Evaluation Of The Skill Of Monthly Precipitation Forecasts From Global Prediction Systems Over The Greater Horn Of Africa
WEATHER AND FORECASTING(2021)
摘要
The skill of precipitation forecasts from global prediction systems has a strong regional and seasonal dependence. Quantifying the skill of models for different regions and time scales is important, not only to improve forecast skill, but to enhance the effective uptake of forecast information. The Subseasonal to Seasonal Prediction project (S2S) database contains near-real-time forecasts and reforecasts from 11 operational centers and provides a great opportunity to evaluate and compare the skill of operational S2S systems. This study evaluates the skill of these state-of-the-art global prediction systems in predicting monthly precipitation over the Greater Horn of Africa. This comprehensive evaluation was performed using deterministic and probabilistic forecast verification metrics. Results from the analysis showed that the prediction skill varies with months and region. Generally, the models show high prediction skill during the start of the rainfall season in March and lower prediction skill during the peak of the rainfall in April. ECCC, ECMWF, KMA, NCEP, and UKMO show better prediction skill over the region for most of the months compared with the rest of the models. Conversely, BoM, CMA, HMCR, and ISAC show poor prediction skill over the region. Overall, the ECMWF model performs best over the region among the 11 models analyzed. Importantly, this study serves as a baseline skill assessment with the findings helping to inform how a subset of models could be selected to construct an objectively consolidated multimodel ensemble of S2S forecast products for the Greater Horn of Africa region, as recommended by the World Meteorological Organization.
更多查看译文
关键词
Africa, Forecast verification, skill, Forecasting, Hindcasts, Model comparison, Model evaluation, performance
AI 理解论文
溯源树
样例
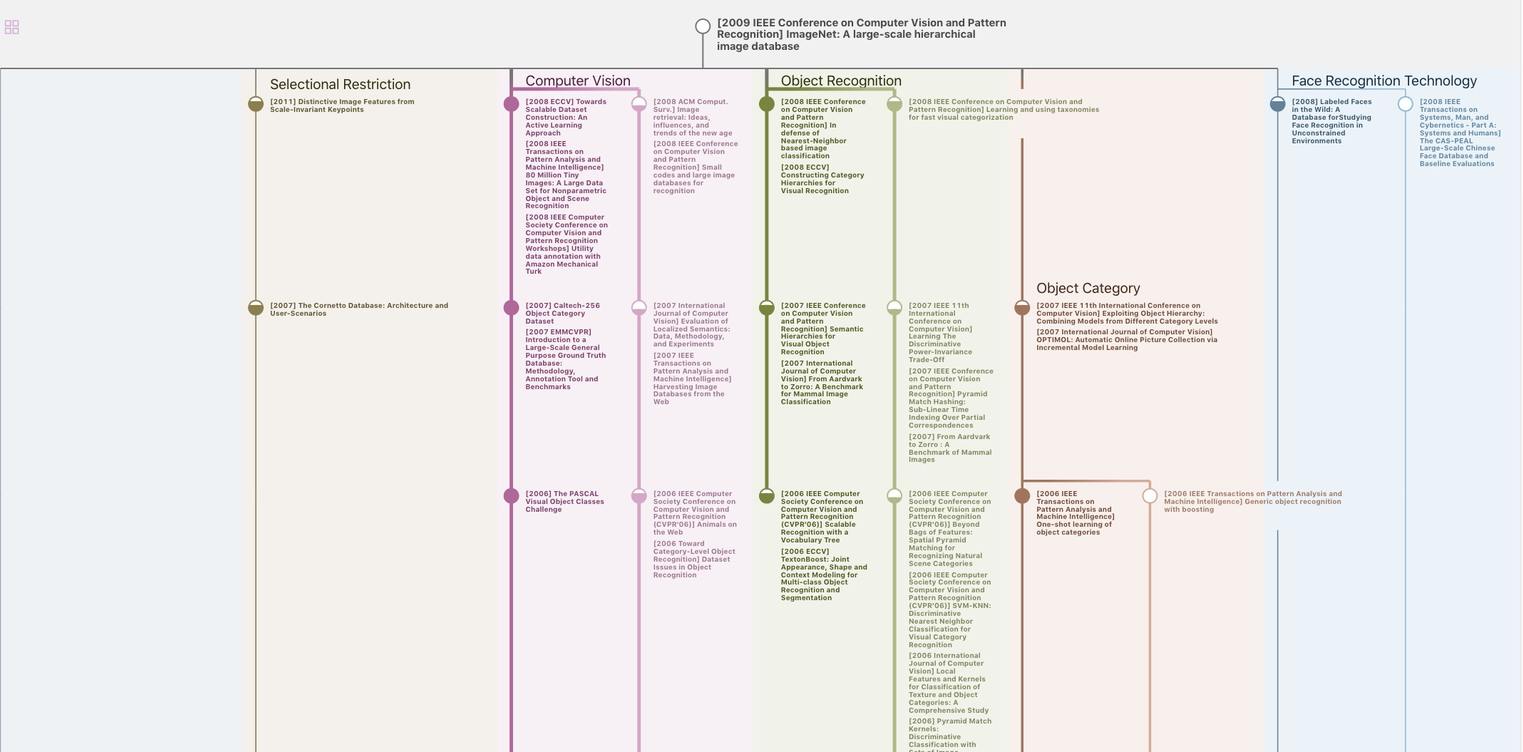
生成溯源树,研究论文发展脉络
Chat Paper
正在生成论文摘要