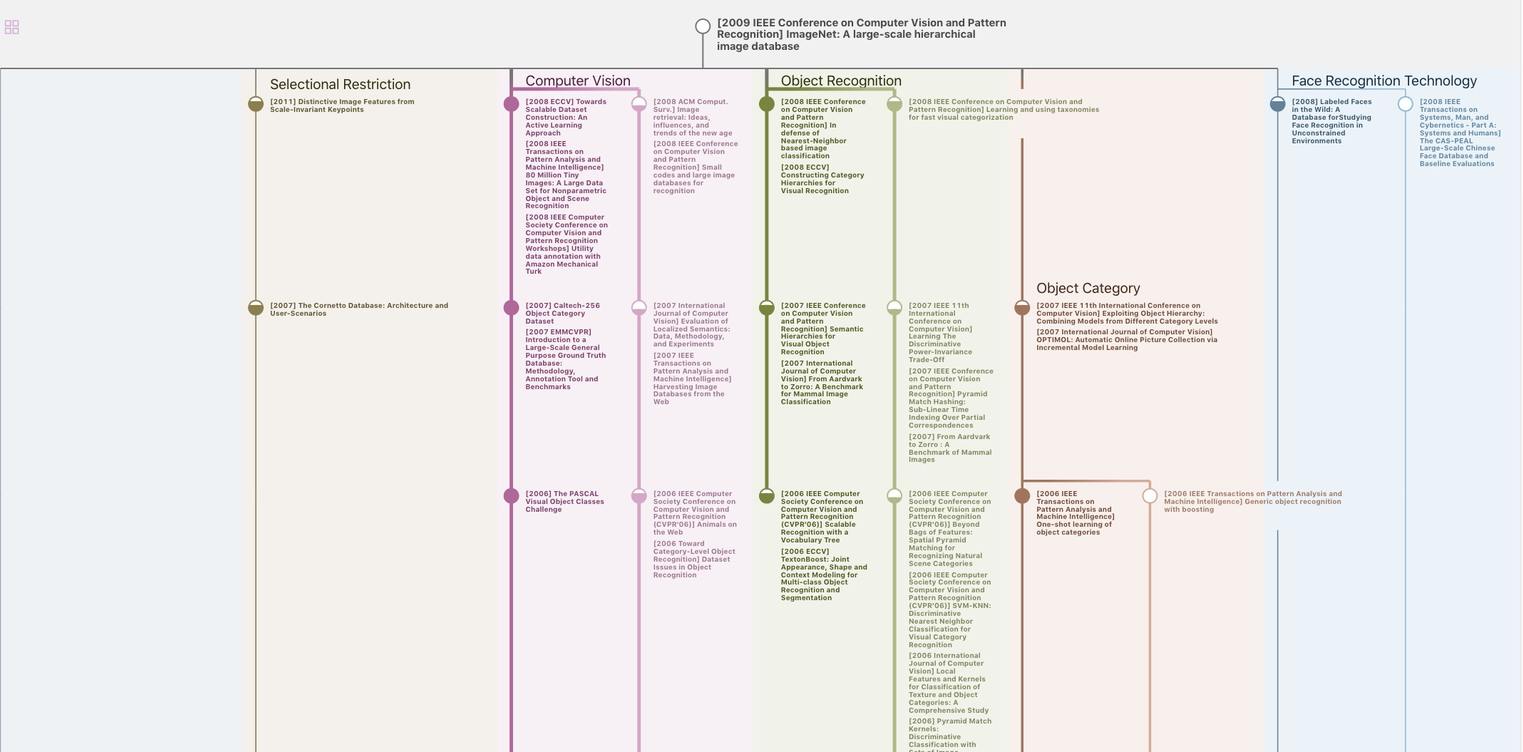
生成溯源树,研究论文发展脉络
Chat Paper
正在生成论文摘要