An Online Learning Algorithm for Non-stationary Imbalanced Data by Extra-Charging Minority Class
ADVANCES IN KNOWLEDGE DISCOVERY AND DATA MINING, PAKDD 2021, PT I(2021)
摘要
Online learning is one of the trending areas of machine learning in recent years. How to update the model based on new data is the core question in developing an online classifier. When new data arrives, the classifier should keep its model up-to-date by (1) learn new knowledge, (2) keep relevant learned knowledge, and (3) forget obsolete knowledge. This problem becomes more challenging in imbalanced non-stationary scenarios. Previous approaches save arriving instances, then utilize up/down sampling techniques to balance preserved samples and update their models. However, this strategy comes with two drawbacks: first, a delay in updating the models, and second, the up/down sampling causes information loss for the majority classes and introduces noise for the minority classes. To address these drawbacks, we propose the Hyper-Ellipses-Extra-Margin model (HEEM), which properly addresses the class imbalance challenge in online learning by reacting to every new instance as it arrives. HEEM keeps an ensemble of hyper-extended-ellipses for the minority class. Misclassified instances of the majority class are then used to shrink the ellipse, and correctly predicted instances of the minority class are used to enlarge the ellipse. Experimental results show that HEEM mitigates the class imbalance problem and outperforms the state-of-the-art methods.
更多查看译文
关键词
Online learning, Imbalanced data, Nonstationary data
AI 理解论文
溯源树
样例
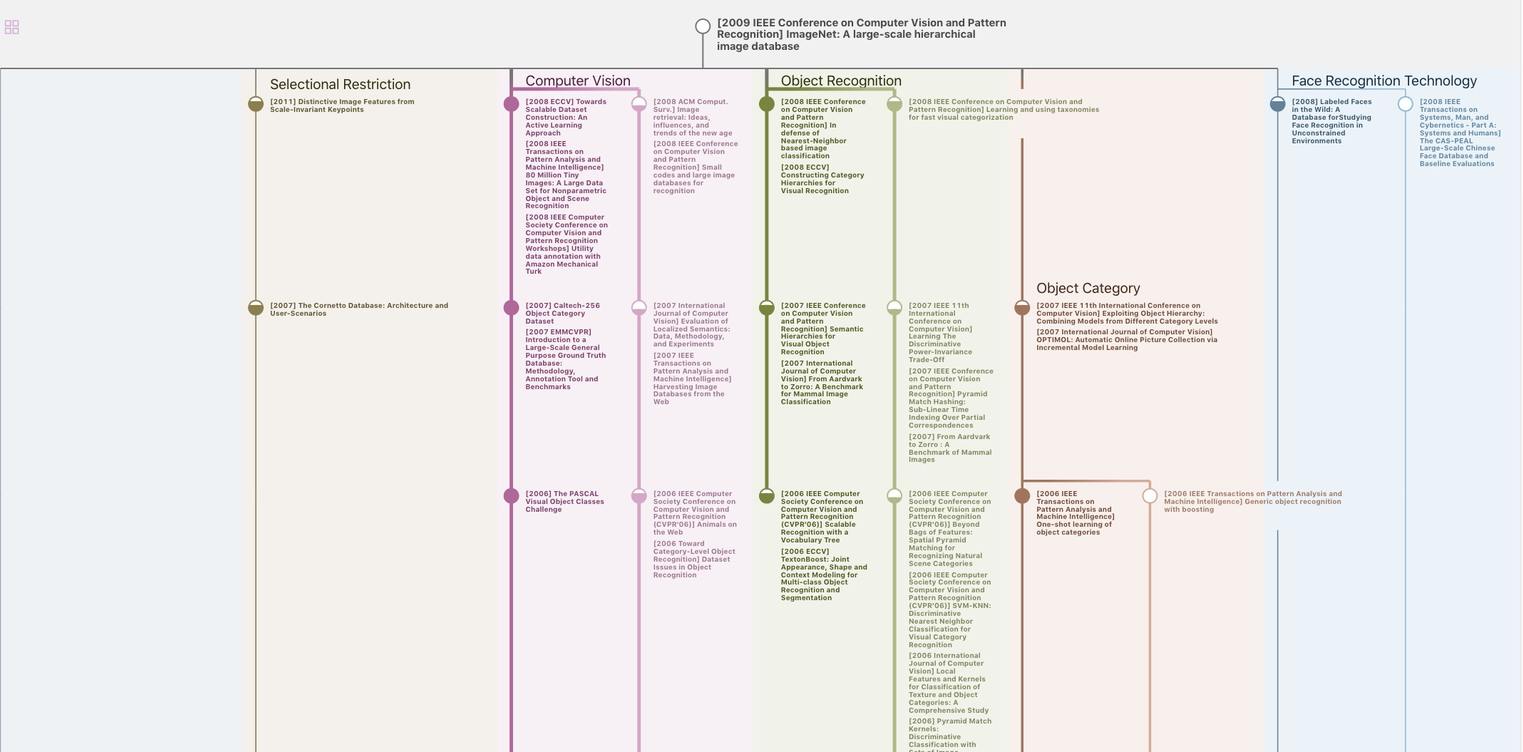
生成溯源树,研究论文发展脉络
Chat Paper
正在生成论文摘要