Transferable Contextual Bandits with Prior Observations
ADVANCES IN KNOWLEDGE DISCOVERY AND DATA MINING, PAKDD 2021, PT II(2021)
摘要
Cross-domain recommendations have long been studied in traditional recommender systems, especially to solve the cold-start problem. Although recent approaches to dynamic personalized recommendation have leveraged the power of contextual bandits to benefit from the exploitation-exploration paradigm, very few works have been conducted on cross-domain recommendation in this setting. We propose a novel approach to solve the cold-start problem under the contextual bandit setting through the cross-domain approach. Our developed algorithm, T-LinUCB, takes advantage of prior recommendation observations from multiple domains to initialize the new arms' parameters so as to circumvent the lack of data arising from the cold-start problem. Our bandits therefore possess knowledge upon starting which yields better recommendation and faster convergence. We provide both a regret analysis and an experimental evaluation. Our approach outperforms the baseline, LinUCB, and experiment results demonstrate the benefits of our model.
更多查看译文
关键词
Contextual bandits, Cross-domain recommendation, Personalized recommendation
AI 理解论文
溯源树
样例
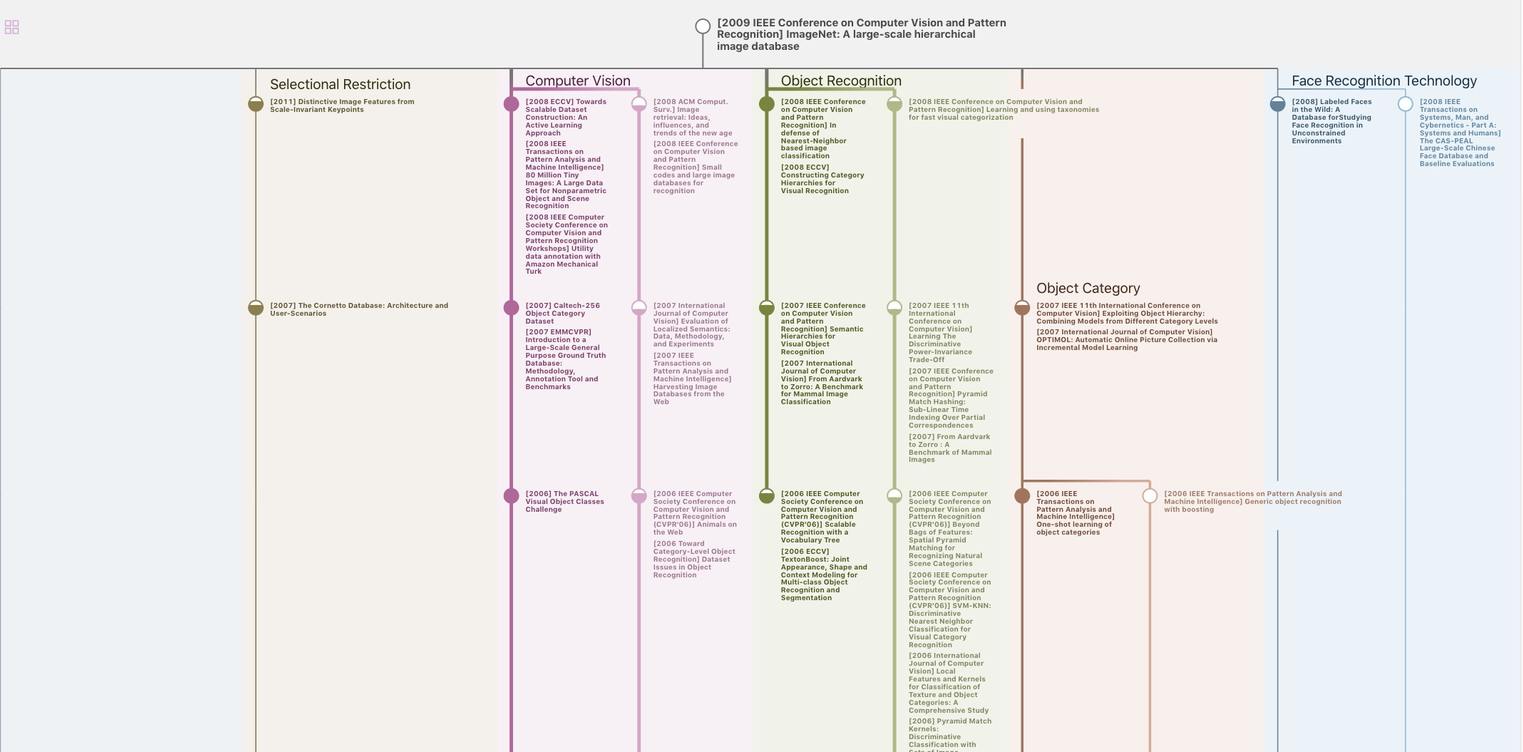
生成溯源树,研究论文发展脉络
Chat Paper
正在生成论文摘要